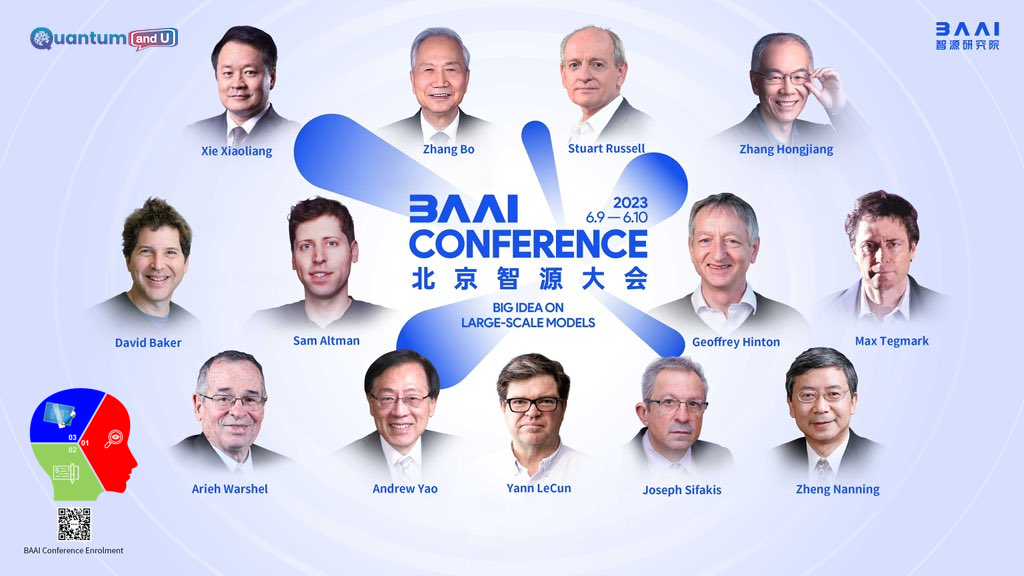
Introduction:
In a recent speech at the BAAI Conference, renowned computer scientist Yann LeCun discussed the challenges of creating artificial intelligence (AI) systems that possess the ability to learn, reason, and plan. LeCun, well-known for his work in deep learning and convolutional neural networks (CNNs), highlighted the limitations of current AI systems and proposed new approaches to address these shortcomings.
Related Sections:
- The Limitations of Current AI Systems:
LeCun pointed out that while AI systems have made significant progress, they still fall short in areas such as learning, reasoning, and planning. He emphasized the drawbacks of supervised learning, reinforcement learning, and self-supervised learning, highlighting their specialized and brittle nature, lack of reasoning abilities, and inability to handle factual information. - Introducing Self-Supervised Learning:
LeCun introduced the concept of self-supervised learning as an alternative approach to training AI systems. Unlike traditional supervised learning, self-supervised learning involves predicting the missing parts of input data, focusing specifically on predicting the last word or token. By training on vast amounts of data, the system can learn to predict future tokens, enabling it to generate coherent sequences of information. - World Models and Hierarchical Planning:
LeCun discussed the idea of world models and hierarchical planning as a framework for reasoning and planning in AI systems. He explained how perception systems analyze the state of the world and combine it with previous knowledge to predict future states based on actions taken. By minimizing a cost function, the system aims to generate a sequence of actions that achieve the desired outcome. - Joint Embedding Predictive Architectures:
LeCun presented joint embedding predictive architectures as a way to train AI systems to predict and integrate information from different modalities. By using regularized methods and minimizing the prediction error, these architectures can learn robust and informative representations, which can be utilized for tasks such as image prediction and planning.
Conclusion:
Yann LeCun’s speech highlighted the need for AI systems that can learn, reason, and plan effectively. He proposed self-supervised learning, world models, and hierarchical planning as key components in achieving these goals. By designing appropriate cost functions and ensuring safety measures, AI systems can become more controllable and reliable. While challenges remain, LeCun’s insights pave the way for further advancements in AI research and development.
Takeaway Key Points:
- Current AI systems lack the ability to learn, reason, and plan effectively.
- Self-supervised learning offers an alternative approach to training AI systems by predicting missing parts of input data.
- World models and hierarchical planning provide a framework for reasoning and generating sequences of actions.
- Joint embedding predictive architectures enable the integration of information from different modalities for prediction and planning tasks.
- Designing robust cost functions and ensuring safety measures are crucial for developing controllable and reliable AI systems.