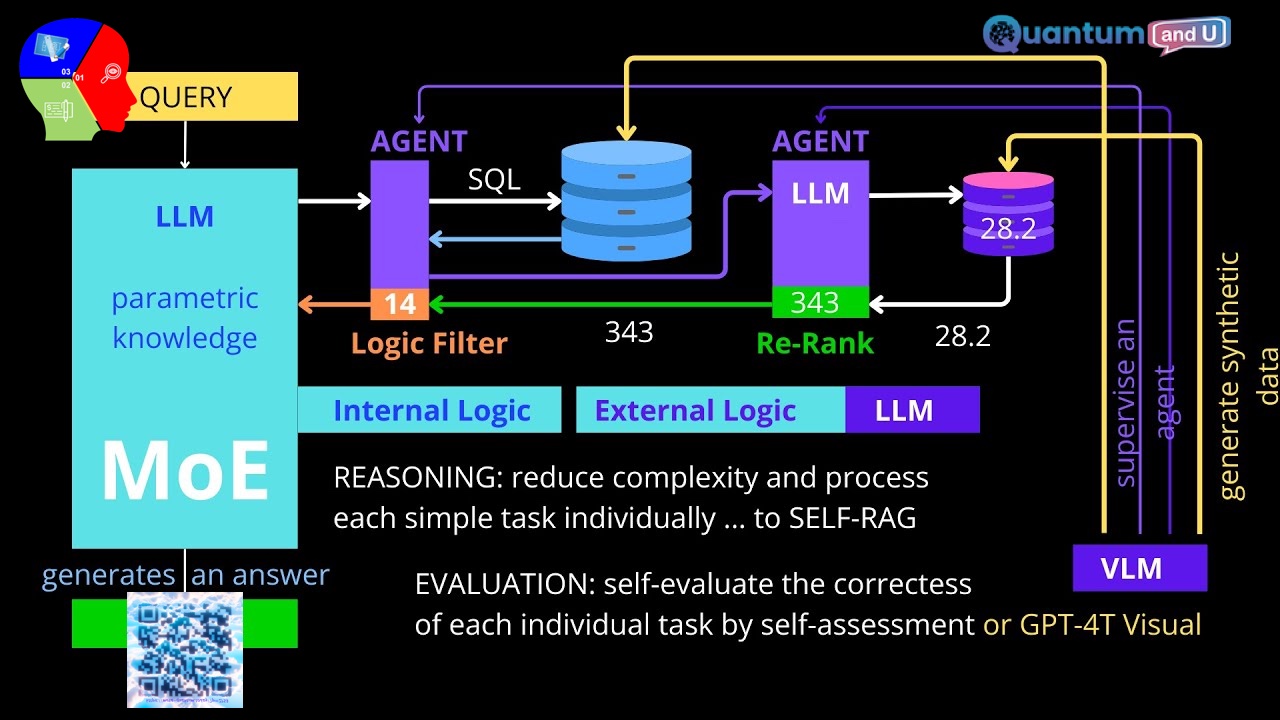
Introduction:
This exploration seeks to understand the buzz around the concept of superhuman capabilities in artificial intelligence, focusing primarily on a system known as RAG (Retrieval-Augmented Generation).
We delve into the complexities and components of the RAG system, aiming to provide a comprehensive understanding of its mechanisms and potential. Our goal is to comprehend the far-reaching implications this system could have on the future of artificial intelligence.
We cover everything from its fundamental principles to its advanced functionalities, aiming to illuminate the various aspects of the RAG system. This will provide a complete understanding of this innovative technology, which promises to expand the boundaries of AI’s superhuman capabilities.
Superhuman Retrieval Augmented Generation (RAG) with AI LLMs:
The key concept:
- LLM: Large Language Model, which is a type of AI system trained on massive amounts of text data to communicate and generate human-like text in response to a wide range of prompts and questions. You can think of it as a powerful language understanding and generation engine.
- Retrieval Augmented Generation (RAG): This is a technique that enhances the capabilities of an LLM by allowing it to access and retrieve information from external sources, like the internet, in addition to its internal knowledge base.
How it works:
- User Input: You ask the LLM a question.
- Retrieval: The LLM first consults its internal knowledge base and potentially retrieves relevant information from external sources.
- Augmentation: The retrieved information is then processed and combined with the LLM’s own knowledge.
- Generation: Finally, the LLM uses this augmented knowledge to generate a response to your question.
