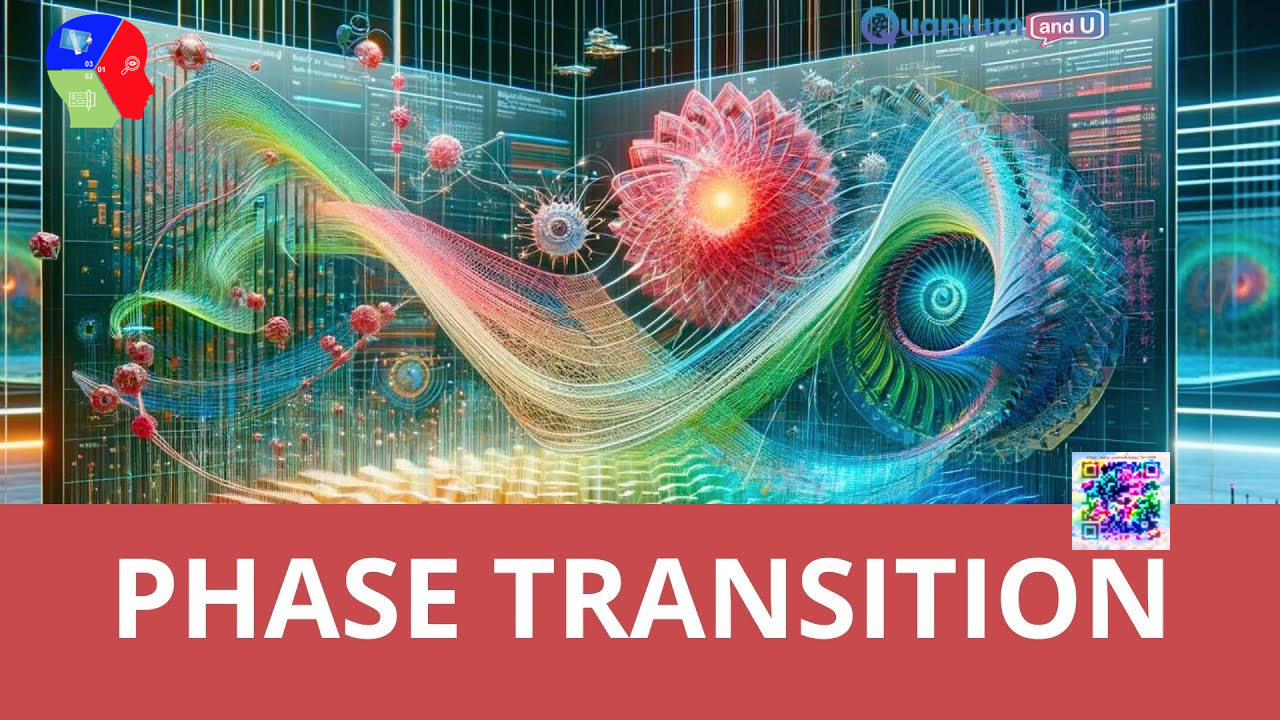
Introduction:
This article presents a detailed analysis of a captivating and complex topic. It explores the phase transition that occurs between positional learning and semantic learning in mathematical models. Specifically, the article focuses on the dot product attention mechanism within Transformer layers, which plays a crucial role in these models.
The discussion centers around a recent and exciting discovery made by researchers in Switzerland. This groundbreaking research provides new insights into the intricate dynamics of the transition between positional and semantic learning, offering valuable information about the inner workings of language models. The implications of this discovery are significant, as it has the potential to greatly enhance our understanding of how language is processed and represented in these models.
LLM phase transition:
The concept of an LLM phase transition is a fascinating and relatively new area of research in the field of large language models. Here’s a breakdown to help you understand it:
What is an LLM?
LLMs, or large language models, are AI systems trained on massive amounts of text data.They can generate text, translate languages, write different kinds of creative content, and answer your questions in an informative way. Examples of LLMs include Gemini, Bard, and GPT-3.
What is a phase transition?
In physics and chemistry, a phase transition is when a system abruptly changes its properties, like water turning to ice. Similarly, the LLM phase transition proposes that there’s a critical point in LLM size where the model undergoes a qualitative change in its capabilities.
What happens in the phase transition?
Researchers are still exploring the details, but here are some key points:
- Below the threshold: Smaller LLMs show gradual improvement with increasing size.
- At the threshold: There’s a critical point where the LLM’s capabilities suddenly jump, exhibiting emergent abilities not seen before.
- Above the threshold: The LLM continues to improve, but the gains are more dramatic compared to the pre-threshold phase. exclamation
What are the implications?
The LLM phase transition has significant implications:
- Understanding LLMs: It helps us understand how LLMs work and why they behave the way they do.
- Developing LLMs: It could guide the development of even more powerful and versatile LLMs.
- Ethical considerations: It raises ethical questions about the potential capabilities and limitations of these advanced AI systems.
What are the challenges?
