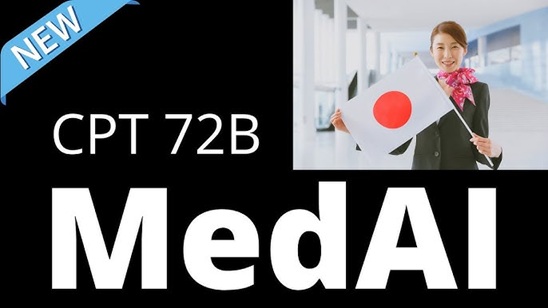
Introduction
This article explores a recent breakthrough in Japanese medical AI: a specialized large language model (LLM) developed for the Japanese medical field. The researchers created a model that achieves stable reasoning patterns—essential for medical applications—by combining continuous pre-training with specialized policy optimization. The article also examines how the growing demand for specialized AI expertise, particularly in quantum computing, affects Traditional Chinese Medicine (TCM).
Quantum AI: The Emerging Frontier
What is Quantum AI?
Quantum AI combines quantum computing with artificial intelligence, leveraging quantum mechanical phenomena like superposition and entanglement to potentially solve complex problems beyond classical computing capabilities.
Key Principles:
- Quantum Bits (Qubits): Unlike classical bits (0 or 1), qubits can exist in superposition, representing multiple states simultaneously
- Quantum Entanglement: Allows qubits to be correlated in ways that have no classical equivalent
- Quantum Parallelism: Enables exploring multiple solutions simultaneously
Potential Applications in AI:
- Accelerating machine learning training on massive datasets
- Solving optimization problems more efficiently
- Enhancing pattern recognition in complex data
- Developing more sophisticated neural networks
Current Limitations:
- Quantum decoherence and error rates
- Limited qubit stability and scalability
- Need for specialized knowledge combining quantum physics, computer science, and AI
Industry Development:
- Major players include IBM, Google, Microsoft, and numerous startups
- Projected to create approximately 250,000 jobs by 2030 and nearly 1 million by 2035
- Significant talent shortage as the field grows faster than education programs can produce experts
Reasoning in Medical LLMs
The Importance of Stable Reasoning:
- Medical AI requires consistent, reliable reasoning paths to support critical healthcare decisions
- Unstable reasoning (varying explanations for the same questions) is unacceptable in clinical settings
- Reasoning transparency allows healthcare professionals to verify AI conclusions
Recent Advances – Japanese Medical LLM Case Study:
- Two-stage approach combining Continuous Pre-Training (CPT) and Reasoning Preference Optimization (RPO)
- CPT instills domain-specific medical knowledge
- RPO ensures consistent reasoning patterns and explanations
Training Methodologies:
- Continuous Pre-Training: Adds specialized medical knowledge to base models
- Most significant performance improvement (80% → 86.7% in the Japanese case study)
- Efficient for domain knowledge transfer
- Reasoning Preference Optimization: Specialized version of Direct Preference Optimization (DPO)
- Creates training data by categorizing responses as correct/incorrect based on final answers
- Optimizes for consistency in reasoning paths, not just correct answers
- Helps maintain stable explanations even at different temperature settings
Evaluation Approaches:
- Multiple-choice question benchmarks (e.g., medical licensing exams)
- Step-by-step explanation requirements to assess reasoning quality
- Comparison of performance with and without explanation requirements
Implementation Challenges:
- Computational resource limitations
- Balancing model size with practicality (e.g., using techniques like Q-LoRA and quantization)
- Creating high-quality training data for reasoning optimization
Convergence of Quantum AI and Medical Reasoning
While still largely theoretical, the convergence of quantum computing and medical LLMs could potentially:
- Process Complex Medical Data: Quantum algorithms may efficiently analyze multidimensional medical datasets
- Optimize Treatment Plans: Find optimal treatment combinations from vast possibility spaces
- Accelerate Medical Research: Speed up drug discovery and biomedical simulations
- Enhance Reasoning Capabilities: Develop more robust reasoning systems that consider multiple diagnostic paths simultaneously
The intersection of these fields represents one of the most promising and challenging frontiers in AI research, requiring interdisciplinary expertise across medicine, AI, and quantum physics.
Video about Reasoning in Medical LLM
Related Section of Video
The core of the video focuses on a research paper published on April 25th titled “Stabilizing Reasoning in Medical LLMs” from the University of Tokyo in collaboration with a Japanese company. The researchers developed a Japanese-specific medical LLM using a two-stage fine-tuning process:
- Continuous Pre-Training (CPT): The researchers started with a base Q1 72B model and enhanced it with Japanese medical domain knowledge. This step significantly improved performance from 80% to 86.7% on their benchmark.
- Reasoning Preference Optimization (RPO): This modified form of Direct Preference Optimization (DPO) was implemented to ensure consistent and coherent reasoning traces, which is crucial for medical applications. Adding RPO provided a marginal improvement from 86.7% to 86.8%.
For their training infrastructure, they used four NVIDIA A100 80GB GPUs with Q-LoRA adaptation and 4-bit quantization to manage computational limitations. The model was evaluated using Japanese medical licensing examination benchmarks in both three-shot settings (with and without explanation requirements).
The study demonstrates that CPT alone yielded the most substantial performance improvement, indicating that continuous pre-training is likely the most resource-efficient method for acquiring general medical knowledge.
