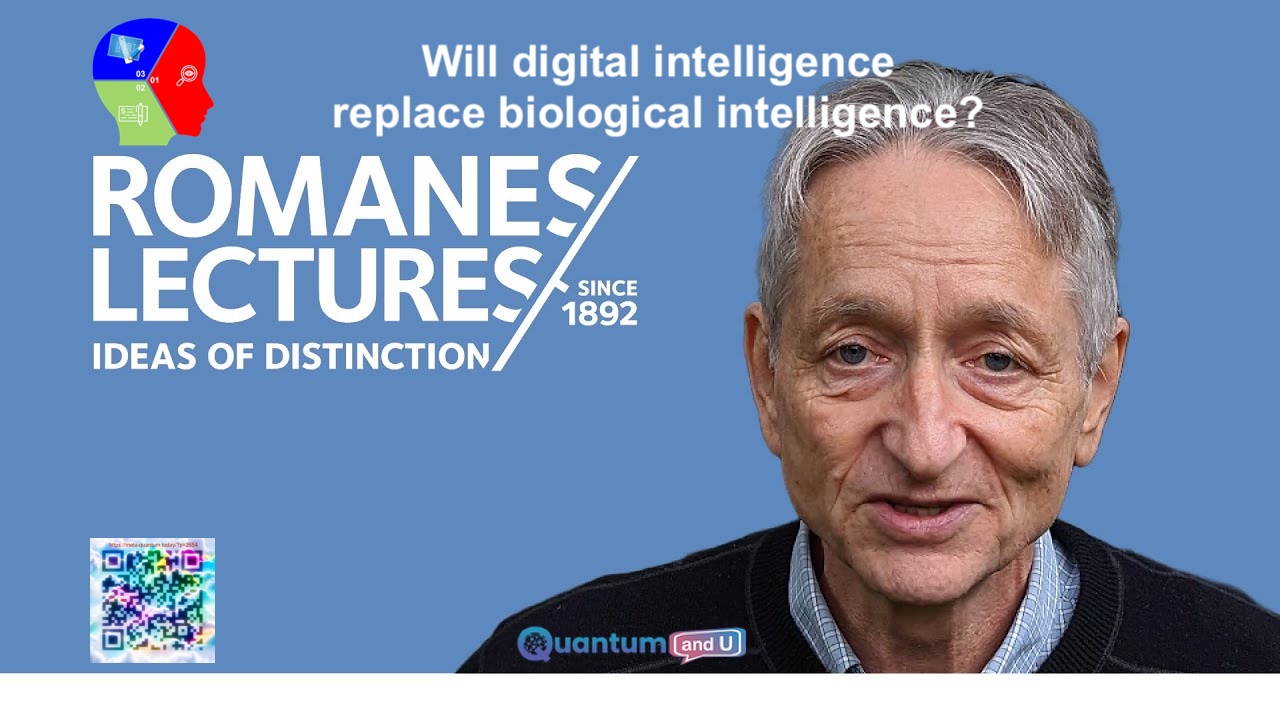
Introduction:
In the Romanes Lecture, Professor Geoffrey Hinton delves into the field of artificial intelligence. He explores neural networks, language models, and the changing landscape of digital intelligence. Hinton presents different paradigms of past intelligence, leading into a comprehensive explanation of artificial neural networks and their learning abilities. He provides insights into the evolution of AI, its challenges, and the possible risks posed by powerful AI systems throughout the lecture.
Digital intelligence (DI) and biological intelligence (BI) :
Digital intelligence (DI) and biological intelligence (BI) are two fundamentally different forms of intelligence that have emerged through distinct evolutionary paths.
Digital intelligence refers to the intelligence exhibited by machines, particularly those programmed with artificial intelligence (AI). AI systems are designed to process information and respond to stimuli in a way that simulates human intelligence, but they do so through entirely different mechanisms than biological brains. DI is based on complex algorithms and vast amounts of data, while BI is rooted in the physical structure and function of the brain.
Biological intelligence, on the other hand, is the intelligence displayed by living organisms, including humans, animals, and even plants to some extent. BI is the product of millions of years of evolution and is mediated by the complex network of neurons and synapses that make up the brain. BI allows organisms to learn, adapt, solve problems, and interact with their environment in a way that is still not fully understood by scientists.
Here’s a table summarizing the key differences between digital and biological intelligence:
Feature | Digital Intelligence (DI) | Biological Intelligence (BI) |
---|---|---|
Substrate | Silicon chips, electronic circuits | Neurons, synapses |
Processing | Based on algorithms and data manipulation | Emerges from the physical structure and function of the brain |
Learning | Through training on large datasets | Through experience and interaction with the environment |
Adaptability | Can be easily modified and updated | Limited by the physical constraints of the brain |
Goals | Defined by the programmers | Emerge from the needs and desires of the organism |
Consciousness | Not currently considered a feature of DI | A defining characteristic of some forms of BI (e.g., humans) |
The relationship between DI and BI is a complex and evolving one. As AI technology continues to advance, it is becoming increasingly sophisticated and capable of performing tasks that were once thought to be the exclusive domain of human intelligence. However, BI remains fundamentally different from DI in its origins, mechanisms, and capabilities.exclamation The future of both DI and BI is uncertain, but it is clear that they will continue to shape the world in profound ways.
