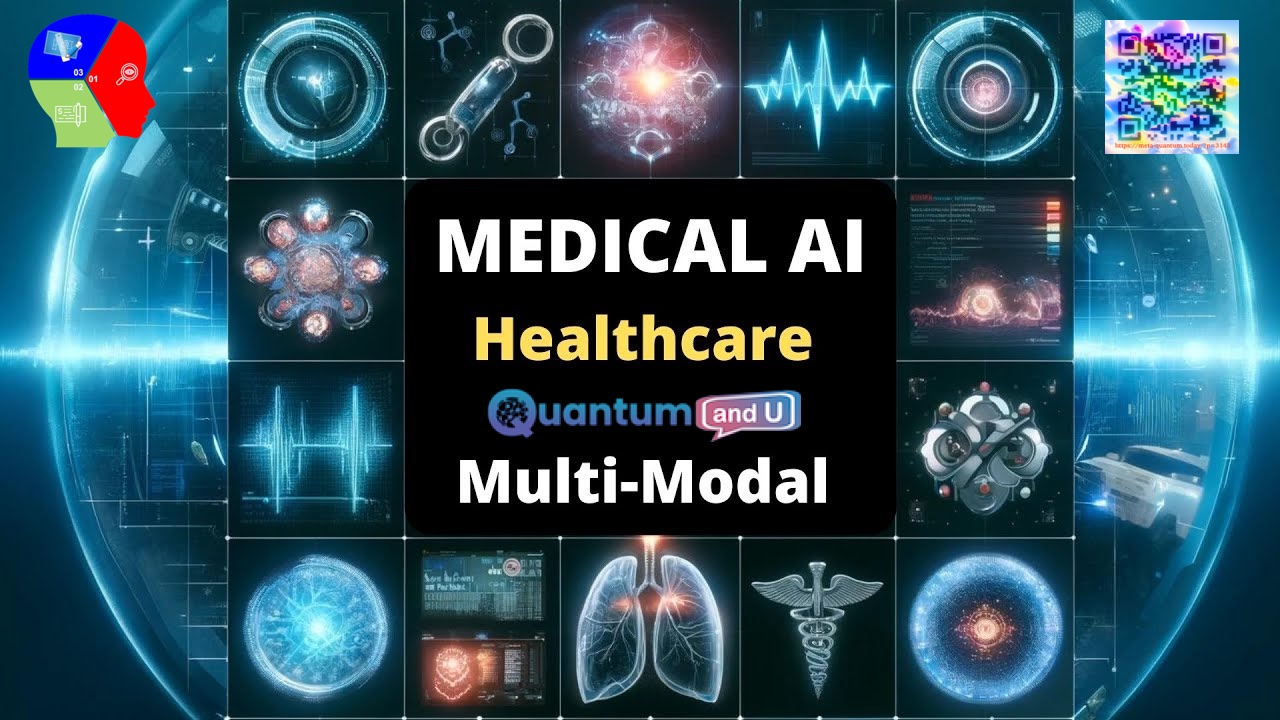
Introduction:
This document delves into the capabilities of large multimodal AI models (LMMs) within the context of medical diagnosis. We’ll explore a study that gauges these models’ performance and reliability when dealing with challenging questions in medical vision and answering tasks. This study also introduces a new dataset, “ProbMed”, specifically designed to rigorously evaluate the capabilities of LMMs in the medical field.
Applying Adversarial Questions to Test Multimodal MED AI Systems
Adversarial question testing goes beyond simply asking tricky questions to a multimodal MED AI system. It’s a structured approach to identify weaknesses and assess the system’s ability to handle real-world complexities. Here’s a breakdown of its application:
I. Design and Development:
- Data Pool Creation: Develop a diverse data pool encompassing various combinations of medical data: images, reports, lab results, etc. This data can include real-world scenarios with ambiguities and inconsistencies.
- Question Formulation: Craft adversarial questions that target specific vulnerabilities. These questions can:
- Introduce irrelevant or misleading information alongside relevant data.
- Highlight potential biases in the training data.
- Present contradictory or ambiguous findings across modalities.
- Simulate unexpected or noisy data often encountered in real-world situations.
II. Implementation and Analysis:
- Present the system with the data and corresponding adversarial questions.
- Analyze the system’s responses:
- Accuracy: Did the system arrive at the correct diagnosis or prediction despite the challenges?
- Reasoning: Can the system explain its reasoning process and justify its conclusions?
- Sensitivity: Does the system’s performance significantly drop when faced with adversarial questions compared to standard tests?
III. Iteration and Improvement:
- Based on the analysis, identify areas where the system struggles.
- Refine the training data and algorithms to address the exposed weaknesses.
- Develop new adversarial questions to test the improvements and iterate on the testing process.
Benefits of Applying Adversarial Questions:
- Improved Robustness: Identifies and strengthens the system against adversarial attacks and unexpected data combinations.
- Reduced Bias: Exposes potential biases in the training data and helps to develop fairer AI models.
- Enhanced Generalizability: Ensures the system can handle situations beyond the training set, leading to more reliable real-world performance.
- Increased Trust: Provides a more rigorous testing methodology, fostering trust in the reliability of multimodal MED AI.
By applying adversarial questions, the develop more robust and trustworthy AI systems that can navigate the complexities of medical decision-making.
Video of Adversarial Question Test of Multimodal MED AI:
Related Sections:
- Personal Anecdote: A recent accident led me to undergo an MRI, sparking my curiosity about the reliability of medical AI systems. As I await the specialist’s analysis of my scans, I decided to investigate how well LMMs can assist in medical diagnoses, especially in critical scenarios.
- The “ProbMed” Dataset: The focus of today’s review is on a study from the University of California and Carnegie Mellon University, which introduced the “ProbMed” dataset. This dataset is designed to rigorously evaluate medical AI models by including questions that require reasoning across multiple diagnostic dimensions such as modality recognition, organ identification, and clinical findings.
- Evaluation of LMMs: The study used three classes of AI models: large foundation models like GPT-4 Vision and Gemini Pro, fine-tuned general domain models for biomedical fields, and narrow domain-specific models focused on specific clinical needs. The evaluation revealed significant weaknesses in these models, particularly when they were tested with adversarial questions.
- Adversarial Questions and Model Robustness: Adversarial questions were introduced to test the robustness and reliability of the AI models. These questions added a second, often misleading query alongside the primary question to see if the models could maintain accuracy. The results showed a dramatic drop in performance, highlighting the models’ sensitivity to increased cognitive load and their struggles with deeper reasoning.
- Performance Insights: The performance metrics were stark. For instance, the LLava-Med model’s accuracy dropped from over 80% to around 3% when faced with adversarial questions. This pattern was consistent across other models, indicating that current LMMs are not yet reliable enough for critical medical applications.
- Implications for Medical AI: These findings underscore the importance of developing more robust AI systems capable of understanding and reasoning through complex medical data. The study’s use of adversarial pairs effectively exposed vulnerabilities in the models’ logic and reasoning capabilities, suggesting a need for further advancements in AI training and evaluation methods.
Impact and Opportunities of Adversarial Question Testing in Southeast Asia’s MED AI
Adversarial question testing holds immense potential for Southeast Asia’s burgeoning MED AI landscape. Here’s how it can impact the region and the opportunities it presents:
Impact:
- Strengthening AI Adoption: Rigorous testing with adversarial questions can build trust in MED AI systems, leading to faster and wider adoption across Southeast Asia’s healthcare systems. This can improve access to advanced diagnostics and potentially save lives.
- Addressing Data Challenges: Southeast Asian countries often grapple with limited healthcare data and potential biases within that data. Adversarial questions can help develop AI systems robust to these challenges, leading to more equitable and inclusive healthcare delivery.
- Promoting Innovation: A focus on adversarial testing can foster a culture of innovation in Southeast Asia’s AI development. Researchers can design MED AI systems specifically tailored to the region’s diverse healthcare needs and data landscape.
Opportunities:
- Collaboration: Countries within Southeast Asia can collaborate to create shared data pools for adversarial question development. This can leverage regional expertise and accelerate AI advancements.
- Focus on Accessibility: Adversarial testing can ensure MED AI systems are accessible to underserved communities within Southeast Asia. This can involve tailoring question sets to address specific regional diseases and healthcare challenges.
- Building Local Expertise: Developing strong adversarial question testing methodologies can create high-demand skillsets within Southeast Asia. This can lead to the creation of new jobs and a more competitive AI workforce in the region.
Challenges:
- Data Availability: As mentioned earlier, limited healthcare data availability remains a hurdle. International collaborations and data anonymization techniques can help address this challenge.
- Regulatory Frameworks: Clear regulatory frameworks specific to AI in medicine are needed to ensure responsible development and deployment of MED AI systems in Southeast Asia.
- Infrastructure Development: Investment in computing infrastructure is crucial to support the complex data processing demands of adversarial question testing.
Conclusion:
IIn conclusion, large multimodal AI models’ current state in medical diagnosis is promising but flawed. The introduction of adversarial questions in the “ProbMed” dataset highlights significant challenges in these models’ complex reasoning tasks. As we continue developing and fine-tuning these systems, it’s vital to address these weaknesses to ensure their reliable assistance in critical medical scenarios. For those interested in exploring AI model fine-tuning and hallucination reduction further, consider my other videos on these topics.
By tackling these challenges and adopting adversarial question testing, Southeast Asia can position itself as a leader in responsible and impactful medical AI development. This could revolutionize healthcare delivery in the region and contribute to improved health outcomes for its population.
Takeaway Key Points:
- The virtual rat brain is a groundbreaking achievement that provides an unprecedented window into the neural mechanisms behind overt actions and covert cognitive processes.
- It opens up new possibilities for understanding real brain function, studying neurological conditions, and testing therapies or neural prosthetics in silico.
- This work has immense potential for revolutionizing robotic control by abstracting the principles of biological intelligence and porting them into new robotic platforms.
- The approach of combining high-fidelity physics modeling with machine learning in a differentiable programming framework represents a powerful paradigm for tackling complex systems analysis across various scientific domains.
- As these virtual world simulations become more realistic, they raise ethical questions about preventing self-awareness or existential risks, which will need to be addressed.
References:
- University of California and Carnegie Mellon University Study on “ProbMed” Dataset
- LLava-Med and Other Fine-Tuned Biomedical AI Models
Stay tuned for more insights and updates on the fascinating world of AI in medicine.