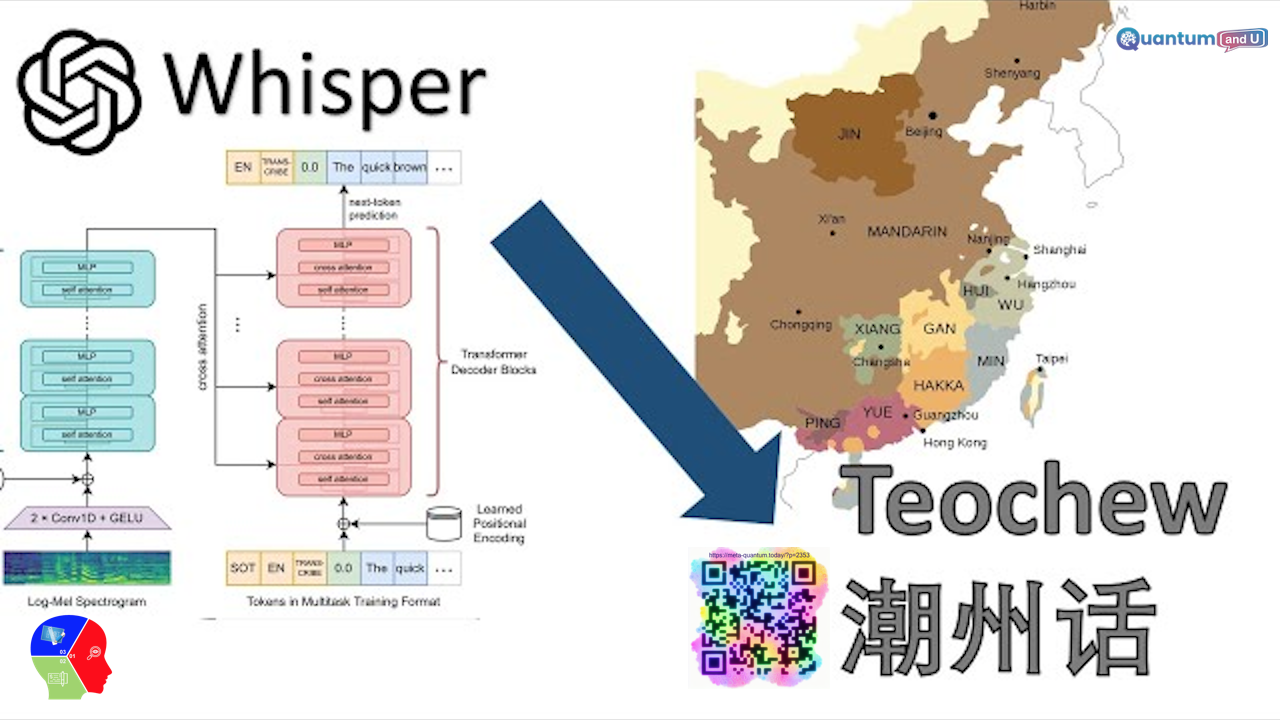
Introduction:
The video provides a detailed demonstration of the step-by-step process for fine-tuning OpenAI’s Whisper model specifically to recognize a less-known Chinese dialect called Teochew. The presenter not only explains the technical aspects of the fine-tuning process, but also discusses the linguistic insights that have contributed to the development of this model. Furthermore, the video highlights the challenges of acquiring data for a low-resource language like Teochew and emphasizes the importance of overcoming these obstacles. By showcasing the overall methodology used in the fine-tuning process, the video offers valuable insights and knowledge for those interested in natural language processing and dialect recognition.
Fine-tuning Whisper for Teochew:
Fine-tuning Whisper for your Chinese dialect, Teochew, is an exciting project with the potential to improve speech recognition accuracy for your specific language variety. Here’s what you can consider:
Data Collection:
- The most crucial aspect is gathering a high-quality dataset of Teochew speech recordings. This includes audio files with corresponding transcripts. Ideally, the recordings should cover diverse speakers, accents, and speaking styles to represent the full range of Teochew speech.
- You can collect data through self-recordings, crowdsourcing platforms, or collaborating with Teochew communities and organizations. Aim for a balanced dataset with enough representative samples.
Model Selection:
- While Whisper is a powerful speech recognition model, consider exploring other options specifically trained on Chinese dialects. You can find pre-trained models for other Sinitic languages like Min Nan or Hakka, which might serve as a better starting point for fine-tuning on Teochew.
- If pre-trained models aren’t available, you can start with a general Chinese speech recognition model and fine-tune it on your Teochew dataset.
Fine-tuning Process:
- Once you have the data and chosen model, you’ll need to set up a training environment with libraries like TensorFlow or PyTorch. Tools like Hugging Face Transformers can simplify the fine-tuning process.
- The fine-tuning involves adjusting the model’s parameters based on your Teochew dataset. This requires expertise in machine learning and natural language processing.
- Consider seeking help from online communities or collaborating with developers familiar with speech recognition and dialect-specific language models.
Evaluation and Refinement:
- After fine-tuning, evaluate the model’s performance on unseen Teochew speech data. Metrics like word error rate (WER) can help assess the accuracy improvement compared to the original model.
- Based on the evaluation, you can further refine the model by adjusting hyperparameters or collecting more data for specific areas where the model struggles.
Additional Considerations:
- Teochew has its own unique vocabulary and pronunciation compared to Mandarin. Ensure your training data reflects these differences effectively.
