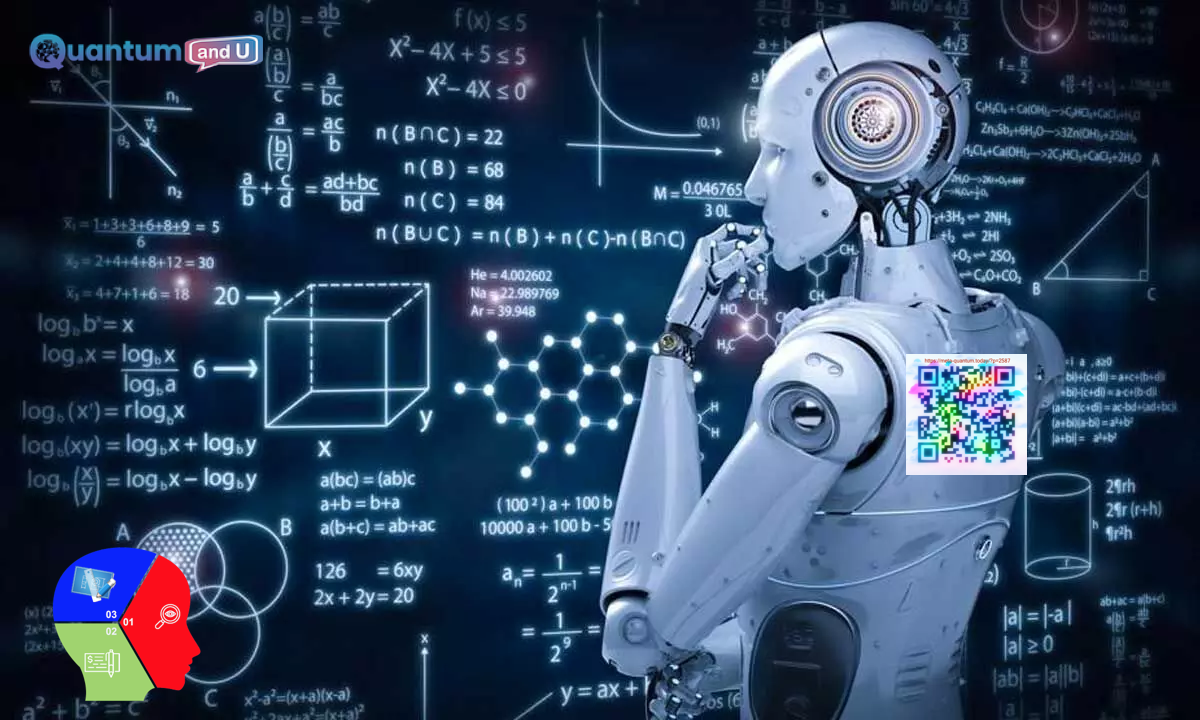
Introduction:
This paper deeply explores the multifaceted challenges that are being faced by the field of robotics in the quest for obtaining a sufficient amount of training data. This issue arises despite the exponential growth of large language models (LLMs). The internet has proven to be a rich source of data for training these LLMs, providing almost an unfathomable amount of information that can be utilized in their development. However, when it comes to acquiring high-quality, relevant, and usable data for robotics, the task becomes significantly more difficult, almost daunting, in comparison.
The discourse primarily revolves around the complexities involved in the process of developing foundational models for robots. These models form the backbone of any robotic system and their development is a crucial aspect of robotics. The paper also delves into the innovative and cutting-edge techniques that researchers and scientists are currently employing and experimenting with, in order to tackle this pressing issue. The primary aim is to shed light on these challenging aspects, and explore potential solutions that can overcome the hurdles in the path of robotic advancements.
Data Scarcity: A Challenge for Robotics, but Not an Insurmountable One
Robotics, like other AI-powered fields, faces a significant challenge: data scarcity. Training effective robotic models requires immense amounts of data, encompassing various scenarios, objects, and situations the robots might encounter. Just like a student struggles to learn a language with limited resources, robots with limited data struggle to perform complex tasks and adapt to new situations.
Researchers are not giving up. Here are some promising approaches being explored to overcome data scarcity in robotics:
1. Transfer Learning and Few-Shot Learning:
- Transfer Learning: This approach involves using a pre-trained model on a large dataset for a related task and then fine-tuning it for the specific robotics application. Imagine a chef, already familiar with many ingredients and techniques, quickly mastering a new recipe. Similarly, a robot trained on extensive general manipulation tasks can be fine-tuned for specific tasks like picking up delicate objects.
- Few-Shot Learning: This method allows robots to learn from just a few examples, mimicking how humans learn new concepts quickly based on limited observations. This approach is particularly helpful for tasks with high variability, where generating massive datasets is impractical.
2. Synthetic Data Generation:
Simulations can be used to generate vast amounts of virtual data, exposing robots to diverse scenarios without the need for real-world experimentation. While synthetic data has limitations, it can provide a valuable training ground for initial learning and can be combined with real-world data for further refinement.
