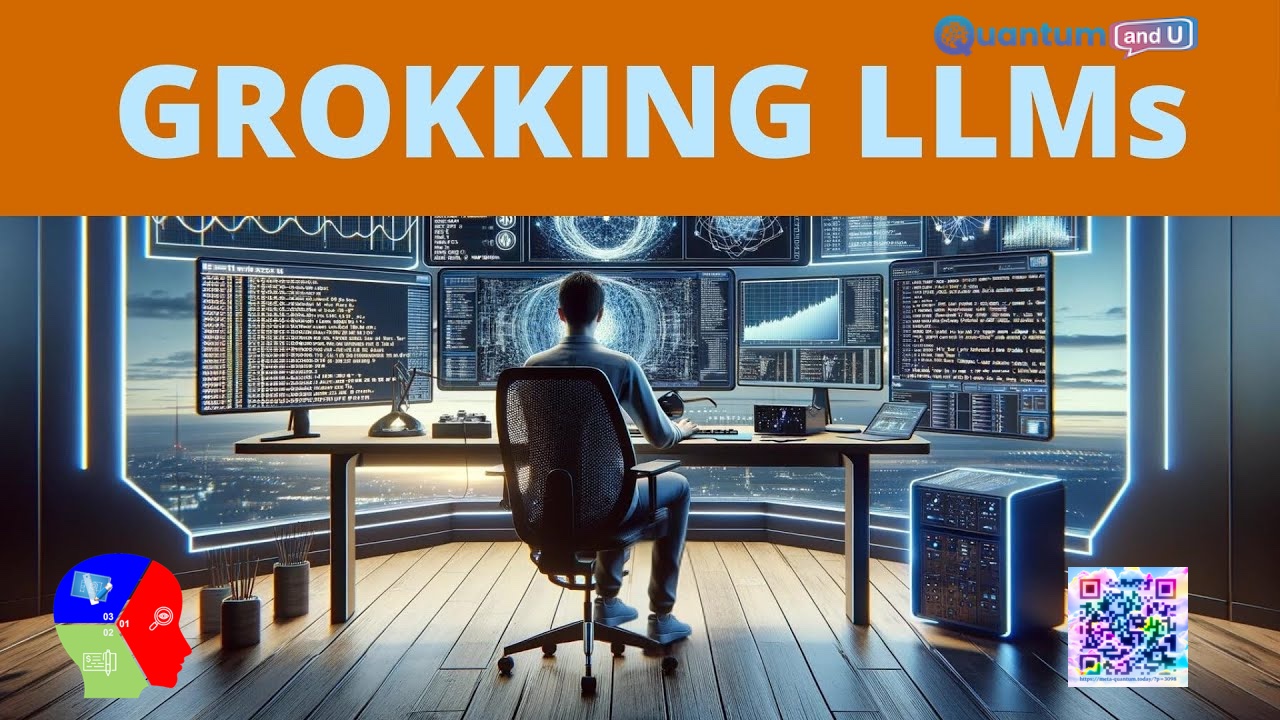
Introduction:
This section explores a groundbreaking discovery concerning Large Language Models (LLMs), specifically a phase transition known as “grokking.” This phase, observed in LLMs, marks a significant improvement in their understanding and generalization abilities, expanding the potential of these models. The presenter underscores the importance of this development and its impact on the future of artificial intelligence research.
Grokking: Learning Beyond Overfitting:
- In machine learning, there’s a challenge called overfitting. This occurs when a model memorizes the training data too well and fails to perform well on unseen data.
- Grokking is a recently discovered phenomenon that defies this intuition. It refers to a situation where a neural network on a small, algorithmic dataset (data generated by a computer program) can experience a sudden jump in generalization performance.
- This jump happens well after the model has apparently overfit the training data (its performance on the training data plateaus or even worsens).
- Essentially, the network seems to “grok” or develop a deep understanding of the underlying pattern in the data, allowing it to perform perfectly on unseen examples.
Implications for AI
- Grokking highlights the limitations of our current understanding of how neural networks learn. It suggests there might be more complex learning mechanisms at play than previously thought.
- Studying grokking can provide valuable insights for developing better training techniques for neural networks, especially when dealing with limited data.
- If we can understand how to induce grokking more reliably, it could lead to AI systems that learn more efficiently and generalize better on real-world tasks.
Grokking in Future of AI
The ability to learn from small datasets is crucial for the advancement of AI. Grokking research offers a promising avenue for:
- Overcoming data scarcity: Many real-world applications struggle with limited data. Grokking suggests ways to train effective AI models even with less data.
- Explainability and interpretability: Understanding how grokking works could shed light on how neural networks arrive at their decisions, making them more interpretable and trustworthy.
- Lifelong learning: Ideally, AI systems should continuously learn and improve. Grokking suggests mechanisms that could enable AI to learn from new data points throughout its lifetime.
Related Sections:
- Background and Motivation: The presenter recalls past discussions about the integration of various functionalities into LLMs and proposes the idea of a dedicated scientific or mathematical AI system. This sets the context for exploring the distinct phases in LLMs’ learning process.
- Definition of Grokking: The concept of grokking is introduced, emphasizing its role in extending the generalization capabilities of LLMs. The presenter draws parallels with prior research and outlines the phenomenon’s significance in understanding LLM behavior.
- Experimental Evidence: Through a series of experiments and analyses, the presenter demonstrates the occurrence of grokking in LLMs. Reference is made to research papers from prestigious institutions like MIT, providing empirical evidence of this phenomenon.
- Phase Transition and Mathematical Insights: The video dives into the intricate details of phase transitions observed in LLMs, shedding light on the underlying mathematical principles. Concepts such as Fourier transformation and geometric structures in embedded spaces are explored to elucidate the phenomenon.
- Implications and Applications: The presenter discusses the broader implications of grokking, particularly its relevance in enhancing complex reasoning abilities in LLMs. This section highlights the potential of grokking to revolutionize AI research and problem-solving approaches.
Potential Impact of Grokking on Southeast Asia and Opportunities:
Grokking’s ability to train AI models effectively with limited data holds significant promise for Southeast Asia, a region with:
- Data Scarcity: Many Southeast Asian countries have limited access to large, labeled datasets compared to developed nations. Grokking could enable the development of AI solutions even in data-poor environments.
- Diverse Applications: From agriculture and healthcare to finance and transportation, Southeast Asia has a wide range of sectors that can benefit from AI. Grokking could accelerate the development of AI-powered solutions for these sectors.
Here are some specific opportunities:
1. Agriculture:
- Precision Farming: Develop AI systems that analyze small datasets of soil conditions, weather patterns, and crop health to optimize fertilizer use and irrigation, improving yields with less data.
- Pest and Disease Detection: Train AI models on limited image data to identify pests and diseases in crops, allowing for early intervention and reduced crop losses.
2. Healthcare:
- Early Disease Diagnosis: Develop AI systems that analyze medical scans (X-rays, MRIs) from smaller patient pools to detect diseases like cancer or heart disease at earlier stages.
- Personalized Medicine: Train AI models on individual patient data (electronic health records) to personalize treatment plans and medication dosages.
3. Finance:
- Microfinance and Loan Risk Assessment: Develop AI models that assess creditworthiness for underbanked populations in Southeast Asia using limited financial data, promoting financial inclusion.
- Fraud Detection: Train AI models to identify fraudulent transactions with limited historical data, improving financial security.
4. Other Opportunities:
- Smart Cities: Develop AI systems to optimize traffic flow, energy consumption, and waste management in Southeast Asian cities using limited sensor data.
- Environmental Monitoring: Train AI models to analyze data from drones and satellites to monitor deforestation, pollution levels, and natural disasters even with limited coverage.
Challenges and Considerations:
- Explainability: While grokking allows for good performance, understanding how it works remains crucial for trusting AI decisions in sensitive areas.
- Data Bias: Limited data can perpetuate existing biases. Careful data collection and curation are essential to avoid biased AI systems.
- Infrastructure: Grokking research is still in its early stages. Implementing AI solutions across Southeast Asia will require robust IT infrastructure.
Conclusion:
This article concludes by highlighting the transformative potential of grokking in LLMs and its role in advancing artificial intelligence. Readers are encouraged to delve into the referenced research papers for more in-depth knowledge of this phenomenon and its implications.
In summary, despite data limitations, grokking offers a substantial opportunity for Southeast Asia to surpass traditional AI development by creating potent AI tools. By tackling the challenges and encouraging research in this field, Southeast Asia can place itself at the cutting edge of AI innovation.
Key Takeaway Points:
- Grokking represents a significant phase transition in LLMs’ learning process, enabling enhanced generalization capabilities.
- Experimental evidence from reputable institutions supports the existence and importance of grokking in LLMs.
- Mathematical insights, including Fourier transformation and geometric structures, provide a deeper understanding of grokking.
- Grokking has implications for improving complex reasoning abilities in LLMs, potentially reshaping AI research paradigms.
Related References:
- Grokking: Generalization Beyond Overfitting on Small Algorithmic Datasets
- MIT publication on phase states in transformers and their implications for generalization.
- Fourier Circuits in Neural Networks: Unlocking the Potential of LLMs in Mathematical Reasoning..
This review encapsulates the exploration of grokking in LLMs, offering viewers a comprehensive understanding of this groundbreaking discovery and its implications for the future of artificial intelligence.