- Consider incorporating linguistic resources like Teochew dictionaries and pronunciation guides to improve the model’s understanding of the language.
- Be mindful of potential biases in your data and training process. Aim for a diverse and representative dataset to avoid perpetuating biases in the final model.
Fine-tuning Whisper for Teochew is a challenging but rewarding project. With careful planning, data collection, and technical expertise, you can contribute to the development of speech recognition technology for under-resourced languages like Teochew.
Video about Fine-Tuning Whisper for Teochew:
Related Sections in this video:
- Introduction to Teochew Dialect:
- Overview of Teochew, a Chinese dialect with 10 million speakers.
- Unique characteristics and its position in the Minan language family.
- Differences from Mandarin and Cantonese.
- Transfer Learning from Mandarin to Teochew:
- Rationale behind choosing Mandarin as a source language.
- Linguistic similarities and differences between Mandarin and Teochew.
- Focus on syntax, word order, phonology, and vocabulary.
- Data Collection and Preprocessing:
- Challenges in obtaining Teochew data, resorting to OCR for subtitles.
- Manually sourcing 60 hours of video data with Teochew speech.
- Data preprocessing steps, including optical character recognition.
- Whisper Model Overview:
- Introduction to the Whisper model, designed for automatic speech recognition (ASR).
- A standard encoder-decoder Transformer architecture with audio input.
- Pre-training on 680,000 hours of multilingual data, available in five sizes.
- Fine-tuning Process:
- Detailed steps involved in fine-tuning the Whisper model on Teochew data.
- Refactoring code for better data set loading using Hugging Face.
- Considerations for batch size, gradient accumulation, and GPU capabilities.
- Evaluation and Results:
- Word error rate analysis on the test set.
- Visualization of training loss curve and model performance on different data sizes.
- Qualitative evaluation of model performance on various words and phrases.
- Comparison with Different Whisper Model Sizes:
- Testing Whisper models of different sizes on Teochew data.
- Insights into the impact of model size on performance improvement.
- Qualitative Evaluation:
- Evaluation of model performance on specific words, considering cognates and frequency.
- Demonstrating challenges with conversational speech and short-length data.
- Interactive Demo:
- Implementation of a Gradio script for an interactive UI with the fine-tuned model.
- Engaging with the model through sentences in Teochew and English for qualitative assessment.
Impact of using AI Fine-Tuning local language in SEA:
Fine-tuning AI for local languages in Southeast Asia has the potential to bring significant positive impacts across various sectors, but also presents some challenges that need to be addressed. Here’s a breakdown of both:
Positive Impacts:
- Improved access to information and technology: By making AI applications and online content understandable in local languages, more people can participate in the digital economy and access essential information like healthcare, education, and government services. This can bridge the digital divide and empower marginalized communities.
- Enhanced cultural preservation and expression: Fine-tuning AI for local languages allows for the creation of tools and platforms that promote and document cultural heritage, languages, and traditions. This can contribute to cultural diversity and identity preservation in the region.
- Boost to economic development: AI applications tailored to local languages can improve communication and collaboration within businesses, leading to increased productivity and efficiency. This can attract foreign investment and stimulate economic growth in the region.
- More personalized and effective AI services: Chatbots, virtual assistants, and other AI-powered services can be more helpful and relevant if they understand and respond in local languages. This can improve customer satisfaction and user experience.
- Greater participation in the global AI ecosystem: By developing expertise in local language AI, Southeast Asian countries can contribute to the advancement of AI research and development globally. This can lead to knowledge sharing and collaboration on AI solutions for various challenges.
Challenges to Consider:
- Data availability and quality: Training AI models for low-resource languages often requires large amounts of labeled data, which can be difficult and expensive to collect. This can lead to limitations in the accuracy and performance of the models.
- Technical expertise and infrastructure: Developing and deploying AI solutions in local languages requires technical expertise in machine learning, natural language processing, and software development. This can be a challenge for countries with limited resources and infrastructure.
- Potential for bias and discrimination: AI models trained on biased data can perpetuate and amplify existing societal inequalities. It’s crucial to ensure fairness and inclusivity when developing and deploying AI solutions in local languages.
- Ethical considerations and privacy concerns: Collecting and using personal data for AI training raises ethical concerns about privacy and data security. Robust regulations and ethical frameworks are needed to ensure responsible development and use of AI in local languages.
Conclusion:
The video concludes with a quick and fun demonstration using the fine-tuned Whisper model in an interactive UI. The presenter encourages viewers to express interest in the video and mentions plans to make the model open source.
In conclusion, fine-tuning AI for local languages in Southeast Asia presents a promising opportunity for inclusive development and cultural preservation. However, it is crucial to address the challenges and ensure responsible development in order to maximize the positive impacts and mitigate potential risks.
Takeaway Key Points:
- Fine-tuning Whisper for a low-resource language involves unique challenges.
- Mandarin serves as a high-resource language for transfer learning to Teochew.
- Data collection includes manual sourcing of video data and OCR for subtitles.
- Detailed insights into the Whisper model architecture and fine-tuning process.
- Evaluation metrics, including word error rate, demonstrate model performance.
- Consideration of different model sizes and their impact on performance.
- Qualitative assessment highlights the model’s strengths and challenges.
- Interactive demo using Gradio for user engagement and model exploration.
Related References: