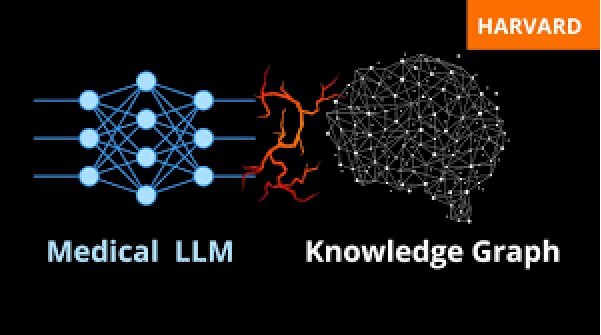
Introduction:
Here the discusses of a new methodology developed by Harvard University to address the challenges faced by large language models (LLMs) in medical reasoning and question-answering. The approach combines LLMs with knowledge graphs to improve accuracy and performance in complex medical tasks.
Harvard University in R&D to address challenges faced by large language models to improve Medical Tasks
Harvard University is actively engaged in research and development to address the challenges faced by large language models (LLMs) in medical reasoning and question-answering. Here are some notable areas of focus:
1. Improving medical knowledge representation and reasoning:
- Developing specialized LLMs for medical domains: Harvard researchers are working on creating LLMs that are specifically trained on large medical datasets, such as electronic health records, medical literature, and clinical guidelines. These specialized models can better understand and reason about complex medical concepts and scenarios.
- Enhancing reasoning capabilities: Researchers are exploring techniques to improve LLMs’ ability to reason about medical information, including chain-of-thought prompting, reinforcement learning, and knowledge graph integration. This involves teaching LLMs to break down complex problems into smaller steps, consider multiple perspectives, and draw logical conclusions based on the available evidence.
2. Ensuring safety and reliability:
- Addressing bias and fairness: Harvard researchers are investigating methods to mitigate bias in LLMs, such as debiasing techniques and careful data curation. This is crucial to ensure that LLMs provide fair and unbiased medical advice.
- Evaluating model performance: Researchers are developing rigorous evaluation frameworks to assess the performance of LLMs in medical tasks, including accuracy, reliability, and explainability. This helps to identify potential limitations and areas for improvement.
3. Enhancing human-AI collaboration:
- Developing effective human-AI interfaces: Harvard researchers are exploring ways to design intuitive and user-friendly interfaces that facilitate collaboration between clinicians and LLMs. This includes developing tools that can help clinicians understand and interpret LLM outputs, as well as provide feedback to improve model performance.
4. Ethical considerations:
- Addressing privacy and security concerns: Harvard researchers are working to ensure that the use of LLMs in healthcare complies with privacy and security regulations. This involves developing techniques to protect patient data and prevent unauthorized access.
- Considering ethical implications: Researchers are also exploring the ethical implications of using LLMs in healthcare, including issues such as accountability, transparency, and the potential for unintended consequences.
5. Example: Diagnosing a Rare Disease:
Consider a hypothetical scenario where an LLM is tasked with diagnosing a rare disease based on a patient’s symptoms. Without a knowledge graph, the LLM might struggle to connect the dots between seemingly unrelated symptoms or consider rare differential diagnoses.
By integrating a medical knowledge graph, the LLM can:
- Identify relevant entities: The model can extract key entities from the patient’s symptoms (e.g., fever, rash, fatigue) and search the knowledge graph for related concepts.
- Explore potential diagnoses: The knowledge graph can suggest potential diagnoses based on the identified symptoms and their relationships.
- Consider rare conditions: The LLM can explore less common diagnoses that might be overlooked without the support of a knowledge graph.
- Provide explanations: The model can trace its reasoning process through the knowledge graph, providing a more transparent explanation of how it arrived at a particular diagnosis.
Overall, Harvard University is at the forefront of research aimed at harnessing the potential of LLMs to improve medical care while addressing the challenges and risks associated with their use. By advancing our understanding of LLMs and developing innovative solutions, Harvard researchers are helping to pave the way for the safe and effective integration of AI into the healthcare system.
Video about the discussion:
Related Sections:
- Problem Statement:
- LLMs struggle with incorrect retrieval, missing key information, and misalignment with scientific and medical knowledge.
- Existing models lack multi-source and grounded knowledge necessary for medical reasoning.
- Current retrieval augmented generation (RAG) methods are vulnerable to errors and lack post-retrieval verification mechanisms.
- Methodology:
- Knowledge Graph-based Agent (KGA) for complex knowledge-intensive question answering in medicine.
- Four-phase approach: Generate, Review, Revise, and Answer.
- Integration of LLMs with domain-specific knowledge graphs.
- Use of UMLS (Unified Medical Language System) codes for entity mapping.
- Alignment of LLM token embeddings with knowledge graph structural embeddings.
- Key Components:
- Triplet generation for medical concepts and relationships.
- Knowledge graph completion tasks for fine-tuning the LLM.
- Error correction and revision mechanism.
- Combination of semantic and structural knowledge for improved reasoning.
- Results:
- Improved performance compared to baseline LLMs, especially for questions involving multiple medical concepts.
- Varying effectiveness depending on the underlying LLM used (e.g., Llama 3.8B vs. Llama 3.1).
Impact of Combining LLMs with Knowledge Graphs on Medical Care in Southeast Asia
The integration of large language models (LLMs) with knowledge graphs has the potential to significantly impact medical care in Southeast Asia in several ways:
1. Improved Access to Medical Information:
- Remote Consultation: LLMs can provide real-time medical information and advice to patients in remote areas, reducing the need for long-distance travel to seek care.
- Language Barriers: LLMs can be trained on multiple languages, making medical information accessible to patients who may not speak the dominant language.
2. Enhanced Disease Diagnosis and Treatment:
- Accurate Diagnosis: LLMs can assist in diagnosing diseases by analyzing patient symptoms and medical history, comparing them to known disease patterns stored in knowledge graphs.
- Personalized Treatment Plans: By understanding a patient’s unique medical history and genetic makeup, LLMs can help recommend personalized treatment plans.
3. Improved Medical Education:
- Interactive Learning: LLMs can create interactive learning experiences for medical students and healthcare professionals, providing personalized feedback and answering questions.
- Knowledge Dissemination: LLMs can help disseminate the latest medical research and best practices to healthcare providers across the region.
4. Drug Discovery and Development:
- Accelerated Research: LLMs can analyze vast amounts of biomedical data to identify potential drug targets and accelerate the drug discovery process.
- Drug Repurposing: By understanding the mechanisms of action of existing drugs, LLMs can help identify new uses for these drugs in treating different diseases.
5. Addressing Healthcare Disparities:
- Bridging the Gap: LLMs can help bridge the gap in healthcare access between urban and rural areas, as well as between developed and developing countries.
- Cost-Effective Solutions: By providing affordable and accessible healthcare services, LLMs can help address healthcare disparities in Southeast Asia.
Challenges and Considerations:
- Data Quality: The quality and completeness of the knowledge graph are crucial for accurate and reliable results.
- Language Barriers: While LLMs can be trained on multiple languages, there may still be challenges in accurately interpreting and translating medical information.
- Ethical Considerations: The use of AI in healthcare raises important ethical questions, such as privacy, bias, and accountability.
Despite these challenges, the potential benefits of combining LLMs with knowledge graphs for medical care in Southeast Asia are significant. By addressing these challenges and leveraging the power of AI, we can improve healthcare outcomes and enhance the quality of life for millions of people in the region.
Conclusion and Key Takeaways:
- The KGA methodology enhances medical question-answering by leveraging LLMs grounded with knowledge graph information.
- Unlike standard RAG approaches, this method uses the LLM to propose potential relationships between medical concepts.
- The system shows improved accuracy, especially for complex queries involving multiple medical concepts.
- The methodology’s effectiveness varies depending on the underlying LLM, suggesting potential for further improvement with more advanced models.
- This approach offers a promising direction for combining the strengths of LLMs and structured knowledge in the medical domain.
Related References:
- Harvard University and Pfizer collaboration on the study.
- TransE paper for translating embeddings in multi-relational data.
- UMLS (Unified Medical Language System) for standardized medical terminology.
Hope this article helps you understand the impact of AI on the Medical Industry.