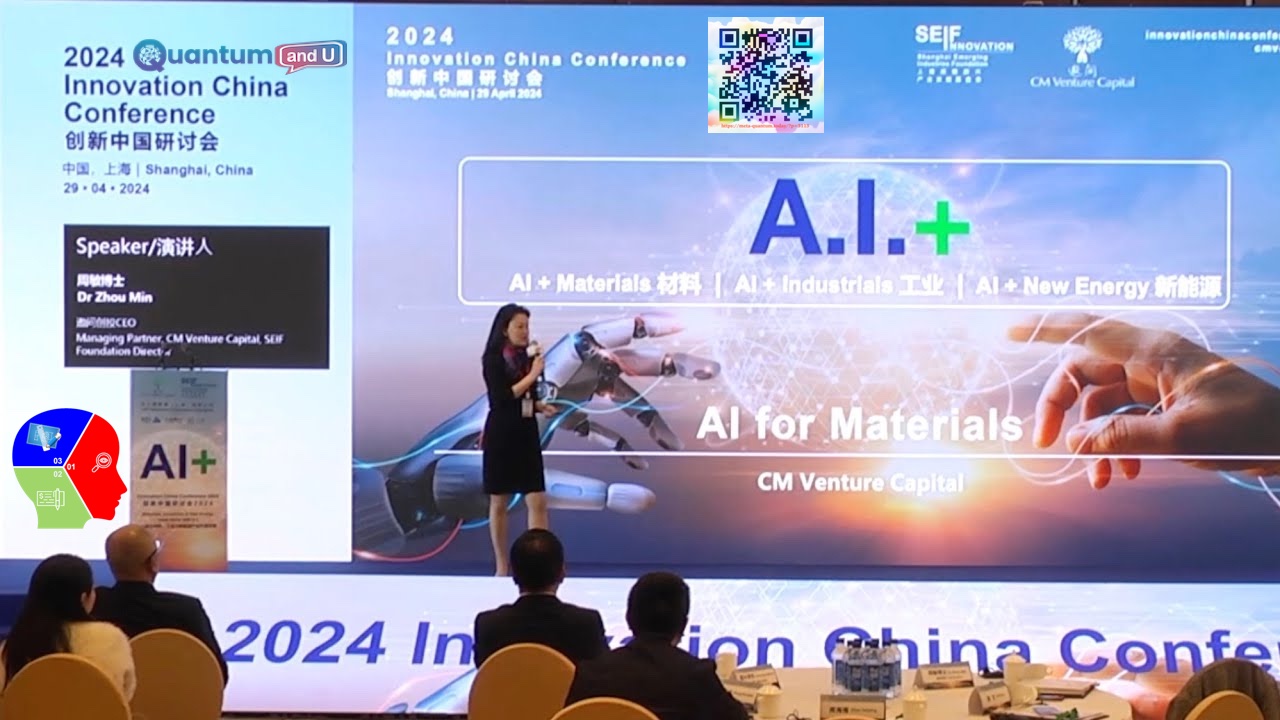
Introduction:
Let’s delve into an enlightening talk by Dr. Min Zhou titled “AI for Materials.” This presentation, part of the Innovation China conference, offered a comprehensive view of the intersection between artificial intelligence and material science.Dr. Zhou began by reflecting on the previous year’s discussion on synthetic biology and its Design-Build-Test-Learn (DBTL) cycle. This cycle is crucial in synthetic biology and mirrors the process in material discovery using AI. The DBTL cycle includes designing, building, testing, and learning from each iteration, which is vital for innovation in materials science.
AI For Materials:
Artificial intelligence (AI) is revolutionizing the field of materials science by offering new ways to design, discover, and develop materials. Here are some of the key areas where AI is having a major impact:
- Materials discovery: AI can be used to screen vast databases of materials to identify candidates with specific properties. This can significantly accelerate the discovery of new materials with desired characteristics, such as high strength, conductivity, or biocompatibility.
- Materials design: AI can be used to design new materials with tailored properties. This is done by training machine learning models on data from existing materials and then using these models to predict the properties of new materials.
- Materials optimization: AI can be used to optimize the properties of existing materials. This can be done by identifying the key factors that control a material’s properties and then using AI to find ways to improve those properties.
- Materials characterization: AI can be used to analyze data from experiments to characterize the properties of materials. This can help scientists to understand how materials behave and to develop new materials with improved performance.
More about SynBio, Click here!
Video: Dr Zhou presentation in 2024 Innovation China Conference:
Key Sections:
- Synthetic Biology (SynBio) and Material Discovery:
- Dr. Zhou revisits the concept of synthetic biology, mentioning the investment in startups like Noel Nutrients, which uses CO2 and hydrogen to produce synthetic proteins.
- The four levels of synthetic biology’s technological challenges are outlined, from wholesale synthesis to the most complex cell-free synthesis.
- He emphasizes the similarities between synthetic biology and material discovery, both of which follow a DBTL cycle.
- Challenges in Material Discovery:
- The presentation highlights the complexities in discovering new materials due to the multi-layered nature of materials, from microscopic to macroscopic levels.
- Dr. Zhou discusses the challenges in rapid experimentation and the necessity of high-quality experimental data, which is both valuable and costly.
- He mentions the need for a combination of public data, model-simulated data, and experimental data to achieve meaningful AI-driven discoveries.
- AI in Material Science:
- The talk covers the evolution of AI in material science, with a mention of significant projects like the Material Genome Project and Google’s AI-driven material discoveries.
- Dr. Zhou critiques some claims, emphasizing that new structures discovered by AI must be practically useful to be considered new materials.
- He underscores the importance of finding good applications along the value chain, noting the difficulties startups face in selecting the right materials to develop.
- Industry and Investment Insights:
- Dr. Zhou provides insights into the current state of the industry, noting that there are more platform companies than product companies in the AI-materials space.
- He shares examples of successful investments, such as Wiing Turtle, which uses AI to develop superalloys for satellite communication.
- The talk concludes with a positive outlook on the growth potential of AI in material science, forecasting a 30% annual growth rate in the next decade.
SynBio with AI: A Game Changer for Thailand?
The convergence of Synthetic Biology (SynBio) and Artificial Intelligence (AI) has the potential to be a game-changer for Southeast Asia, and Thailand is well-positioned to be a leader in this exciting field. Here’s a closer look at the impact, potential benefits, and business opportunities this holds for Thailand:
Positive Impacts:
- Addressing Thailand’s Challenges:
- Sustainable Agriculture: Thailand is a major agricultural producer. SynBio can help develop crops resistant to local diseases and pests, reducing reliance on pesticides and improving food security. AI can optimize farming practices for increased yield and resource efficiency.
- Waste Management: SynBio can engineer microbes to break down plastic waste and other environmental pollutants. AI can be used to identify the most effective organisms for specific waste types and optimize bioremediation processes.
- Public Health: SynBio holds promise for developing new drugs, vaccines, and diagnostics. AI can accelerate drug discovery and personalize medicine based on individual genetic profiles.
- Boosting Thailand’s Economy:
- Bio-Industry Hub: Thailand can become a hub for SynBio research and development, attracting international collaborations and investment. This can create high-skilled jobs and boost the bio-economy.
- Entrepreneurial Ecosystem: The emergence of SynBio startups will stimulate entrepreneurship and economic growth. Thailand’s strong tech startup scene can be a launchpad for innovative SynBio companies.
- Export Potential: Thailand can develop and export bio-based materials, biofuels, and other SynBio products, creating new revenue streams.
Business Opportunities in Thailand:
- Biorefineries: Investing in facilities to produce bioplastics, biofuels, or other bio-based products using SynBio can be a lucrative venture.
- AI for SynBio Design: Developing AI tools and services specifically tailored for SynBio applications in areas like protein design or genetic circuit optimization presents a significant opportunity.
- Precision Agriculture Merging SynBio innovations with AI-powered agricultural solutions can create a powerful market for Thai companies.
- Biomedical Research: Thailand can leverage its existing strengths in healthcare and research to become a leader in SynBio-based medical advancements.
Challenges and Considerations:
- Regulatory Framework: Thailand needs to develop a clear and supportive regulatory framework for SynBio to ensure safe and responsible development.
- Infrastructure and Talent: Investments in research infrastructure, training programs, and attracting skilled professionals in SynBio and AI are crucial.
- Public Engagement: Open communication and public education about SynBio’s benefits and potential risks are essential for building trust and social acceptance.
Conclusion:
In conclusion, Dr. Min Zhou’s presentation skillfully navigates the intersection of AI and material science. The main takeaways are the complex challenges of material discovery, the crucial need for high-quality data, and the significance of selecting appropriate applications. Even with these challenges, the field is on the brink of significant growth and innovation.