Benefits of Superhuman RAG:
- More informative responses: By incorporating external information, RAG allows LLMs to provide more comprehensive and informative answers.
- Improved accuracy: Access to factual data from the real world can help LLMs avoid making factual errors.
- Versatility: RAG enables LLMs to tackle a wider range of questions and tasks.
Things to Consider:
- Complexity: Building and implementing a Superhuman RAG system requires advanced techniques and significant computational resources.
- Data Quality: The accuracy and usefulness of the retrieved information heavily depend on the quality and reliability of the external sources.
Video of the Superhuman RAG with LLM:
Related Sections:
- Overview of RAG:
- RAG comprises a large language model (LLM) that integrates retrieval, augmentation, and generation processes.
- It involves pre-training with neural network tensor weights, fine-tuning for specific domains, and alignment for friendly behavior.
- When encountering gaps in data, RAG retrieves information from databases or the internet to augment its knowledge.
- Complexities in Retrieval, Augmentation, and Generation:
- Retrieval involves encoding vast knowledge into neural network and mathematical vector spaces.
- Augmentation integrates retrieved data with LLM’s parametric knowledge, enhancing the generation process.
- High-dimensional embeddings are essential but require dimensionality reduction for efficient mathematical operations.
- Quantization techniques aim to simplify mathematical calculations while preserving accuracy.
- Multi-Agent Systems:
- Agents range from simple rule-based to sophisticated, internet-connected entities.
- In a multi-agent scenario, agents collaborate to retrieve, filter, and augment information before generating responses.
- Challenges arise in ensuring logical coherence and accuracy, requiring reasoning capabilities and external evaluations.
- Implications and Challenges:
- Superhuman claims in AI raise ethical and practical concerns, as AI models may synthesize data without discernment.
- Long-form factuality studies aim to verify the accuracy of AI-generated content.
- Economic implications highlight the need for reliable AI models and the ongoing research in addressing complex problems.
Impact of Superhuman RAG on Southeast Asia Business Development: Opportunities and Challenges
Superhuman Retrieval Augmented Generation (RAG) has the potential to significantly impact business development in Southeast Asia, offering both exciting opportunities and challenges.
Opportunities:
- Enhanced Market Research: Businesses can leverage RAG-powered LLMs to gather in-depth information on Southeast Asian markets. This can include competitor analysis, consumer trends, and regulatory landscapes across different countries.
- Targeted Marketing and Sales: LLMs with access to real-time data can personalize marketing campaigns and sales strategies for specific Southeast Asian demographics.
- Improved Customer Service: RAG-based chatbots can provide multilingual customer support across Southeast Asia, catering to diverse customer needs and languages.
- Innovation and New Product Development: LLMs can analyze vast amounts of data to identify new business opportunities, product ideas, and market gaps specific to Southeast Asia.
- Streamlined Operations: RAG can automate tasks like data analysis, report generation, and competitor monitoring, freeing up human resources to focus on strategic initiatives.
Challenges:
- Data Availability and Quality: The effectiveness of RAG hinges on the quality and accessibility of data sources. Limited or unreliable data in certain Southeast Asian markets could hinder the accuracy of LLM outputs.
- Language Barriers: Southeast Asia is a linguistically diverse region. RAG systems need to be able to handle multiple languages effectively to be truly useful for businesses.
- Cultural Nuances: LLMs trained on Western data may struggle to understand cultural subtleties in Southeast Asian business practices. Businesses need to be mindful of potential biases and ensure the LLM is trained on culturally appropriate data.
- Technical Expertise: Implementing and maintaining RAG systems requires technical expertise, which might be a barrier for smaller businesses in Southeast Asia.
Conclusion:
The idea of superhuman AI, as demonstrated by systems like RAG, represents the continuous effort to utilize AI for intricate tasks. Despite the current strategies involving the simplification of complexity into manageable segments, it’s understood that this may not be the final solution. Ongoing research and ethical considerations play a crucial role in dealing with the intricacies of AI advancement.
In summary, Superhuman RAG offers a potent tool for business development in Southeast Asia. By thoughtfully addressing the challenges and ensuring responsible execution, businesses can use RAG to secure a competitive advantage and unveil new growth prospects in the region.
Key Takeaway Points:
- RAG integrates retrieval, augmentation, and generation processes in AI.
- Multi-agent systems enhance AI capabilities but pose challenges in ensuring logical coherence.
- Ethical considerations and ongoing research are crucial in harnessing AI’s potential responsibly.