Impact of Quantum AI and Advanced Reasoning on Traditional Chinese Medicine (TCM)
Traditional Chinese Medicine (TCM), with its holistic approach and complex diagnostic systems, presents both unique challenges and opportunities for integration with Quantum AI and advanced reasoning systems. Here’s how these technologies could impact TCM:
Diagnostic Enhancement and Pattern Recognition
Pulse Diagnosis Digitization:
- Quantum computing could help analyze the subtle variations in TCM pulse diagnosis (which identifies dozens of pulse qualities)
- Advanced sensors paired with quantum pattern recognition could capture and interpret pulse patterns that currently rely on practitioner experience
Facial and Tongue Analysis:
- Quantum-enhanced image processing could recognize subtle patterns in tongue and facial diagnostics
- LLMs with stable reasoning pathways could explain connections between observed patterns and underlying imbalances
Five Elements and Zang-Fu Theory Integration:
- Quantum computing’s ability to model complex interrelated systems could help digitize the relationships between the Five Elements and organ systems
- Create comprehensive models of how TCM understands the body’s interconnected nature
Herbal Medicine Optimization
Formula Customization:
- Quantum algorithms could navigate the vast combinatorial space of herbal formulas (sometimes combining 10-20 herbs)
- Optimize personalized formulas by considering patient constitution, symptoms, and herb interactions simultaneously
Active Compound Analysis:
- Map complex interactions between multiple compounds in TCM herbs that create synergistic effects
- Identify previously unknown mechanisms of action in traditional formulations
Quality Assessment:
- Develop advanced models for evaluating herb quality based on growing conditions, harvesting time, and processing methods
- Create standardization frameworks that respect TCM principles while ensuring consistency
Integration with Western Medicine
Bridging Medical Paradigms:
- Medical LLMs with stable reasoning could act as translators between TCM concepts and Western medical terminology
- Help practitioners from both traditions understand patient conditions through multiple frameworks
Research Validation:
- Quantum computing could help design complex clinical trials that respect TCM’s holistic approach
- Advanced modeling of multi-target therapeutic effects that align with TCM’s systems-based approach
Personalized Integrative Medicine:
- Create treatment plans that optimally combine TCM and Western approaches
- Model how acupuncture, herbs, pharmaceuticals, and lifestyle changes interact as a system
Preservation and Evolution of Traditional Knowledge
Knowledge Formalization:
- Capture tacit knowledge from master practitioners through advanced reasoning systems
- Create comprehensive knowledge bases that preserve centuries of clinical observations
Theory Modernization:
- Quantum models might help reinterpret classical TCM concepts like Qi and meridians in ways that bridge traditional understanding with contemporary science
- Evolve TCM theory while maintaining its core philosophical foundations
Practical Implementation Considerations
Cultural Sensitivity:
- AI systems would need to be trained on culturally appropriate data that respects TCM’s philosophical foundations
- Reasoning pathways must incorporate TCM’s holistic diagnostic approach rather than imposing reductionist frameworks
Practitioner Augmentation:
- Technology designed to support rather than replace the skilled TCM practitioner
- Preserve the essential human elements of TCM practice while enhancing diagnostic precision
Regulatory Frameworks:
- Development of appropriate validation systems that acknowledge TCM’s different epistemological approach
- Standards for AI applications in TCM that ensure safety while respecting traditional methodologies
Conclusion
This development exemplifies an important emerging trend in artificial intelligence: the creation and deployment of region-specific AI models that are deeply attuned to local languages, cultural contexts, and specialized knowledge domains. These models demonstrate respect for and understanding of the nuanced ways different cultures approach knowledge and practice. The Japanese medical LLM represents a particularly noteworthy advancement in the development of specialized AI systems, showcasing how targeted models can deliver consistent, reliable reasoning capabilities essential for critical fields like healthcare. The success of this implementation suggests a promising direction for future AI development that prioritizes cultural specificity alongside technical excellence. Furthermore, the convergence of Quantum AI capabilities and advanced reasoning systems with Traditional Chinese Medicine presents an exciting opportunity for revolutionary progress in traditional medical practices. This integration has the potential to catalyze a renaissance in traditional medicine, offering a pathway to preserve and enhance centuries-old wisdom while simultaneously improving its precision, reliability, and accessibility within modern healthcare frameworks. This careful balance between honoring traditional knowledge and leveraging cutting-edge technology could serve as a model for similar integrations across other specialized domains.
Key Takeaways:
- Localized AI Models Matter: Creating region-specific models in native languages that respect local cultural contexts is valuable, as demonstrated by this Japanese medical AI system.
- Continuous Pre-Training (CPT) Is Highly Effective: For domain specialization, adding knowledge through CPT provided the most significant performance improvement (80% to 86.7%), suggesting this is an efficient approach for knowledge transfer.
- Stable Reasoning Is Critical for Medical AI: The researchers prioritized consistent reasoning patterns through RPO, acknowledging that inconsistent explanations are unacceptable in clinical settings.
- Computational Resource Limitations Affect Research: The presenter highlighted how limited computational resources (using only four A100 GPUs and 4-bit quantization) potentially constrained the research outcomes.
- Talent Shortage in Advanced AI Fields: The video concludes by discussing the growing demand for specialized AI talent, particularly in quantum computing, with projections of 250,000 new jobs by 2030 and nearly 1 million by 2035.
- Bridging Ancient Wisdom with Modern Technology: TCM’s holistic patterns align with quantum computing’s ability to model complex interconnected systems. Quantum algorithms can map the multidimensional relationships in Five Elements theory and herb interactions
- Personalized Medicine Revolution: Quantum computing can optimize individual herbal formulas from thousands of possible combinations. Reasoning LLMs can explain treatment rationales in culturally appropriate frameworks. Patient-specific models can integrate pulse, tongue, and constitution diagnostics with biomedical data.