Studying the LLM phase transition is complex:
- Defining the threshold: It’s challenging to pinpoint the exact size or metric where the transition occurs.
- Observing the change: Measuring the qualitative change in capabilities can be subjective and difficult to quantify.
- Replicability: Reproducing the phase transition consistently in different settings is crucial for validation. exclamation
Video about LLM Phase Transition:
Related Sections about the Video:
- Exploration of Dot Product Attention: The review begins by introducing the research conducted by the team in Switzerland, who investigated how dot product attention layers learn either positional or semantic attention matrices. They employed a non-linear self-attention layer with trainable parameters, analyzing the system in the limit of high-dimensional data with a large number of training examples.
- Phase Transition in Learning: The review highlights the significant finding of a phase transition in the system’s behavior, observed during a simple histogram task. This transition from positional to semantic attention suggests a fundamental shift in how language models perceive and process information, akin to a phase transition in theoretical physics.
- Implications for Model Training: The review discusses the potential implications of understanding this phase transition for training large language models. It suggests that by pinpointing the conditions under which the transition occurs, researchers could develop more efficient training mechanisms, leading to faster and more accurate models.
- Challenges and Opportunities: The review also addresses the limitations of models stuck in the positional phase, emphasizing their struggle to comprehend complex semantic tasks. However, understanding and leveraging the phase transition could lead to improved model adaptability and performance across a wider range of tasks and domains.
Impact of the LLM phase transition on SEA:
The potential impact of the LLM phase transition on Southeast Asia and the market opportunities it presents are diverse and complex. Here’s a breakdown of some key areas to consider:
Positive impacts:
- Economic growth: LLMs can improve efficiency and productivity across various sectors like agriculture, manufacturing, and logistics services. This can stimulate economic growth and create new jobs.
- Education and skills development: LLMs can personalize learning experiences, translate educational materials, and automate administrative tasks, making education more accessible and efficient.
- Language translation and communication: LLMs can break down language barriers, facilitating communication and collaboration across different cultures and regions. This can be particularly beneficial in Southeast Asia with its diverse linguistic landscape.
- Content creation and media industry: LLMs can assist in content creation for marketing, entertainment, and news, boosting the media industry in the region.
- Government services and citizen engagement: LLMs can improve government service delivery, automate tasks, and facilitate citizen engagement through chatbots and virtual assistants.
Market opportunities:
- Developing and deploying LLMs tailored to Southeast Asian languages and cultures: There’s a need for LLMs that understand the nuances of local languages and cultural contexts. Companies developing and deploying such models can cater to a large and growing market.
- Building applications and services powered by LLMs: Enterprises can leverage LLMs to automate tasks, improve customer service, and personalize their offerings. This creates opportunities for startups and established companies to develop innovative applications and services.
- Investing in LLM research and development: Governments and universities can invest in LLM research to develop advanced models and applications relevant to the region’s needs.
- Providing data and infrastructure for LLM development: Companies can offer data and infrastructure solutions to support the development and deployment of LLMs in the region.
Challenges:
- Digital divide: Unequal access to technology and the internet can limit the benefits of LLMs for certain populations. Bridging the digital divide is crucial.
- Data privacy and security: LLMs rely on large amounts of data, raising concerns about privacy and security. Robust data protection regulations and ethical AI practices are essential.
- Job displacement: Automation through LLMs could lead to job displacement in certain sectors. Upskilling and reskilling initiatives are needed to ensure a smooth transition.
- Bias and discrimination: LLMs trained on biased data can perpetuate discriminatory outcomes. Careful data selection and mitigation strategies are crucial to prevent biased outputs.
Conclusion:
The review emphasizes the significance of this discovery and its potential impact on the development of language models. It suggests that optimizing training processes and data presentation can improve model performance while reducing computational resources and training time. Additionally, understanding the phase transition may lead to new model architectures and learning strategies, advancing the field of natural language processing.
In this conclusion, the LLM phase transition presents both opportunities and challenges for Southeast Asia. By addressing the challenges and leveraging the potential, the region can benefit from the advancements in LLMs and ensure their contribution to inclusive and sustainable development.
It is important to note that this is a rapidly evolving field, and the specific impacts and opportunities may vary depending on the country, industry, and application. Therefore, staying informed and adapting to the changing landscape will be crucial for individuals and organizations in Southeast Asia to maximize the potential of LLMs.
Key Takeaway Points:
- The phase transition from positional to semantic attention in language models has significant implications for model training and performance.
- Understanding this transition could lead to more efficient training mechanisms, faster model convergence, and improved adaptability across tasks.
- Leveraging the phase transition could result in models that are more interpretable, transparent, and capable of handling complex linguistic tasks with greater accuracy.
References:
- A Simple Explanation for the Phase Transition in Large Language Models with List Decoding: https://arxiv.org/pdf/2303.13112** by Vamsi K Amalladinne, Jean-Francois Chamberland, and Krishna R Narayanan
- Study of the possibility of phase transitions in LLMs
- How LLMs are impacting Southeast Asian telcos