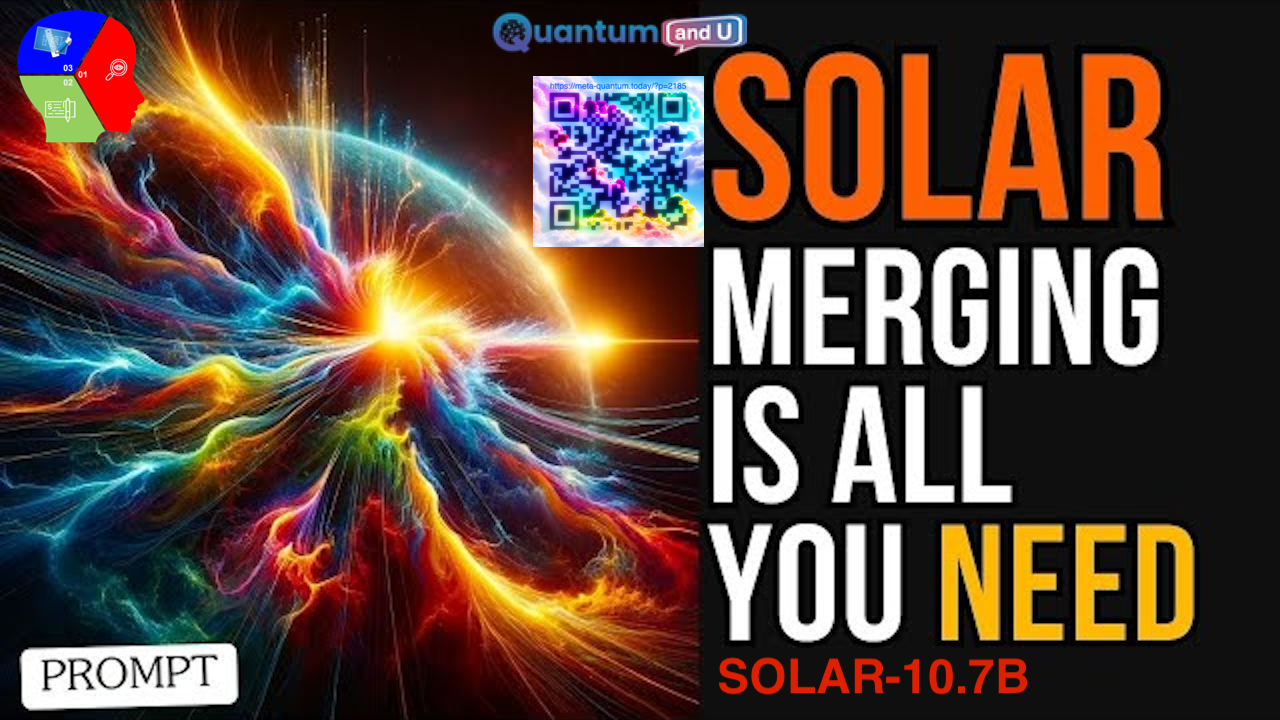
Introduction:
The blog post extensively discusses the groundbreaking and innovative SOLAR-10.7B model, which currently holds the top position on the open LLM Leaderboard on Hugging Face. This model is truly exceptional as it combines the strengths of multiple models, demonstrating its superiority even in comparison to larger alternatives such as Mixtral MoE. The author goes into great detail about the fascinating depth-up scanning technique employed by this model, offering valuable insights into its intricate architecture and impressive performance. Additionally, the blog provides a comprehensive analysis of the various aspects that contribute to the success and effectiveness of the SOLAR-10.7B model, ensuring a thorough understanding of its capabilities and potential applications.
SOLAR-10.7B: Merging Models – The Next Big Thing in AI?
The idea of merging different large language models (LLMs) like SOLAR-10.7B has gained significant traction in recent times, and for good reason. It holds the potential to unlock new levels of performance and capabilities in AI, potentially surpassing what individual models can achieve.
Here’s a breakdown of the concept and its potential implications:
What is Model Merging?
Model merging, also known as ensemble learning in the context of LLMs, involves combining multiple pre-trained models into a single, unified model. This can be done in various ways, such as:
- Parameter averaging: Averaging the weights of the individual models to create a new set of weights for the merged model.
- Knowledge distillation: Training a smaller model on the outputs of the larger models, effectively capturing their knowledge in a more compact form.
- Model fusion: Combining the outputs of different models through a gating mechanism to produce a more informed final output.
Benefits of Model Merging:
- Improved performance: Merged models can potentially outperform individual models on various tasks, such as question answering, summarization, and code generation. This is because they leverage the strengths of each individual model and mitigate their weaknesses.
- Enhanced robustness: Merged models are generally more robust to noise and errors in the data they are trained on. This is because they have a broader and more diverse set of knowledge representations.
- Increased flexibility: By combining different models with specialized skills, merged models can tackle a wider range of tasks and adapt to different contexts more effectively.
Challenges and Considerations:
- Complexity: Merging models can be computationally expensive and require careful design to avoid introducing new errors or biases.
- Interpretability: Understanding how merged models arrive at their outputs can be challenging, making it difficult to debug or trust their decisions.
- Data requirements: Training and fine-tuning merged models often require even more data than individual models, which can be a bottleneck in some cases.
The Future of Model Merging:
Despite the challenges, model merging is a promising area of research with the potential to revolutionize the field of AI. As research progresses and computational resources become more powerful, we can expect to see even more sophisticated and effective merging techniques emerge.
SOLAR-10.7B specifically:
While the specific details of SOLAR-10.7B and its merging approach are not widely available, the sheer size of the model (10.7B parameters) suggests it has the potential to be a valuable component in a merged model ensemble. Its combination with other specialized models could lead to significant advancements in various AI domains.
Overall, model merging is a fascinating concept with the potential to shape the future of AI. It’s an area worth watching closely as researchers continue to explore its possibilities and push the boundaries of what’s possible with large language models.
Video about the SOLAR-10.7B:
Sections about the video:
- Depth-Up Scanning Technique:
- SOLAR-10.7B’s uniqueness lies in depth-up scanning, a method where a 32-layer Llama 2 base model is combined with pre-trained weights from Mixtral 7B.
- Copies of the model are created, and the top or bottom eight layers are removed, resulting in a 48-layer model with 10.7 billion parameters.
- Unlike MoE, depth-up scanning simplifies training and doesn’t require additional modules.
- Training and Fine-Tuning:
- The blog explains the continued pre-training of SOLAR-10.7B, including an instruct fine-tune version.
- Fine-tuning occurs in two stages: instruct fine-tuning and alignment tuning through DPO.
- Data contamination test results reveal minimal contamination on benchmarks used for instruct fine-tuning.
- Benchmark Results and Model Testing:
- Despite having only 11 billion parameters, SOLAR-10.7B achieves state-of-the-art results on the LLM Leaderboard.
- The author tests the model using the Transformer package from Hugging Face, demonstrating its logical reasoning and creative writing capabilities.
- The model outperforms in generating responses to various prompts, including ethical dilemmas and logical questions.
- Programming Capabilities:
- SOLAR-10.7B showcases proficiency in programming tasks, successfully writing Python scripts and generating HTML code for specific functions.
- The model excels in handling creative writing tasks, providing contextually relevant and coherent responses.
Impact of SOLAR-10.7B in AI to SEA and Market Size:
The potential impact of SOLAR-10.7B, a 10.7 billion parameter large language model (LLM), on AI in Southeast Asia is significant and multifaceted, with implications across various sectors and applications. Here’s a breakdown of its potential impact and market size:
Impact Areas:
- Natural Language Processing (NLP) for local languages: SOLAR-10.7B’s massive dataset could be fine-tuned to understand and process diverse Southeast Asian languages, enabling advancements in NLP tasks like machine translation, chatbots, and virtual assistants tailored to the region’s needs.
- Machine translation for regional communication: Breaking down language barriers is crucial for Southeast Asia’s interconnectedness. SOLAR-10.7B could power highly accurate and nuanced machine translation systems, facilitating communication between communities and fostering regional collaboration.
- Content creation and summarization for local news and media: The model’s ability to generate and summarize text could be harnessed to create engaging and informative content in local languages, catering to the region’s diverse news and media landscape.
- Education and research with access to vast information: SOLAR-10.7B could democratize access to information and personalized learning experiences for students and researchers across Southeast Asia. Its ability to answer complex questions and generate different creative text formats could revolutionize education and research.
- Agriculture and environmental monitoring with data analysis: The model’s ability to analyze large datasets could be applied to agriculture and environmental monitoring in Southeast Asia. This could lead to improved crop yields, natural resource management, and disaster preparedness.
- Business and finance with improved market insights and automation: Businesses in Southeast Asia could leverage SOLAR-10.7B for market analysis, customer service automation, and even generating financial reports. This could streamline operations, boost efficiency, and gain valuable market insights.
- Government services and citizen engagement with AI assistance: Governments in Southeast Asia could utilize SOLAR-10.7B to improve citizen services, provide information, and even gather feedback through chatbots and virtual assistants. This could enhance government transparency and citizen engagement.
Market Size:
Estimating the market size for SOLAR-10.7B’s applications in Southeast Asia is challenging due to several factors, including:
- Varying adoption rates across sectors and countries: Different sectors and countries within Southeast Asia have varying levels of technological infrastructure and AI readiness, impacting adoption rates.
- Specific applications and value propositions: The market size will depend on the specific applications and value propositions developed using SOLAR-10.7B, which are still evolving.
- Data availability and privacy concerns: Access to and privacy considerations surrounding data required to fine-tune and deploy SOLAR-10.7B applications will play a role in market size.
However, considering the vast potential of SOLAR-10.7B across various sectors and its ability to address critical regional needs, the market size for its applications in Southeast Asia is estimated to be significant, potentially reaching billions of dollars in the long term.
It’s important to note that these are just potential impacts and market size estimations. The actual realization of these possibilities will depend on various factors, including ongoing research, development, and adaptation of SOLAR-10.7B for specific Southeast Asian contexts.
Conclusion:
SOLAR-10.7B, a highly remarkable model, has gained significant attention not only for its exceptional performance on the leaderboard but also for its revolutionary method of merging models through depth-up scanning. This unique approach sets SOLAR-10.7B apart from other models and showcases its advanced capabilities in creative writing and programming tasks. However, it is important to note that SOLAR-10.7B does have certain limitations when it comes to logical reasoning. Nevertheless, SOLAR-10.7B presents itself as a promising alternative to MoE (Mixture of Experts), paving the way for future advancements in model development and opening up new possibilities in the field.
5 Key Takeaways:
- Depth-Up Scanning Innovation: SOLAR-10.7B merges models using depth-up scanning, offering a novel alternative to traditional architectures.
- Compact yet Powerful: With only 11 billion parameters, the model surpasses larger counterparts, showcasing efficiency in performance.
- Instruct Fine-Tuning Success: The instruct fine-tuning process, coupled with alignment tuning, contributes to the model’s robustness and effectiveness.
- Versatile in Prompt Responses: SOLAR-10.7B exhibits versatility in generating responses to various prompts, excelling in creative writing and programming tasks.
- Future Potential: The model’s architecture hints at future possibilities, suggesting it could be a viable alternative or complementary approach, especially if combined with mixture of experts.