Video : Will digital intelligence replace biological intelligence?
Related Sections of the video:
- Introduction to Neural Networks: Hinton introduces neural networks as models inspired by the biological brain’s ability to learn and reason. He contrasts the logic-inspired approach with the biologically inspired approach, emphasizing the importance of learning in intelligence.
- Artificial Neural Networks: Explaining the structure of artificial neural networks, Hinton discusses their layers of neurons and their ability to learn features relevant to tasks like image recognition.
- Training Neural Networks: Hinton delves into the process of training neural networks, discussing methods like mutation and how neural networks learn to represent complex data.
- Language Models: He explores the development of language models, debunking notions that language isn’t learned and highlighting breakthroughs in training neural networks to understand syntax and semantics.
- Risks of AI: Hinton discusses various risks associated with powerful AI systems, including fake media, job loss, surveillance, and the existential threat of superintelligent AI systems.
- Analogue Computation: The lecture concludes with Hinton’s exploration of analogue computation, suggesting it as a potential avenue for energy-efficient, highly capable AI systems.
Impact of DI and BI on SEA: Opportunities and Challenges
DI (Digital Intelligence):
Impact:
- Economic growth: DI can drive economic growth in Southeast Asia by:
- Automating tasks: Increasing efficiency and productivity in various sectors like manufacturing, agriculture, and logistics.
- Creating new industries: Fostering the development of new industries like AI-powered services and robotics.
- Boosting trade: Facilitating smoother international trade through enhanced logistics and supply chain management.
- Improved public services: DI can enhance the delivery of public services in areas like:
- Education: Utilizing AI for personalized learning and educational support.
- Healthcare: Applying AI for disease diagnosis, drug discovery, and telehealth services.
- Governance: Streamlining administrative processes and improving transparency through data analysis.
- Social development: DI can contribute to social development by:
- Bridging the digital divide: Providing access to information and communication technologies for marginalized communities.
- Empowering individuals: Offering tools for education, skill development, and entrepreneurship.
Challenges:
- Job displacement: Automation through DI can lead to job losses in certain sectors, requiring workforce reskilling and adaptation.
- Ethical considerations: Issues like data privacy, bias in algorithms, and responsible development of AI need careful consideration.
- Digital divide: Unequal access to technology and digital literacy can exacerbate existing social inequalities.
Opportunities:
- Investment in AI infrastructure: Governments and private companies can invest in developing AI infrastructure, research, and talent development.
- Focus on human-centered AI: Ensuring that AI development prioritizes human values, ethics, and collaboration with humans.
- Building digital literacy: Implementing programs to equip individuals with the skills needed to thrive in a digitalized world.
BI (Biological Intelligence):
Impact:
Biotechnological advancements: Advancements in bioengineering and genomics can lead to:
- Improved healthcare: Development of personalized medicine, new treatments for diseases, and improved diagnostics.
- Sustainable agriculture: Development of pest-resistant crops, improved crop yields, and more efficient food production.
- Environmental conservation: Utilizing biotechnologies for bioremediation, waste management, and sustainable resource utilization.
Challenges:
- Ethical concerns: Ethical considerations regarding genetic engineering, human enhancement, and potential environmental risks need careful evaluation.
- Accessibility and affordability: Ensuring equitable access to the benefits of biotechnology for all members of society.
- Regulation and governance: Implementing robust regulations to ensure the responsible and safe development and application of biotechnologies.
Opportunities:
- Investment in research and development: Supporting research and development in life sciences and biotechnology to address regional challenges.
- Promoting public awareness and education: Fostering public understanding of the potential and limitations of biotechnologies.
- Developing regulatory frameworks: Establishing clear and comprehensive regulatory frameworks for the development and application of biotechnologies.
Conclusion:
To conclude the lecture, Professor Hinton shares his conviction that digital intelligence could exceed biological intelligence. This is particularly true as advances in analogue computation provide energy-efficient solutions. He underscores the importance of considering the risks of powerful AI systems and the necessity of ethical AI development.
Southeast Asia could achieve considerable economic growth, enhance public services, and address pressing societal issues by leveraging the potential of both digital and biological intelligence. However, navigating the challenges these technologies pose requires thoughtful planning, ethical considerations, and investment in infrastructure and human resources.
Takeaway Key Points:
- Neural networks emulate the learning capabilities of the human brain, evolving from a logic-inspired to a biologically inspired approach.
- Training neural networks involves optimizing weights to improve performance, with breakthroughs in language models demonstrating their ability to understand syntax and semantics.
- Risks associated with AI include fake media, job displacement, surveillance, and the existential threat of superintelligent AI systems.
- Analogue computation presents a potential path towards energy-efficient AI, but ethical considerations are crucial in AI development.