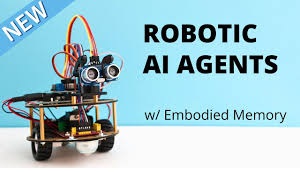
Introduction:
This article delves into cutting-edge research on robotic AI agents equipped with novel embodied memory constructs, with a particular focus on their potential applications in disaster response scenarios. It provides an in-depth exploration of how these advanced technologies can significantly enhance and streamline rescue operations in complex, dynamic, and often hazardous environments. The research specifically addresses situations where traditional communication networks may be compromised or entirely non-functional, highlighting the crucial role of autonomous AI agents in such challenging circumstances.
The study examines the integration of sophisticated memory systems within AI agents, enabling them to navigate, process information, and make decisions independently. This autonomous capability is especially vital in disaster zones where external guidance may be limited or unavailable. The article further investigates how these AI agents can effectively collect, analyze, and utilize real-time data to support and augment human rescue efforts, potentially saving more lives and improving overall disaster response efficiency.
Robotic AI Agents with Embodied Memory for Disaster Response
The integration of embodied memory constructs into robotic AI agents holds significant promise for enhancing their capabilities in disaster response scenarios. Embodied memory, inspired by biological memory systems, allows agents to store and retrieve information based on their physical interactions with the environment. This enables them to learn from their experiences, adapt to changing conditions, and make more informed decisions in complex and dynamic environments.
Potential Applications of Embodied Memory in Disaster Response
- Situational Awareness and Mapping:
- Dynamic Map Creation: Embodied memory can help agents create and update real-time maps of disaster-affected areas, incorporating information from sensors, visual data, and physical interactions.
- Object Recognition and Tracking: Agents can learn to identify and track critical objects like survivors, debris, or hazardous materials through their embodied experiences.
- Search and Rescue Operations:
- Efficient Search Patterns: Embodied memory can enable agents to develop efficient search strategies based on past experiences and environmental cues.
- Human-Robot Interaction: Agents can use embodied memory to understand and respond to human cues and gestures, improving collaboration during rescue efforts.
- Infrastructure Assessment and Damage Evaluation:
- Structural Analysis: Embodied memory can help agents assess the structural integrity of buildings and infrastructure by analyzing their physical interactions and sensor data.
- Damage Classification: Agents can learn to classify different types of damage (e.g., collapse, fire, flooding) based on their embodied experiences.
- Hazardous Material Handling:
- Safe Navigation: Embodied memory can assist agents in navigating hazardous environments by learning to avoid obstacles and identify potential dangers.
- Material Identification: Agents can use embodied memory to identify and classify hazardous materials based on their physical properties and sensory data.
- Long-Term Autonomy:
- Adaptability: Embodied memory can help agents adapt to changing conditions and learn from their mistakes, enabling them to operate autonomously for extended periods.
- Resilience: Embodied memory can make agents more resilient to failures and damage, allowing them to continue their missions even in challenging circumstances.
Video about Embodied RAG:
Related Sections of the above video:
- Disaster Response Phases:
- Reconnaissance and Geo-mapping
- Knowledge Sharing and Coordinated Response
- Enhancing Human Rescue Efforts
- Technological Components:
- Topological Map Construction
- Hierarchical Semantic Forest
- Non-parametric Embodied Memory
- Implementation Details:
- In-agent Memory System
- Multi-modal Data Collection
- Vision Language Model Integration
- Retrieval and Generation Process:
- Graph-based Knowledge Representation
- Hierarchical Clustering for Semantic Understanding
- Query Processing and Response Generation
- Challenges and Future Directions:
- Advanced Multi-sensor Data Fusion
- Multi-perspective Correlation
- Temporal Deviation Handling
Key Challenges and Future Directions
- Data Integration and Fusion: Effectively integrating data from various sensors and sources into embodied memory systems is a critical challenge.
- Scalability: Developing embodied memory systems that can handle large amounts of data and complex environments is essential for real-world applications.
- Ethical Considerations: Ensuring the ethical use of robotic AI agents with embodied memory in disaster response scenarios is crucial, particularly regarding privacy, safety, and accountability.
By addressing these challenges and leveraging the potential of embodied memory, robotic AI agents can play a vital role in enhancing disaster response efforts, saving lives, and minimizing damage.
Conclusion and Key Takeaways:
The cutting-edge approach to embodied AI for disaster response, combining topological mapping with hierarchical semantic understanding. This technology enables AI agents to navigate, comprehend, and respond effectively in complex environments without relying on external networks. The system’s ability to construct and utilize non-parametric memory for both navigation and language generation represents a significant advancement in embodied AI.
Key points include:
- The importance of in-agent memory for autonomous operation in disaster scenarios
- The combination of topological mapping and semantic understanding for enhanced situational awareness
- The potential for improved coordination and efficiency in rescue operations
- The need for further research in areas such as multi-sensor fusion and temporal data handling
Related References:
- Research paper on “General Non-parametric Embodied Memory for Retrieval and Generation”
- Stanford University’s work on Multiscale Insight Agents for Advanced AI Reasoning
- DARPA (Defense Advanced Research Projects Agency) funded research
- Lockheed Martin Corporation’s partial funding of the research
- NetworkX software for implementing topological maps
- Robot Operating System (ROS) for potential implementation