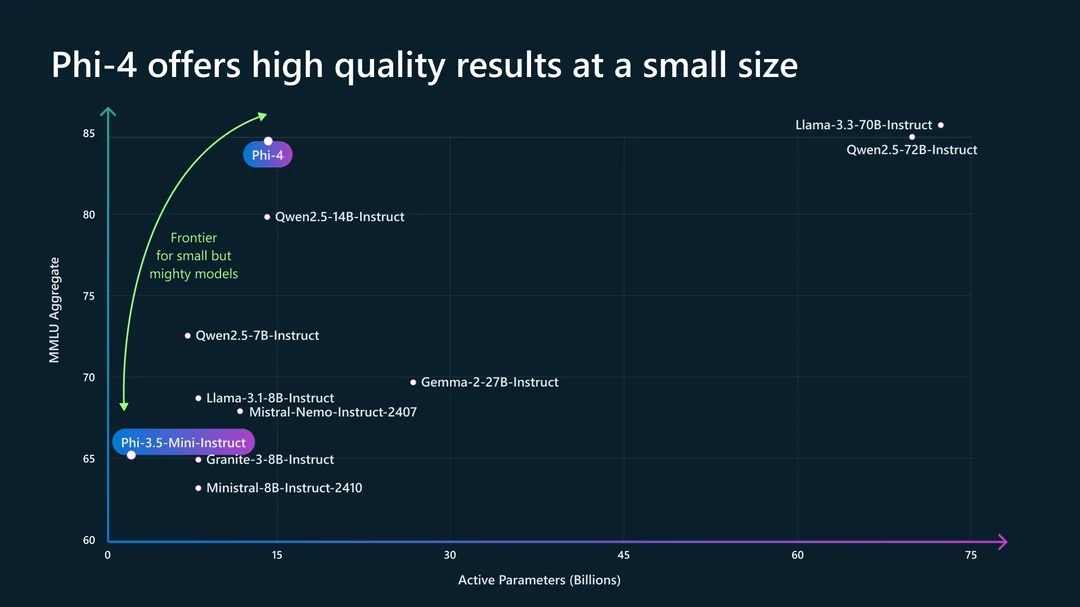
Introduction:
Microsoft recently released Phi-4, a 14 billion parameter language model. While Microsoft claims it outperforms GPT-4 and Claude 3.5 Sonnet in mathematics, the review examines its actual capabilities and limitations when running locally through Ollama.
Understanding Phi-4 and Ollama:
- Phi-4: A powerful language model developed by Microsoft Research, known for its efficiency and performance.
- Ollama: An open-source framework designed for running large language models (LLMs) on local machines.
Steps to Run Phi-4 with Ollama:
- Hardware Requirements: Ensure your system meets the demanding hardware requirements of Phi-4. This typically includes a powerful GPU (like an NVIDIA RTX 4090 or equivalent) with ample VRAM, a high-end CPU, and sufficient RAM.
- Ollama Installation: Follow the official Ollama installation instructions for your operating system.
- Model Download: Obtain the Phi-4 model weights. These are usually available through unofficial channels or shared within research communities.
- Ollama Configuration: Configure Ollama to load and run the Phi-4 model. This may involve specifying the model’s path, adjusting settings for optimal performance, and allocating sufficient resources.
- Testing and Usage: Once set up, you can start interacting with the locally running Phi-4 model through Ollama’s interface.
Important Considerations:
- Hardware Limitations: Running large models like Phi-4 locally can be resource-intensive. Be prepared for potential performance bottlenecks if your hardware doesn’t meet the model’s requirements.
- Model Availability: Accessing the Phi-4 model weights might involve navigating unofficial sources or research communities.
- Technical Expertise: Setting up and running LLMs locally requires some technical proficiency.
- Legal and Ethical Implications: Using models without proper authorization or for inappropriate purposes can have legal and ethical consequences.
Additional Tips:
- Refer to the Ollama documentation and community forums for detailed guidance and troubleshooting assistance.
- Consider starting with smaller Phi models or other LLMs supported by Ollama to gain experience before attempting to run Phi-4.
- Regularly update Ollama and your system to benefit from the latest performance optimizations and bug fixes.
Video About Phi-4 on Local Ollana:
Key Sections:
- Installation and Setup
- Can be run through Ollama locally
- Accessible via terminal or OpenWebUI interface
- Requires moderate system resources (16GB RAM minimum)
- Mathematical Capabilitie
- Shows strong chain-of-thought reasoning
- Performs well on word problems and puzzle solving
- Less accurate on direct calculations compared to Claude 3.5
- Good at explaining mathematical reasoning steps
- Problem-Solving Abilities
- Excels at structured problems like Sudoku and tic-tac-toe
- Demonstrates logical thinking and step-by-step analysis
- Sometimes misses details despite good reasoning approach
- Limitations
- Lacks function calling capabilities
- No inference-time compute support
- Not as strong in coding as Claude 3.5 or GPT-4
- Basic roleplay capabilities, less personality than Llama models
- Coding Performance
- Adequate for basic JavaScript and Python tasks
- Can handle simple React applications
- Struggles with complex code fixing and specific requirements
Step-by-step instructions for installing and running Phi-4 on Ollama.
Prerequisites:
Make sure you have Ollama installed on your system
- For Mac/Linux:
curl -fsSL <https://ollama.com/install.sh> | sh
- For Windows:
Download from https://ollama.com/download
Step 1: Installing Phi-4
- Open your terminal or command prompt
- Search for Phi-4 model:
ollama list
- Currently, you’ll need to pull the vanel/phi-4 model:
ollama pull vanel/phi-4
For better performance with more RAM, you can use the Q8_0 version:ollama pull vanel/phi-4-q8_0
Step 2: Running Phi-4 via Terminal
- Basic usage:
ollama run vanel/phi-4
- This will start an interactive chat session where you can type queries
Step 3: Setting up OpenWebUI (Optional but recommended)
- Install Docker if you haven’t already (from docker.com)
- Pull the OpenWebUI Docker image:
docker pull ghcr.io/open-webui/open-webui:main
- Run OpenWebUI:
docker run -d -p 3000:8080 -v open-webui:/app/backend/data --network host ghcr.io/open-webui/open-webui:main
Step 4: Accessing OpenWebUI
- Open your web browser
- Go to:
http://localhost:3000
- In the model selection, choose “vanel/phi-4” or “phi-4” (once officially released)
Step 5: Optimizing Performance (Optional)
- Set environment variables for better performance:
export OLLAMA_HOST=0.0.0.0:11434
- Adjust system resources in Docker settings if needed
- Recommended: At least 16GB RAM allocation
- More RAM will improve response speed and quality
Verification and Testing:
- Test the installation with a simple query:
ollama run vanel/phi-4 "What is 2+2?"
- Try a more complex mathematical problem to test reasoning:
ollama run vanel/phi-4 "If a store sells apples for $1 each and oranges for $1.50 each, and you have $10, how many of each can you buy if you want twice as many apples as oranges?"
Troubleshooting Tips:
- If Ollama isn’t responding:
sudo systemctl restart ollama
- Check Ollama status:
ollama ps
- Clear cache if needed:
ollama rm vanel/phi-4 ollama pull vanel/phi-4
Important Note to Remember:
- Keep Ollama updated for best performance
- The model name might change to simply “phi-4” when officially released
- Performance depends on your system’s capabilities
- The first run might take longer as it downloads and initializes the model
Conclusion:
Phi-4 is impressive for its size (14B parameters) but doesn’t surpass Claude 3.5 or GPT-4. Its strength lies in chain-of-thought reasoning, but it’s limited by lack of function calling and inference-time compute capabilities.
Key Takeaways:
- Excellent chain-of-thought reasoning for its size
- Good for basic enterprise tasks like summarization and RAG
- Potential for agent-based workflows if enhanced with function calling
- Best suited for simpler tasks rather than complex coding or specialized domains
- Shows promise but needs further development to compete with larger models