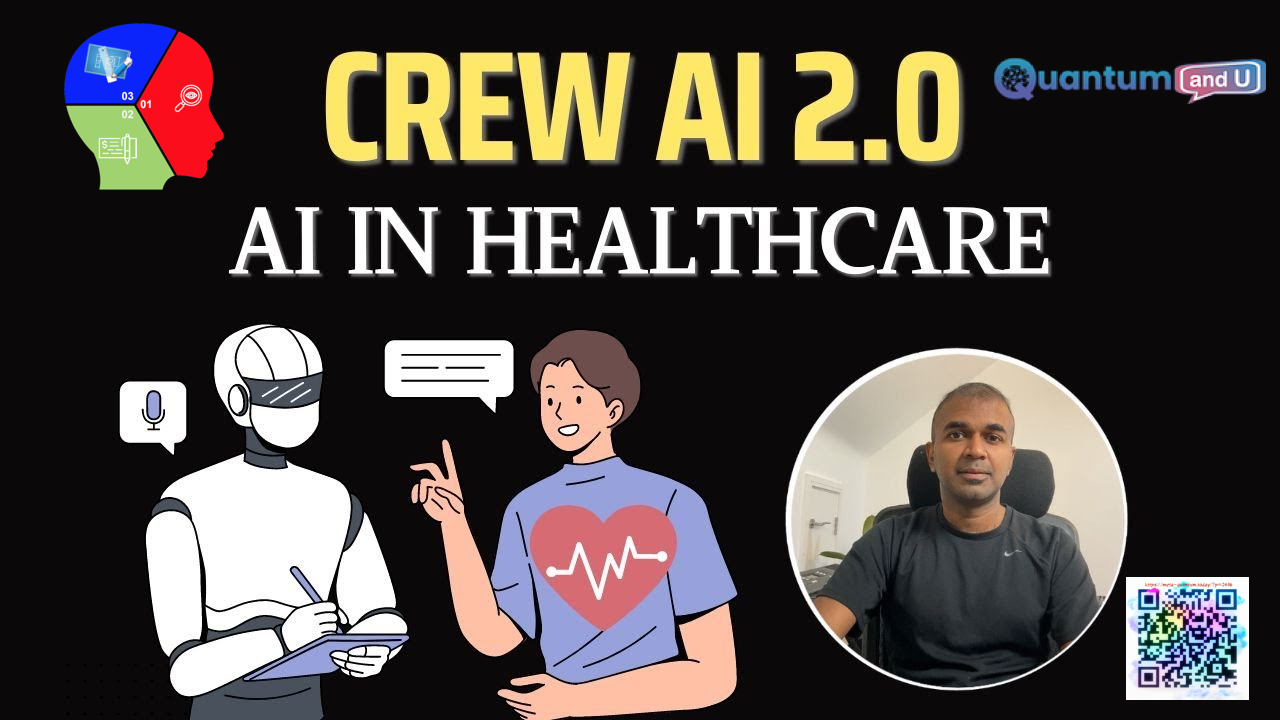
Introduction:
In this section, we will explore the groundbreaking developments of Crew AI 2.0. It demonstrates the remarkable capabilities of AI agents working together in healthcare research. This new version introduces exciting features such as hierarchical task performance, asynchronous requests, and improved coordination among agents. These advancements represent a significant departure from the traditional approach of sequential task execution and instead provide a more efficient and sophisticated hierarchical framework. By harnessing the power of AI, Crew AI 2.0 revolutionizes healthcare research, offering countless possibilities for collaboration and innovation in the field.
Crew AI 2.0: Collaborative AI Agents for Complex Tasks:
Crew AI is a framework for building autonomous AI agents designed to work together collaboratively to tackle complex tasks. This collaboration aspect distinguishes it from single-agent frameworks like X-Agent or Autogen.
Key Features in Healthcare Research
- Simultaneous Research: Crew AI 2.0 introduces a hierarchical task structure, allowing multiple AI agents to work on different aspects of a research project simultaneously. This can significantly speed up the research process.
- Manager and Agent Roles: The manager agent assigns tasks to researcher and writer agents, who then coordinate and communicate to complete the task efficiently.
- Seamless Collaboration: Crew AI facilitates smooth communication and delegation among agents, ensuring tasks are completed in a coordinated manner.
- Flexibility and Adaptability: The framework can be adapted to various healthcare research scenarios, from literature reviews to data analysis and report generation.
Potential Applications
- Literature Reviews: Agents can scan vast databases, summarize relevant articles, and identify key findings.
- Data Analysis: Agents can analyze large datasets, identify patterns, and generate insights.
- Report Generation: Agents can draft reports based on research findings, saving researchers time and effort.
- Drug Discovery: Agents can screen vast chemical libraries to identify potential drug candidates.
- Clinical Trials: Agents can analyze data from clinical trials and support researchers in drawing conclusions.