While AI is in the nascent stage of development in materials science, it holds potential to revolutionize the field. With AI, scientists can create novel materials with unprecedented properties. This could catalyze breakthroughs across various sectors, including medicine, energy, aerospace, and transportation.
Thailand possesses a unique opportunity to utilize SynBio in tandem with AI to address its challenges, foster a sustainable bio-economy, and emerge as a regional leader in this transformative field. By promoting innovation, attracting talent, and cultivating a supportive regulatory environment, Thailand can tap into the vast potential of SynBio and AI for a brighter future.
Takeaway Points:
- The “design-build-test-learn” cycle is crucial in both synthetic biology and material science.
- Scaling deep learning for materials discovery
- The Importance of High-Quality Reagents in Accurate …
- Materials Selection in Product Development: Challenges …
- Millions of new materials discovered with deep learning
About SynBio and Materials Synthetic Biology
Synthetic biology (SynBio) is joining forces with materials science to create a new field called materials synthetic biology. This exciting area focuses on using the power of living organisms to design and manufacture novel materials or improve existing ones.
How SynBio is shaking things up in materials science:
- Bio-based manufacturing:
Synbio allows engineering of organisms like bacteria or yeast to produce precursors for materials. These precursors can be used to create bioplastics, biofuels, or even sustainable alternatives to traditionally produced materials. - Living factories:
Scientists can design organisms to act as tiny factories, producing materials with specific properties. Imagine bacteria programmed to synthesize self-healing concrete or glowing fabrics! - Biodegradation and sustainability:
SynBio offers a path to producing materials that are biodegradable or can be broken down by natural processes. This can significantly reduce dependence on non-renewable resources and contribute to a more sustainable future. - Responsive Materials:
Synthetic biology allows for the creation of materials that respond to their environment. For instance, materials that change color with temperature or self-repair when damaged are becoming a possibility.
The potential applications of SynBio in materials science are vast and constantly evolving. Some areas of ongoing research include:
- Biofabrication:
Using 3D printing techniques with bio-inks derived from living cells to create tissues and organs for medical applications. - Self-healing materials:
Developing materials that can autonomously repair damage, inspired by the regenerative abilities of biological systems. - Bioelectronics:
Integrating biological components with electronic devices to create novel sensors, actuators, and even biocomputers.
SynBio using AI in its development
Synthetic biology (SynBio) and Artificial Intelligence (AI) are becoming a powerful duo, accelerating progress in the field. Here’s how AI is supercharging SynBio:
1. Design and Optimization:
- Protein Design: AI can analyze massive datasets of protein structures and functions, allowing for the design of entirely new proteins with desired properties. This could lead to novel enzymes, biocatalysts, or even custom-built materials.
- Genetic Circuit Design: SynBio often involves engineering complex genetic circuits within organisms. AI can predict how these circuits will behave and optimize them for efficiency and desired outcomes.
- Strain Engineering: AI can analyze data on different strains of organisms and predict which ones are best suited for specific SynBio applications. This streamlines the development process and saves valuable time and resources.
2. Data Analysis and Modelling:
- Massive Data Management: SynBio experiments generate huge amounts of data. AI can sift through this data, identify patterns, and make predictions about biological systems. This allows researchers to gain deeper insights and make more informed decisions.
- Predictive Modeling: AI can be used to create models that predict the behavior of biological systems under different conditions. This allows SynBio researchers to virtually test their designs before actual experimentation, saving time and resources.
- Process Optimization: AI can analyze data from fermentation and production processes in SynBio. This helps identify ways to optimize these processes for increased efficiency and yield.
3. Automation and Exploration:
- Automated Design: AI can automate some aspects of SynBio design, freeing up researchers to focus on more complex tasks. This can significantly accelerate the design cycle.
- Unexplored Design Space: AI can explore vast design spaces that would be impossible to navigate manually. This allows researchers to discover novel and potentially groundbreaking solutions.
Challenges and the Future:
While the potential of AI in SynBio is immense, there are challenges. These include:
- Data Quality and Availability: Effective AI models require high-quality and readily available data. This is an ongoing area of focus for the SynBio community.
- Explainability and Trust: Understanding how AI models arrive at their conclusions is crucial for building trust in their predictions. Researchers are working on developing more transparent AI models for SynBio.
Despite these challenges, the future of SynBio with AI is incredibly bright. This powerful combination has the potential to revolutionize various fields, from medicine and materials science to biofuels and agriculture.