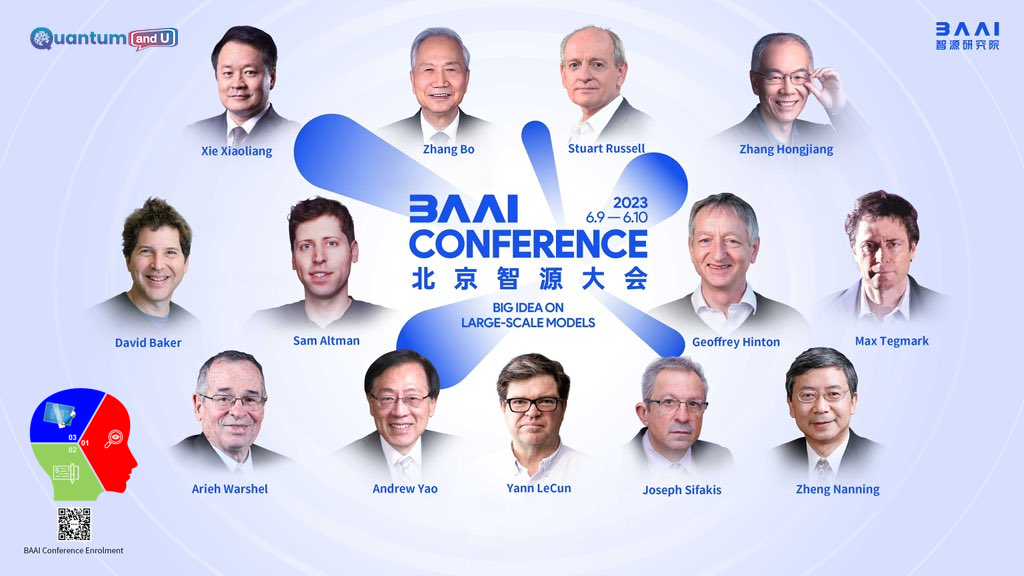
In this blog review, we will delve into a presentation titled “Scaling Large Language Models: From Power Law to Sparsity” by Zhou Yanqi at the 2023 Beijing Zhiguan Conference. The talk discusses the evolution of deep learning models and the challenges posed by the limitations of scaling Moore’s Law. The speaker shares insights from research work at Google Brain, focusing on topics such as T5 unifying the text transformer, Moe architecture, advanced Moe techniques, and sparsity in large language models.
Related Sections:
- Moore’s Law and Power Law in Deep Learning:
The talk begins by highlighting the influence of hardware development on the thriving growth of deep learning models. The speaker explains Moore’s Law, which postulates that the number of transistors on a chip will double every one to two years, driving the development of large deep learning models. However, it is noted that the scaling of Moore’s Law is reaching its limits, posing challenges for further model scaling. - Research Work on Large Language Models:
The speaker discusses research conducted at Google Brain, including the T5 model and recent advancements in Moe architecture. The T5 model, along with the C4 dataset, has significantly contributed to the research community, serving as a basis for numerous follow-up papers. Transfer learning and contextual few-shot learning are explained, shedding light on the differences between these approaches. - Efficient Scaling with Sparsity:
The presentation explores the concept of sparsity in large language models and introduces sparsely gated models with a smaller number of activated parameters compared to previous models like GPT-3. The advantages of sparsity, such as reduced training energy and improved training convergence, are discussed. The speaker explains the use of expert choice routing to address loading imbalance issues and proposes the “Brainformer” architecture, which demonstrates faster training and step times compared to previous models. - Progressive Lifelong Learning:
The talk introduces progressive lifelong learning on a mixture of experts, a method to incrementally learn new training data while retaining previous knowledge. The speaker highlights the benefits of this approach, such as improved performance on downstream tasks and better retention of old data. The use of a lifelong learning Moe is compared to multitask learning, showcasing its advantages even with limited access to specific data sets.
Conclusion:
In conclusion, the presentation provides valuable insights into the scaling of large language models, tackling the challenges posed by the limitations of Moore’s Law. The speaker discusses various research works, including advancements in Moe architecture and the benefits of sparsity. Additionally, the concept of progressive lifelong learning on a mixture of experts is introduced as a method to efficiently adapt language models to new training data while retaining previous knowledge.
Key Takeaway Points:
- Deep learning models have thrived due to hardware development, but the scaling of Moore’s Law is reaching its limits.
- Research work at Google Brain, including the T5 model and the C4 dataset, has significantly contributed to the field of large language models.
- Sparsity in large language models offers advantages such as reduced training energy and improved training convergence.
- Expert choice routing helps address loading imbalance issues, leading to faster training and improved efficiency.
- Progressive lifelong learning on a mixture of experts allows for incremental learning while retaining previous knowledge, leading to improved downstream task performance.
Overall, the talk provides valuable insights into the challenges and advancements in scaling large language models, offering a comprehensive overview of the topic.