Benefits
- Increased Efficiency: Simultaneous research and collaboration can significantly accelerate research processes.
- Improved Accuracy: Combining the expertise of multiple agents can lead to more accurate and comprehensive results.
- Reduced Costs: Automating tasks can free up researchers’ time and potentially reduce research costs.
- Scalability: Crew AI can be scaled to handle large and complex research projects.
Video About AI 2.0 – AI in Healthcare:
Related Sections about the Video:
- Setting Up Crew AI 2.0:
- The video begins with an overview of the setup process, including installation steps and prerequisite packages such as Crew AI, Doug Go Search, and Lang Chain Community.
- Viewers are guided through exporting the OpenAI API key and creating necessary files for implementation.
- Defining Agents and Tasks:
- Detailed instructions are provided on defining different agents, such as managers, researchers, and writers, each assigned specific roles within the research process.
- The significance of the callback function is highlighted, showcasing its role in automating post-task actions, such as sending notifications.
- Creating Custom Tools and Tasks:
- The video illustrates the creation of custom tools, including content extraction from web pages, to enhance research capabilities.
- Tasks are defined with asynchronous execution, enabling concurrent processing of multiple requests by agents.
- Hierarchical Task Execution:
- The transition from sequential to hierarchical task execution is explained, emphasizing improved workflow management and task coordination.
- The demonstration includes examples of tasks running concurrently and their subsequent integration into the final report.
- Incorporating Human and External Models:
- Insights are provided on incorporating human input and external language models, such as Ola, to augment research capabilities while maintaining data privacy.
- Practical examples showcase the versatility of Crew AI 2.0 in accommodating diverse research needs.
Impact of Crew AI 2.0 in SEA’s Healthcare Industry:
Positive Impacts:
- Increased Research Efficiency: The ability to conduct research simultaneously could significantly accelerate the pace of medical discoveries and innovation in Southeast Asia, where research resources are often limited.
- Improved Healthcare Outcomes: More accurate and comprehensive research findings could lead to better diagnosis, treatment, and disease prevention strategies, ultimately improving healthcare outcomes for the region’s population.
- Reduced Healthcare Costs: Automating tasks and improving efficiency could potentially reduce research and development costs, making healthcare more affordable in Southeast Asia.
- Enhanced Accessibility: AI-powered healthcare research could be particularly beneficial in remote and underserved areas, where access to specialists and advanced healthcare facilities is limited.
- Boosted Economic Growth: The development and application of Crew AI 2.0 could create new jobs, attract investments, and contribute to the growth of the healthcare technology sector in Southeast Asia.
Market Opportunities:
- Developing and Selling Crew AI 2.0 Applications: Companies can develop and sell Crew AI 2.0 applications tailored to the specific needs of healthcare researchers and institutions in Southeast Asia.
- Providing Data and Training Services: Companies can offer data curation and training services to help researchers train Crew AI 2.0 agents on Southeast Asian-specific datasets.
- Consulting and Implementation Services: Companies can provide consulting and implementation services to help healthcare institutions integrate Crew AI 2.0 into their research workflows.
- Partnering with Research Institutions and Government Agencies: Collaborations between technology companies, research institutions, and government agencies can accelerate the development and adoption of Crew AI 2.0 in Southeast Asia.
Challenges and Considerations:
- Data Availability and Quality: Access to high-quality, diverse, and anonymized healthcare data is crucial for training Crew AI 2.0 effectively in the Southeast Asian context.
- Infrastructure and Technological Limitations: Some parts of Southeast Asia may lack the necessary infrastructure and technological capabilities to fully utilize Crew AI 2.0.
- Ethical Concerns: Ethical considerations surrounding data privacy, AI bias, and transparency in decision-making need to be addressed carefully.
- Regulatory Landscape: The regulatory landscape for AI in healthcare varies across Southeast Asian countries, and companies need to navigate these regulations carefully.
Overall, Crew AI 2.0 has the potential to significantly impact the healthcare industry in Southeast Asia. However, addressing the challenges and ensuring responsible development and implementation are crucial for realizing its full potential.
Conclusion:
Crew AI 2.0 represents a promising approach to collaborative AI-driven healthcare research. While challenges remain, its potential to accelerate research, improve accuracy, and reduce costs make it a valuable tool for researchers and healthcare professionals.
Takeaway Key Points: The review concludes with key takeaways and insights into the capabilities of Crew AI 2.0:
- Enhanced hierarchical task execution facilitates seamless coordination among AI agents, leading to more efficient research outcomes.
- Integration of custom tools and asynchronous processing expands research capabilities, allowing for simultaneous handling of multiple tasks.
- Flexibility in incorporating human input and external models ensures adaptability to diverse research requirements while safeguarding data privacy.
Related References: For further exploration and implementation of Crew AI 2.0, viewers are encouraged to refer to the following resources: