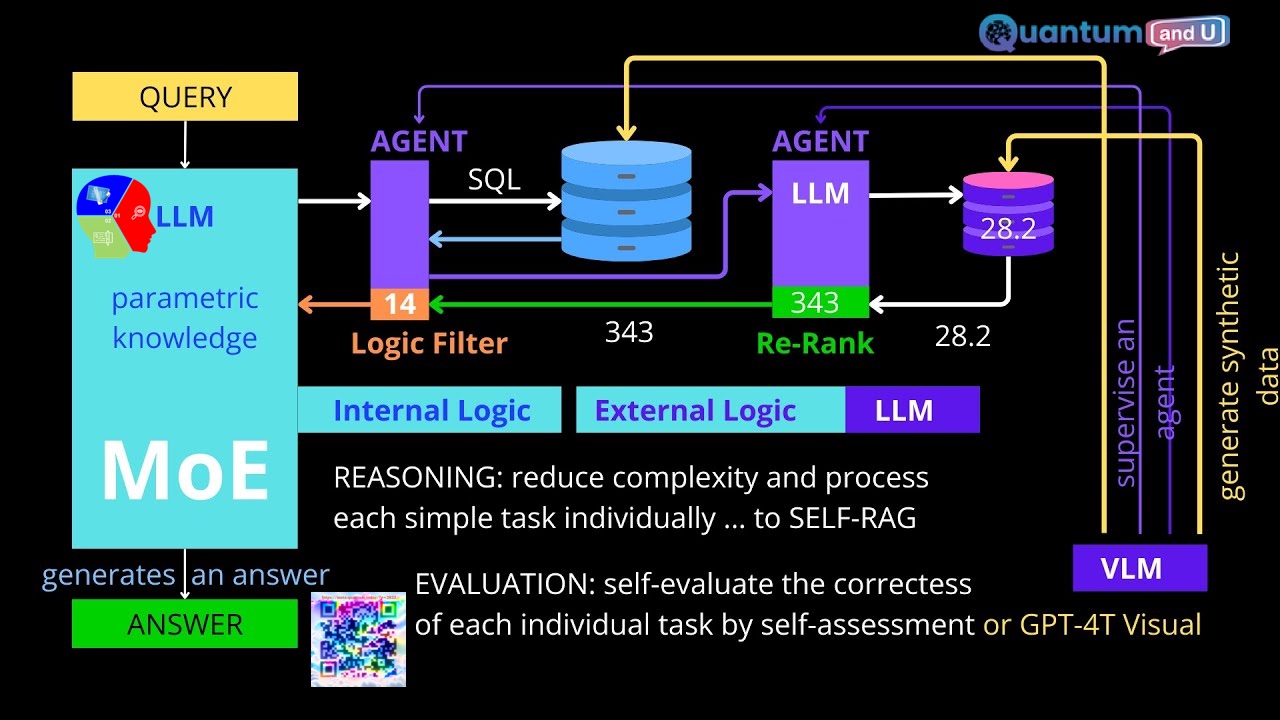
Introduction:
Welcome! As we journey into the evolving world of artificial intelligence, our focus today is the groundbreaking Retrieval Augmented Generation (RAG). This transformative technology is reshaping our understanding of AI’s potential. This document aims to demystify RAG and its profound implications for AI’s future. Dive with us into RAG’s technical aspects and its role in bolstering AI capabilities. Let’s explore how RAG is driving the AI revolution.
AI – Superhuman RAG:
“Superhuman RAG” sounds like an enthusiastic way to describe a Retrieval-Augmented Generation (RAG) system. RAG is a technique that combines large language models (LLMs) with external knowledge retrieval to create more informative and accurate outputs.
RAG Explained:
- Retrieval: When you give RAG a prompt or question, it searches through a vast amount of text data (like articles, code, or books) to find relevant pieces of information.
- Augmentation: RAG takes the retrieved information and uses it to inform the LLM’s generation process. This helps the LLM to understand the context of the prompt and generate a more relevant and accurate response.
- Generation: Finally, the LLM uses its knowledge and the retrieved information to create a response, like answering your question or writing a creative text format.
Superhuman Aspects:
- Enhanced Accuracy: By incorporating external knowledge, RAG can potentially provide more accurate and reliable information compared to LLMs on their own.
- Improved Context Understanding: RAG helps the LLM grasp the context of a prompt, leading to more focused and relevant responses.
- Greater Knowledge Base: By leveraging external data, RAG can access a broader range of information than an LLM’s internal knowledge base alone.
Is it Really Superhuman?
While RAG is a powerful technique, it still has limitations. The quality of the retrieved information and the effectiveness of the LLM play a big role. Additionally, RAG systems require significant computing power and data to function effectively.
Video about AI – Superhuman RAG:
Related Sections of the above video:
- Understanding RAG Technology:
- RAG operates on a foundation of a Large Language Model (LLM) which synthesizes vast amounts of data to generate responses.
- Through pre-training and fine-tuning, the LLM learns to retrieve information from databases or the internet to enhance its responses.
- The process involves complex computations within high-dimensional vector spaces, necessitating techniques like dimensionality reduction for efficiency.
- Complexity Simplification and Quantization:
- Despite the complexity, efforts are made to simplify computations through techniques like quantization, reducing precision for faster processing.
- Ongoing research explores further simplifications while maintaining accuracy, such as 1.58-bit representation.
- Agent Systems and Multi-Agent Interactions:
- Agents, ranging from simple rule-based to sophisticated AI models, play crucial roles in the RAG framework.
- Multi-agent systems enable collaboration and communication among agents to enhance problem-solving capabilities.
- Specialization within agent groups ensures expertise in various domains, facilitating coherent reasoning and evaluation.
- Challenges and Future Directions:
- Concerns arise regarding the reliability and factuality of synthesized information, prompting research into fact-checking methodologies.
- Ethical considerations regarding the use of superhuman AI and its implications on decision-making processes.
- The need for continuous refinement and development to address inherent complexities and limitations in current AI systems.
Impact of Superhuman RAG on SEA:
The arrival of a superhuman RAG system could have a significant impact on Southeast Asia, bringing both challenges and exciting opportunities. Let’s explore some potential effects:
Positive Impacts:
- Boosting Research and Development: RAG could accelerate scientific progress by enabling researchers to access and analyze massive datasets more efficiently. This could lead to breakthroughs in fields like medicine, agriculture, and disaster management.
- Enhanced Education and Learning: RAG-powered educational tools could personalize learning experiences and provide students with more relevant and in-depth information.
- Improving Infrastructure Development: RAG could help with complex infrastructure projects by analyzing vast amounts of data on geography, resources, and environmental factors. This could lead to more efficient and sustainable infrastructure development.
- Empowering Local Businesses: RAG could empower local businesses by providing them with better market insights, competitor analysis, and translation services.
Challenges:
- Digital Divide: Unequal access to technology could exacerbate the digital divide, leaving some communities behind. Governments and NGOs would need to invest in bridging this gap.
- Job displacement: Automation powered by RAG could lead to job losses in some sectors. Governments and educational institutions would need to focus on retraining and reskilling the workforce.
- Ethical Considerations: Bias in the training data could lead to biased outputs from RAG systems. Careful attention needs to be paid to ensuring ethical development and deployment of this technology.
- Data Privacy: The vast amount of data needed for RAG systems raises concerns about data privacy. Regulations and best practices for data collection and use would be crucial.
Opportunities:
- Developing a Skilled Workforce: Southeast Asia can focus on developing a skilled workforce that can work alongside and manage RAG systems. This could create new jobs in areas like data analysis, system development, and ethical oversight.
- Promoting Innovation: The region can foster a culture of innovation by encouraging the development of applications and services built on top of RAG technology.
- Enhancing Regional Collaboration: Southeast Asian countries could collaborate on developing and deploying RAG systems for regional challenges like climate change and disaster preparedness.
Conclusion:
RAG technology represents the effort to utilize AI for superhuman capabilities. Despite its promising advancements, challenges such as reliability, factuality, and ethical use persist. Navigating this landscape requires a thorough understanding of AI’s foundational principles and a forward-thinking approach.
Overall, RAG shows promise in advancing the abilities of Language Models, bringing them closer to human-level understanding and generation. Although it’s not quite superhuman yet, it signifies a significant step forward.
The influence of superhuman RAG on Southeast Asia will hinge on the development and application of the technology. By actively addressing challenges and capitalizing on opportunities, Southeast Asia can exploit RAG to become a more prosperous and innovative region.
Key Takeaway Points:
- RAG technology leverages LLMs to synthesize vast amounts of data and enhance responses through retrieval and augmentation processes.
- Techniques like quantization and multi-agent systems contribute to computational efficiency and problem-solving capabilities.
- Ongoing research focuses on fact-checking methodologies and ethical considerations to address challenges and ensure responsible AI development.
Related References:
- Google Deep Mind’s research on factuality verification.
- GitHub repositories for exploring RAG and multi-agent systems.
- Articles discussing the economic and ethical implications of superhuman AI.
Thank you for joining us on this exploration of RAG technology. Stay tuned for more insights into the evolving landscape of artificial intelligence!