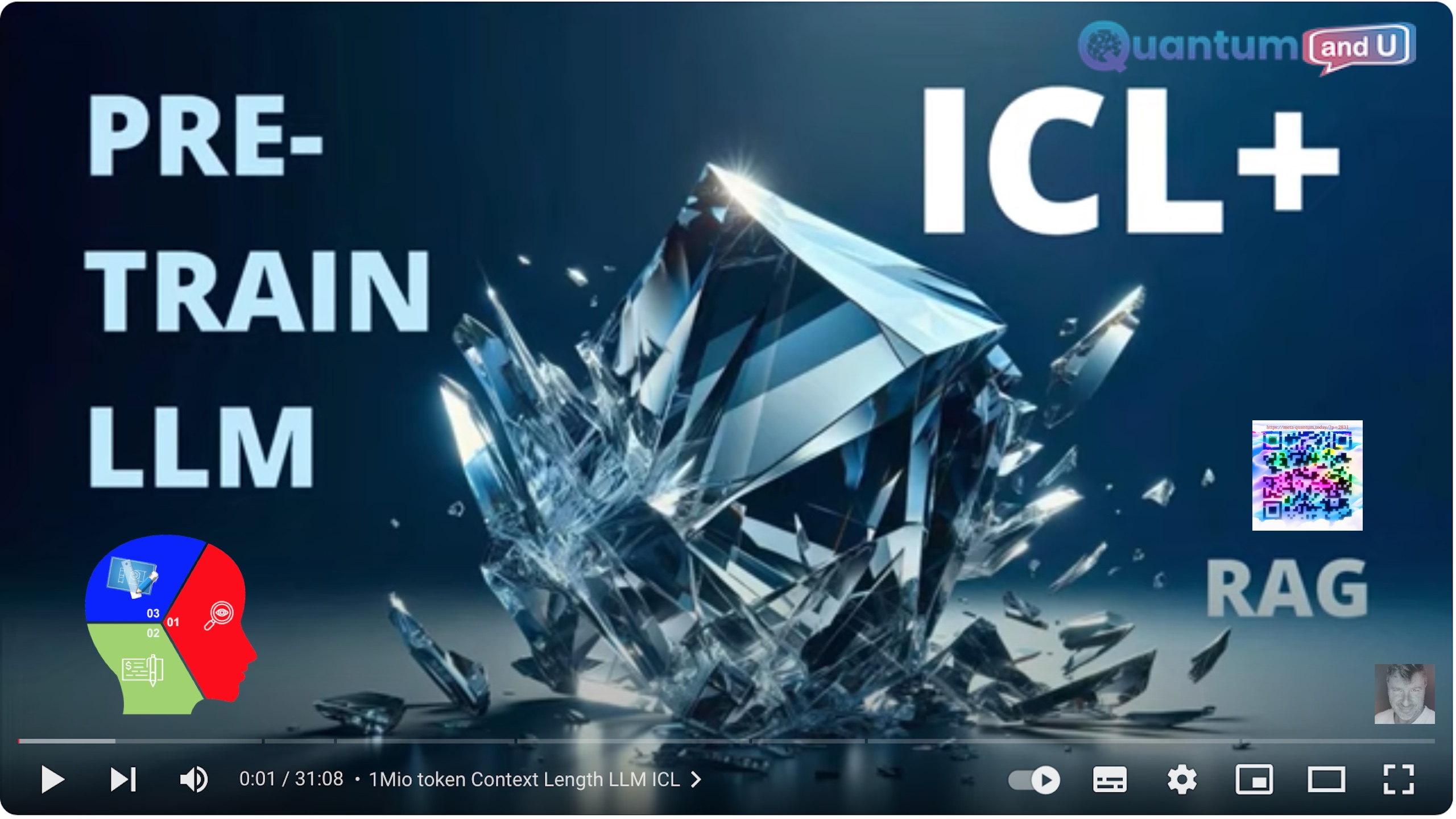
Introduction:
Today, we’re thrilled to introduce the revolutionary In Context Learning Plus (ICL+). This major advancement in Artificial Intelligence seeks to redefine our understanding and challenge the traditional limits of fine-tuning.
ICL+ extends beyond the conventional, breaking boundaries and pioneering a path where context lengths can expand up to an impressive one million tokens. We’re not just pushing the boundaries, but redefining them. This isn’t just a small step, but a massive leap in the world of AI learning.
So, let’s embark on this journey of exploration and discovery together. Let’s redefine what’s possible in this rapidly evolving field of AI learning, with ICL+ at the forefront. Join us as we step into the future, a future of endless possibilities.
About Fine-Tuning and ICL plus:
Fine-tuning and In-Context Learning (ICL) are both techniques used to improve the performance of large language models (LLMs) on specific tasks. Here’s a breakdown of each:
Fine-Tuning
- Involves training an existing LLM on a specific dataset of labeled examples.
- These examples typically involve instructions and their corresponding outputs.
- For instance, the task might be sentiment analysis, where the model is given a sentence and needs to label it as positive, negative, or neutral.
- Fine-tuning helps the LLM learn the patterns in the data and improve its performance on similar tasks.
ICL
- Stands for In-Context Learning.
- Involves providing the LLM with additional information or context during the query itself.
- This context can include instructions, previous examples, or desired outcomes.
- The LLM can then use this context to better understand the intent of the query and generate a more relevant response.
ICL vs Fine-Tuning
- Fine-tuning is a more general technique that can be applied to a wider range of tasks.
- ICL is a more targeted approach that is specifically designed to improve the performance of LLMs on specific queries.
- Research suggests that ICL can be particularly effective for smaller LLMs, while fine-tuning might be more beneficial for larger models.
ICL Plus:
- ICL Plus refers to a specific approach to ICL that leverages a combination of techniques, potentially including fine-tuning, to enhance the model’s performance.
- The idea is to leverage the strengths of both techniques: fine-tuning for initial task knowledge and ICL for adaptation based on context. pen_spark
Here are some additional points to consider:
- ICL prompts can include exemplars, which are specific examples of the desired outcome.
- Studies have shown that using exemplars in ICL prompts can significantly improve the performance of LLMs.
- The effectiveness of both fine-tuning and ICL depends on the quality and quantity of the data used.
I hope this explanation clarifies the concepts of Fine-Tuning and ICL! If you’d like to delve deeper into any specific aspect, feel free to ask.
Video about ICL:
Sections in the Video:
- Current Landscape:
- Traditional methods like fine-tuning and DPO alignment have been the norm, but they come with limitations, especially with proprietary models.
- Introduction of ICL+ with its expansive context lengths, offering a new approach to learning without extensive cloud compute hours.
- Expanding Context Lengths:
- Discussion on the implications of context length on tasks like summarization, highlighting the constraints of shorter lengths.
- Exploration of advancements in context length, such as Google GPT-1.5 Pro’s one million token capacity, and Microsoft’s push towards 32,000 token lengths.
- Divergent Perspectives:
- Examination of contrasting research perspectives on methods like Rag, questioning its impact on LLMs and potential for inducing hallucinations.
- Insights into ongoing studies in domains like finance and medicine, hinting at the complexity of integrating new data structures.
- Empowering ICL+:
- Transition from few-shot to many-shot examples in ICL+, leveraging the expanded context length to enhance learning.
- Illustration of significant performance improvements, even surpassing fine-tuning, through the provision of extensive examples.
- Understanding Task Dependency:
- Analysis of performance variance across different tasks and domains, emphasizing the importance of tailored approaches.
- Exploration of learning curves and saturation effects, shedding light on optimal example thresholds for tasks like translation and code verification.
- Reinforced vs. Unsupervised ICL:
- Differentiation between reinforced and unsupervised ICL approaches, highlighting the autonomy gained through the latter.
- Evaluation of performance metrics and nuances between the two methods, showcasing instances where unsupervised ICL excels.
Potential Impact of ICL Plus on Southeast Asia and Business Opportunities:
ICL Plus, with its ability to leverage context and adapt to specific situations, has the potential to significantly impact Southeast Asia and create new business opportunities. Here’s a breakdown of the potential effects:
Positive Impacts:
- Improved Language Processing: ICL Plus can enhance the performance of LLMs in understanding Southeast Asian languages, which often have unique dialects and nuances. This could lead to more accurate applications like machine translation, chatbots, and voice assistants tailored for the region.
- Localized Solutions: Businesses can leverage ICL Plus to develop custom solutions for Southeast Asian markets. Imagine chatbots trained on local customer service inquiries or virtual assistants understanding regional business practices.
- Personalized Marketing: ICL Plus can personalize marketing campaigns by analyzing customer data and context. Businesses can target specific demographics with culturally relevant messaging, leading to increased engagement and sales.
- Education and Research: ICL Plus can power intelligent tutoring systems that adapt to individual student learning styles. It can also aid researchers by analyzing vast amounts of data in local languages, accelerating scientific progress.
Business Opportunities:
- ICL Plus Development and Training: Companies can specialize in developing and training ICL Plus models for Southeast Asian languages. This could involve creating training data sets and customizing models for specific industries.
- Building ICL Plus Applications: Businesses can develop applications that leverage ICL Plus capabilities. This could include chatbots for customer service, virtual assistants for businesses, or marketing automation tools.
- Data Analysis and Consulting: Consulting firms can offer services to help businesses leverage ICL Plus for data analysis and insights generation. This could be particularly valuable for understanding customer behavior and market trends.
Challenges to Consider:
- Data Availability: Training ICL Plus models requires large amounts of data in Southeast Asian languages. Data collection and anonymization could pose challenges.
- Technical Expertise: Implementing ICL Plus requires expertise in machine learning and NLP. Businesses might need to invest in training or hire specialists.
- Ethical Considerations: As with any AI technology, ethical considerations around bias and data privacy need to be addressed when deploying ICL Plus solutions.
Conclusion:
The introduction of ICL+ signifies a major shift in AI learning, offering flexibility, efficiency, and autonomy. It enables us to push large language models (LLMs) to unprecedented performance levels without traditional fine-tuning by using extensive context lengths and diverse learning methods. However, further exploration and understanding of task dependencies and methodological approaches are necessary.
In summary, ICL+ holds significant potential to enhance language processing and open up new business opportunities in Southeast Asia. However, successful implementation of this technology calls for addressing issues related to data availability, technical expertise, and ethical considerations.
Key Takeaways:
- ICL+ offers a breakthrough in AI learning, eliminating the need for extensive fine-tuning.
- Expanding context lengths enable more comprehensive learning and task performance improvements.
- Task-specific approaches and understanding learning curves are crucial for optimizing ICL+ effectiveness.
- Unsupervised ICL shows promise in fostering autonomy and reducing reliance on labeled data.
References: