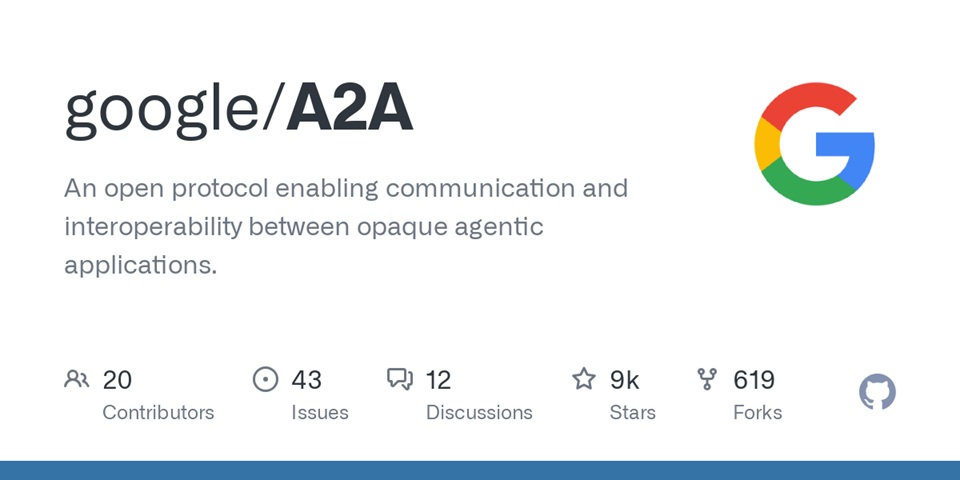
Introduction
This review examines a recent Google’s Agent-to-Agent (A2A) protocol alongside the Model Context Protocol (MCP) within the framework of multi-agent AI systems. It provides a comprehensive overview of how these protocols work together in Google’s new Agent Development Kit (ADK), explaining their distinct purposes, compatibility features, and practical applications across different AI ecosystems.
Video about the Google A2A with MCP within the framework
Understanding the Protocol Architecture :
Model Context Protocol (MCP) Explained
The video begins by explaining the Model Context Protocol (MCP), which uses a client-server architecture to connect AI models with external data and tools. This protocol enables agents to access services like Google Search or weather data through structured formats (typically JSON), effectively transforming these services into accessible external data tools.
Agent Development Kit (ADK) Overview
Google’s Agent Development Kit (ADK) is highlighted as a solution that simplifies agent development, particularly when using Google’s ecosystem. A key advantage of ADK is its compatibility with existing MCP client-server architectures, allowing organizations with legacy systems to integrate seamlessly with the new framework.
Agent-to-Agent (A2A) Protocol
The A2A protocol represents a higher-level communication system designed specifically for complex interactions between intelligent agents. Unlike MCP, which focuses on data exchange, A2A enables:
• Complex multi-turn dialogues between agents
• Context maintenance throughout conversations
• Dynamic behavior adjustments based on conversation flow
• Autonomous decision-making and problem-solving
Distinguishing Between Tools and Agents
The video emphasizes an important conceptual distinction:
• Tools: Narrow in scope with clear inputs/outputs and no intelligence required
• Agents: Feature context awareness, memory capabilities, autonomy in decision-making, and can engage in multi-turn conversations
A single AI system can incorporate multiple protocol layers:
• Function as an MCP client (requesting services)
• Operate as an MCP host (exposing internal tools)
• Utilize A2A protocol for communication with other intelligent agents
Cross-Ecosystem Compatibility
One of the most significant advantages highlighted is the cross-ecosystem interoperability of the A2A protocol. With over 50 partner companies already integrated (including Accenture, Salesforce, SAP, MongoDB, and Neo4J), the protocol enables communications across different AI frameworks and vendor solutions.
Technical Implementation Details
The A2A protocol is built upon:
• Standard HTTP for transport
• JSON RPC 2.0 for message exchange
• Server-Sent Events (SSE) for real-time streaming
A key component is the “agent card” – a JSON file attached to each agent that:
• Is visible to all other agents
• Hosts a well-known URL advertising the agent’s capabilities
• Provides connection details
• Enables dynamic discovery by other agents
Practical Example: Research Assistant Scenario
The video provides a practical example of a virtual research assistant that:
- Uses MCP as a client to query scientific publication databases
- Functions as an MCP host to provide analysis
- Employs A2A to communicate with lab equipment agents to conduct experiments
This demonstrates how multiple protocols can work together in a complex AI ecosystem, allowing for rich interactions between different agent types while maintaining backward compatibility with existing systems.
Conclusion
The video explains how Google has designed an agent-to-agent protocol that works across different ecosystems while maintaining compatibility with legacy MCP systems. This approach represents a significant advancement in multi-agent AI systems by enabling:
Key Takeaways
• Seamless integration between diverse A2A agents and MCP-powered tools
• Cross-ecosystem compatibility that transcends vendor-specific limitations
• Dynamic agent and tool discovery mechanisms
• Enterprise-level security with authentication and authorization features
• Support for both short-term interactions and long-running tasks
• Open-source approach encouraging broader adoption and innovation
Related References
- Google’s Agent Development Kit (ADK) documentation
- A2A protocol specifications
- Model Context Protocol (MCP) framework documentation
- Various agent frameworks mentioned: