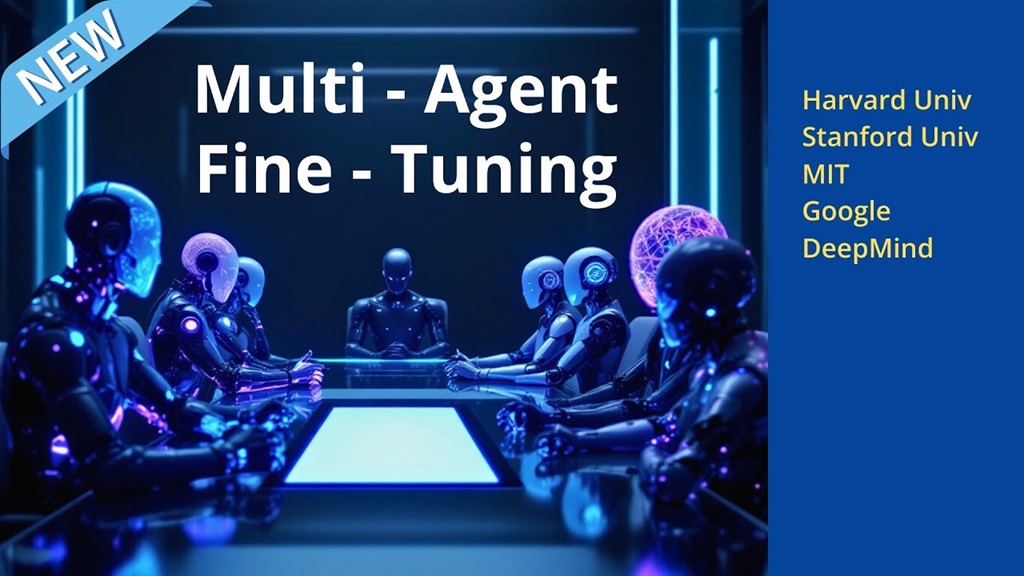
Introduction:
A groundbreaking collaborative research paper, jointly authored by leading researchers from MIT, Harvard University, Stanford University, and Google DeepMind, delves into the innovative field of multi-agent fine-tuning for language models. This cutting-edge research addresses a significant and persistent challenge in the field: the phenomenon where language models exhibit diminishing returns and decreased performance improvements after undergoing multiple iterations of fine-tuning. This limitation has long been a bottleneck in advancing the capabilities of language models, making this research particularly significant for the future of artificial intelligence development.
Enhancing Language Model Performance and Diversity Through Multiagent Fine-Tuning
A team of researchers from MIT, Harvard University, Stanford University, and Google DeepMind:
The collaborative research paper explores a novel approach to fine-tuning large language models (LLMs) by leveraging multi-agent interactions. The proposed method, dubbed “Multiagent Fine-Tuning,” involves training a society of LLMs to collaboratively solve tasks. This approach departs from traditional single-agent fine-tuning, where a single model is trained on a fixed dataset.
Key Contributions:
- Multiagent Debate: The paper introduces a multiagent debate framework where multiple LLMs engage in iterative discussions to generate high-quality training data. https://openreview.net/forum?id=ChNy95ovpF#:~:text=Metareview%3A,to finetune GPT-3.5 models.
- Specialized Agents: By fine-tuning LLMs on data generated through multiagent debate, the researchers demonstrate the creation of specialized agents with distinct strengths. https://arxiv.org/abs/2501.05707
- Enhanced Performance and Diversity: Empirical results show that multiagent fine-tuning leads to significant improvements in reasoning tasks and zero-shot generalization, while also promoting diversity in reasoning chains. https://arxiv.org/abs/2501.05707#:~:text=As a result%2C our overall,fine-tuning than single-agent
Methodology: The multiagent fine-tuning process consists of two key steps:
- Multiagent Debate: A group of LLMs, either identical or fine-tuned versions of the same model, are tasked with generating responses to a given problem. These responses are then iteratively refined through a series of debates, where LLMs build upon each other’s outputs. https://arxiv.org/html/2501.05707v1#:~:text=Multiagent debate (Du et al.%2C 2023) involves a,versions of the same model—&text=2.4 Multiple Iterations of Finetuning,-Report issue for&The finetuned models are capable of generating responses through multiagent debate.
- Specialized Fine-Tuning: Each LLM is then fine-tuned on its own generated data, leading to specialization in specific aspects of the task. https://arxiv.org/html/2501.05707v1#:~:text=We then introduce our approach,on its own generated data.
Results:The researchers conducted experiments on both open-source and proprietary LLMs, demonstrating the effectiveness of multiagent fine-tuning across various reasoning tasks. The specialized agents consistently outperformed single-agent fine-tuned models, showcasing the benefits of collaborative training.
https://arxiv.org/html/2501.05707v1
Multiagent fine-tuning presents a promising avenue for enhancing LLM performance and diversity. By fostering collaboration among LLMs, this approach unlocks new possibilities for creating more robust and versatile AI systems.
Figure 1: A visual representation of the multiagent fine-tuning process.
Figure 2: Performance comparison between single-agent and multiagent fine-tuned LLMs on a reasoning task.
Video about Multi-agent Fine Tuning:
Key Sections:
Problem Statement:
- Traditional fine-tuning approaches show decreased performance gains after multiple rounds
- Single-agent systems face limitations in knowledge improvement
- Need for a new approach to maintain consistent performance improvements
Multi-Agent Framework:
- System uses five AI agents starting from identical base models
- Includes a “boss” agent (critique model) for feedback and integration
- Implements debate-style interaction between agents
- Uses majority voting to create training datasets
- Incorporates supervised fine-tuning for all agents, including the critique model
Implementation Details:
- Uses standard benchmark datasets (arithmetic, GSM, and Math)
- Requires 4 H100 NVIDIA GPUs with 240GB GPU memory
- Inference runs take 12-24 hours
- Fine-tuning process estimated at approximately 10 days
Results and Performance:
- Shows improvement from 57.5% to 67% accuracy on math datasets
- Demonstrates better generalization across different task types
- Achieves 54% accuracy on GSM dataset when trained only on math problems
Limitations and Considerations:
- Questions about the role of multiple inference runs versus actual learning
- Potential limitations in knowledge domains outside of training scope
- Uncertainty about genuine reasoning improvements versus probabilistic distribution effects
Impact to South East Asia for the next 5 years, Specialy Singapore and Malaysia
The advancements in multi-agent fine-tuning for language models (LLMs) have the potential to significantly impact Southeast Asia, particularly Singapore and Malaysia, over the next 5 years. Here are some key areas:
1. Enhanced Language Technologies:
- Improved Language Translation and Understanding: More accurate and nuanced translation services will facilitate cross-border communication and trade within the region. This can break down language barriers in business, education, and tourism.
- Advanced Chatbots and Virtual Assistants: Businesses can leverage AI-powered customer service, leading to improved efficiency and customer satisfaction. This can be particularly beneficial for sectors like tourism and e-commerce.
- Personalized Education: LLMs can be used to create personalized learning experiences, adapting to individual student needs and learning styles. This can help address educational disparities and improve learning outcomes.
2. Economic Growth and Innovation:
- Automation and Productivity: The automation of repetitive tasks can free up human workers for more creative and strategic roles, boosting productivity across various sectors.
- New Business Opportunities: The development of innovative LLM-powered applications can create new businesses and job opportunities, particularly in the technology and AI sectors.
- Improved Decision-Making: LLMs can analyze large datasets and provide valuable insights to businesses and governments, enabling better decision-making and policy formulation.
3. Social and Cultural Impact:
- Preservation of Languages and Cultures: LLMs can be used to preserve endangered languages and cultures by creating digital archives and developing language learning tools.
- Increased Accessibility to Information: LLMs can help bridge the digital divide by providing access to information and services in local languages.
- Enhanced Creativity and Innovation: LLMs can be used as creative tools, assisting artists, writers, and musicians in their work and inspiring new forms of artistic expression.
Specific Considerations for Singapore and Malaysia:
- Multilingualism: Both countries are multilingual societies, making advancements in LLM technology particularly relevant.
- Focus on Education: Singapore and Malaysia have strong education systems, and LLMs can play a crucial role in enhancing the quality of education and preparing students for the future.
- Economic Diversification: Both countries are seeking to diversify their economies, and LLMs can be a key driver of innovation and economic growth.
Challenges and Considerations:
- Ethical Concerns: It is crucial to address ethical concerns related to bias, fairness, and the responsible use of AI.
- Data Privacy and Security: Ensuring the privacy and security of data used to train and deploy LLMs is paramount.
- Job Displacement: The automation of certain tasks may lead to job displacement, requiring proactive measures to reskill and upskill the workforce.
Overall, the advancements in multi-agent fine-tuning for LLMs present both significant opportunities and challenges for Southeast Asia, particularly Singapore and Malaysia. By embracing these technologies responsibly and addressing the associated challenges, these countries can harness the power of AI to drive economic growth, improve social well-being, and enhance the quality of life for their citizens.
Conclusion:
In conclusion, advancements in multi-agent fine-tuning for Large Language Models (LLMs) hold immense potential for Southeast Asia, particularly in countries like Singapore and Malaysia. This technology can significantly impact various sectors, including education, business, and culture.
- Enhanced Language Technologies: Improved translation services, advanced chatbots, and personalized education are just a few examples of how LLMs can benefit the region.
- Economic Growth: Increased productivity, new business opportunities, and improved decision-making capabilities are key economic drivers that LLMs can facilitate.
- Social and Cultural Impact: LLMs can play a vital role in preserving languages and cultures, increasing access to information, and fostering creativity.
However, it’s crucial to acknowledge and address the challenges that come with this technology.
- Ethical Concerns: Issues like bias and fairness must be carefully considered and mitigated.
- Data Privacy and Security: Robust measures are needed to protect sensitive data used to train and deploy LLMs.
- Job Displacement: The automation of certain tasks may require proactive measures to reskill and upskill the workforce.
By embracing these technologies responsibly and strategically, Southeast Asian nations can leverage the power of AI to drive economic growth, improve social well-being, and enhance the quality of life for their citizens. This requires a multi-faceted approach that includes:
- Investing in AI research and development: Supporting research institutions and fostering collaborations between academia and industry.
- Developing a skilled AI workforce: Investing in education and training programs to equip the workforce with the necessary skills.
- Establishing ethical guidelines and regulations: Ensuring the responsible and ethical development and deployment of AI technologies.
- Promoting public awareness and engagement: Educating the public about the potential benefits and risks of AI.
Key Takeaways:
- Novel approach to multi-agent fine-tuning shows promise for improving LLM performance
- Framework enables specialization and diversity across agent responses
- Method potentially mitigates limitations of single-agent self-improvement
- Questions remain about the true nature of the performance improvements
- System requires significant computational resources
Related References:
- Improving Factuality and Reasoning in Language Models through Multi-Agent Debate
- Standard benchmark datasets: GSM, Math, and arithmetic testing sets
- LLM memorization
This article explains a complex new approach to LLM improvement while raising important questions about the nature and limitations of the achieved performance gains.