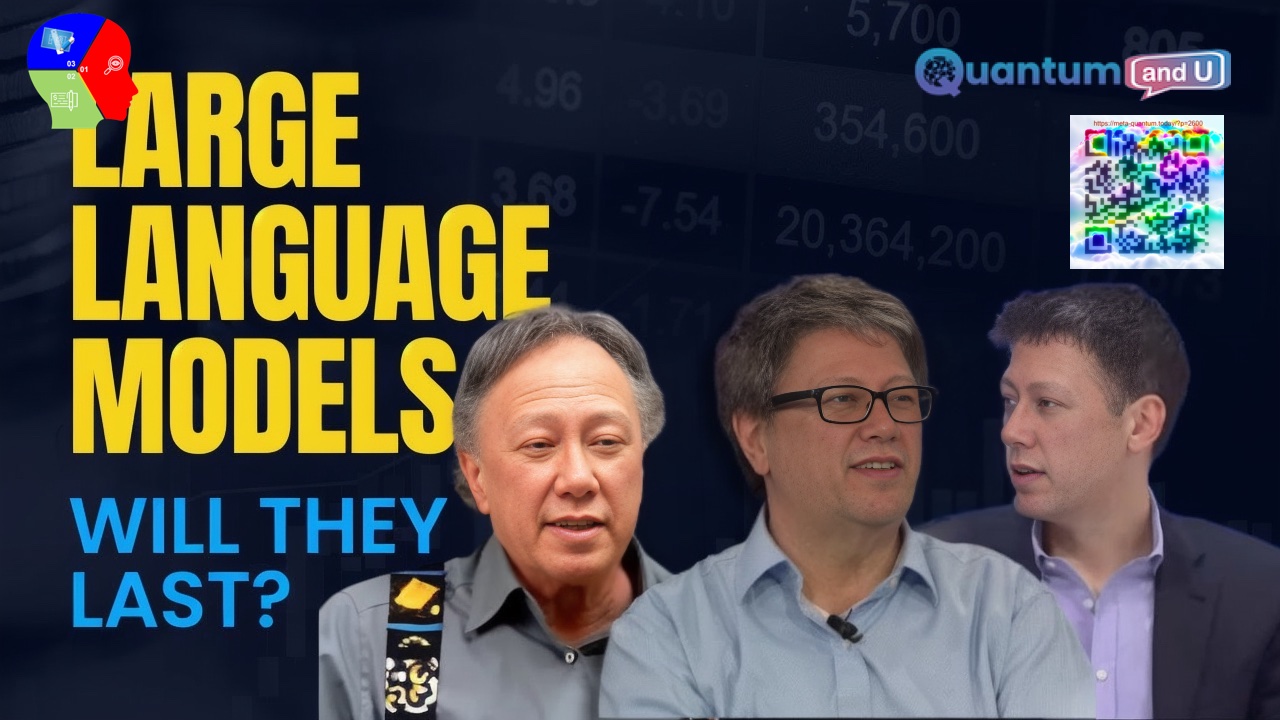
Introduction:
This article provides a detailed examination of large language models (LLMs) and their evolving role in the rapidly changing landscape of artificial intelligence (AI). This perspective is shared by prominent industry figures such as Yann LeCun from Meta, futurist Ray Kurzweil, Jack Hidary from Sandbox AQ, and several academics who have recently participated in international forums with AI as a central topic. We’ve consolidated these various viewpoints to offer a holistic view on the subject. This piece explores the insights of these experts, who believe that while LLMs are a significant breakthrough in the current AI environment, they may not remain at the forefront of AI progress in the future. This implies that there are more groundbreaking AI innovations to anticipate.
LLMs Beyond Characters, Towards Meaning:
Large Language Models (LLMs) are just one facet of the ever-evolving landscape of artificial intelligence (AI). It’s important to recognize the diversity present in both the types of AI models and their functionalities.
Beyond LLMs: While LLMs excel at processing and generating text, other AI models specialize in different domains:
- Computer vision models: These models analyze and interpret visual data, enabling applications like image recognition, object detection, and autonomous vehicles.
- Robotics: This field integrates various AI models with physical robots, allowing them to interact with the environment, learn, and adapt.
- Reinforcement learning models: These models learn through trial and error, making them adept at games, simulations, and dynamic decision-making tasks.
Exploring the future: The future of AI models holds immense potential for growth and diversification:
- Multimodal models: These models integrate different AI capabilities, like combining language understanding with visual processing, for more comprehensive and human-like interactions.
- Explainable AI: This area focuses on making AI models transparent and understandable, allowing humans to better grasp their decision-making processes.
- Neurosymbolic AI: This combines symbolic reasoning with neural network learning, aiming to create AI systems capable of logic, reasoning, and common-sense understanding.
Beyond characters, meaning, and reasoning: While understanding characters, words, and reasoning are crucial aspects of human intelligence, AI research delves into other areas as well:
- Philosophy: AI researchers are exploring how to imbue ethical considerations, values, and philosophical principles into AI models to promote responsible and beneficial applications.
- Creativity: Research is ongoing in developing AI models that can generate creative content like art, music, and literature, pushing the boundaries of human-machine collaboration.
The future of AI promises exciting advancements in diverse areas, moving beyond LLMs and towards a more comprehensive and multifaceted understanding of human intelligence and its potential applications.
Video about LLMs and Future AI Models:
Related Sections about the Video:
- Skepticism Towards LLMs:
- Experts like Yann LeCun and Sam Altman express skepticism, viewing LLMs as transitional tools rather than the ultimate solution in AI.
- Concerns are raised about the limitations of LLMs, including their inability to comprehend causation, reason, or interact with the physical world effectively.
- Limitations of LLMs:
- LLMs excel in processing vast amounts of text data but face challenges in domains where data is scarce, such as material or molecule creation.
- They lack physical intuition and understanding of causation, hindering their ability to perform tasks requiring real-world interaction, like autonomous driving or household chores.
- Evolutionary Needs:
- Calls for a paradigm shift in AI development away from reliance solely on LLMs towards more advanced approaches.
- Emphasis on the necessity of machines understanding causation, which requires the ability to conduct experiments and interact with the physical world.
- Proposed Solutions:
- Suggestions for making LLMs smarter include mimicking how infants learn about the world through observation and interaction.
- Proposals for training LLMs using video data to enhance their understanding of real-world dynamics, highlighting ongoing research efforts in this direction.
Understanding the Future AI Models and opportunities:
Understanding the future of AI models and opportunities is crucial for several reasons:
Preparation and Adaptation:
- Individuals: By understanding the potential impact of AI on various sectors, individuals can prepare for potential career changes, upskill themselves, and adapt to the evolving job market.
- Businesses: Businesses can leverage AI to improve efficiency, gain a competitive edge, and develop innovative products and services aligned with future needs.
- Governments: By anticipating the opportunities and challenges posed by AI, governments can develop policies and regulations to ensure responsible development, address ethical concerns, and promote responsible use of AI for societal benefit.
Informed decision making:
- Individuals: Understanding the capabilities and limitations of AI models allows individuals to make informed decisions about interacting with them, trusting their outputs, and avoiding potential biases.
- Businesses: Businesses can make informed decisions about investing in AI technologies, knowing the potential benefits, costs, and ethical considerations involved.
- Governments: Governments can develop informed policies and regulations regarding AI development, deployment, and use based on a clear understanding of its potential impact.
Shaping the future:
- By engaging with discussions about the future of AI, individuals and organizations can actively contribute to shaping its development in a responsible and ethical manner that aligns with societal values and promotes human well-being.
- Understanding the opportunities can help individuals and organizations contribute to the development and application of AI in ways that address real-world challenges, solve problems, and improve lives.
Conclusion:
The review recognizes the current commercial value of text-based LLMs despite their limitations. However, it emphasizes the need for continued innovation and exploration of alternatives to LLMs to achieve true artificial general intelligence (AGI).
In general, understanding the future of AI models and their opportunities enables individuals, businesses, and governments to adapt to the changes AI brings, make informed decisions, and help shape a positive future for everyone.
Key Takeaway Points:
- LLMs are groundbreaking but may not represent the future pinnacle of AI.
- Concerns exist regarding the limitations of LLMs in understanding causation and interacting with the physical world.
- Calls for a shift towards AI approaches that prioritize real-world understanding and causation.
- Ongoing research explores methods to enhance LLMs’ capabilities through video data and mimicking infant learning.
- Despite limitations, current LLMs offer significant commercial value, but future advancements are necessary for true AGI.