3. Efficient Data Collection Techniques:
Researchers are investigating ways to collect real-world data more efficiently. Some examples include:
- Human-in-the-loop learning: Humans can provide guidance and feedback to robots during their training, allowing them to learn faster and from smaller datasets.
- Multiple robot collaboration: By sharing their experiences and data, multiple robots can collectively learn more efficiently than individual ones.
- Learning from human demonstrations: Robots can learn by observing and mimicking human demonstrations of tasks, reducing the need for extensive manual programming.
Overall, while data scarcity remains a hurdle, the combination of innovative learning techniques, synthetic data generation, and efficient real-world data collection holds promise for overcoming this challenge and advancing the field of robotics.
It’s important to remember that these are ongoing research areas, and significant progress is being made. While challenges remain, the future of robotics appears bright due to the dedication of researchers and the continuous development of novel solutions.
Video about the Robot Data Problem:
Related Video Sections:
- The Data Dilemma:
- Boston Dynamics’ transition from focusing on physical capabilities to independent intelligence sets the stage for exploring robotics’ data challenges.
- The cerebellum’s role in human motor control highlights the complexity of replicating dexterity in robots.
- Scaling solutions from balance to dexterity using more data is theorized, but high-quality data for robot motions is scarce.
- Vision-language models prove valuable for instructing robots, which primarily rely on visual feedback.
- Real World Data Collection:
- Simulations offer a viable but limited approach to generating training data, while real-world data collection is time-consuming.
- Teleoperation and research papers like Aloha, Umi gripper, and rt1 demonstrate innovative methods for gathering real-world data.
- Google’s rt2 and Auto RT leverage large language models and unsupervised learning to augment data collection and improve robot capabilities.
- Lesson from LLMS:
- Developing smarter representations can mitigate the need for extensive training data.
- Balancing the trade-off between training data and computational power is crucial for efficient model development.
- Improving algorithmic approaches like backpropagation can enhance learning efficiency in robotics.
The Impact of Robotics in Southeast Asia: Opportunities and Challenges
The rise of robotics in Southeast Asia presents both opportunities and challenges for the region. Here’s a closer look:
Opportunities:
- Increased Productivity and Efficiency: Robots can take over repetitive and dangerous tasks, allowing humans to focus on higher-level activities. This can lead to increased productivity and efficiency across various industries, like manufacturing, agriculture, and logistics.
- Enhanced Product Quality and Consistency: Robots can perform tasks with greater precision and consistency than humans, leading to higher-quality products and services. This can be particularly beneficial in sectors like electronics, automotive, and healthcare.
- Labor Cost Savings: While some job displacement may occur, the cost savings associated with automating certain tasks can be significant, making Southeast Asian companies more competitive globally. However, it’s crucial to ensure a smooth transition for displaced workers.
- New Job Creation: The robotics industry itself will create new job opportunities, including robot designers, engineers, programmers, and technicians for maintenance and operation.
- Addressing Labor Shortages: As some Southeast Asian countries face aging populations and declining birth rates, integrating robots can help address potential labor shortages in specific sectors.
Challenges:
- Job displacement: As mentioned, automation might lead to job losses, particularly in low-skilled labor-intensive industries like garment manufacturing. Governments and businesses must proactively reskill and upskill workers to equip them for the changing job market.
- Income Inequality: The benefits of automation may not be equally distributed, potentially exacerbating existing income inequality. Policies should be designed to ensure inclusive growth and ensure everyone benefits from technological advancements.
- Ethical considerations: The use of robots raises ethical concerns, such as data privacy, safety, and potential biases in decision-making algorithms. Addressing these concerns proactively is crucial for responsible adoption of robotics.
- Initial Investment Costs: Implementing and maintaining robots requires significant investment, which may be challenging for some smaller businesses and economies.
Conclusion:
Despite progress in large language models, the persistent challenge in robotics is data scarcity. Researchers are exploring various methods, such as real-world data collection and smarter representations, to overcome this obstacle. Vision-language models and unsupervised learning are promising innovations that could enhance robot capabilities. However, achieving a balance between training data and computational efficiency is crucial for future progress in robotics.
The impact of robotics in Southeast Asia is diverse. It’s essential to seize opportunities while also strategically addressing challenges through reskilling initiatives and ethical considerations.
Remember:
- The influence of robotics will differ across countries and sectors within Southeast Asia.
- Successful navigation of the region’s future work landscape depends on collaboration among governments, businesses, and educational institutions.
By adopting a responsible and proactive approach, Southeast Asia can optimize the benefits of robotics and minimize potential drawbacks.
Key Takeaways:
- Robotics faces challenges due to data scarcity, hindering advancements in autonomous systems.
- Innovative methods like teleoperation and simulation-based data collection offer partial solutions.
- Fusion of robotics with large language models opens new avenues for research and development.
- Developing smarter representations and refining algorithms are critical for overcoming data limitations in robotics.
References: