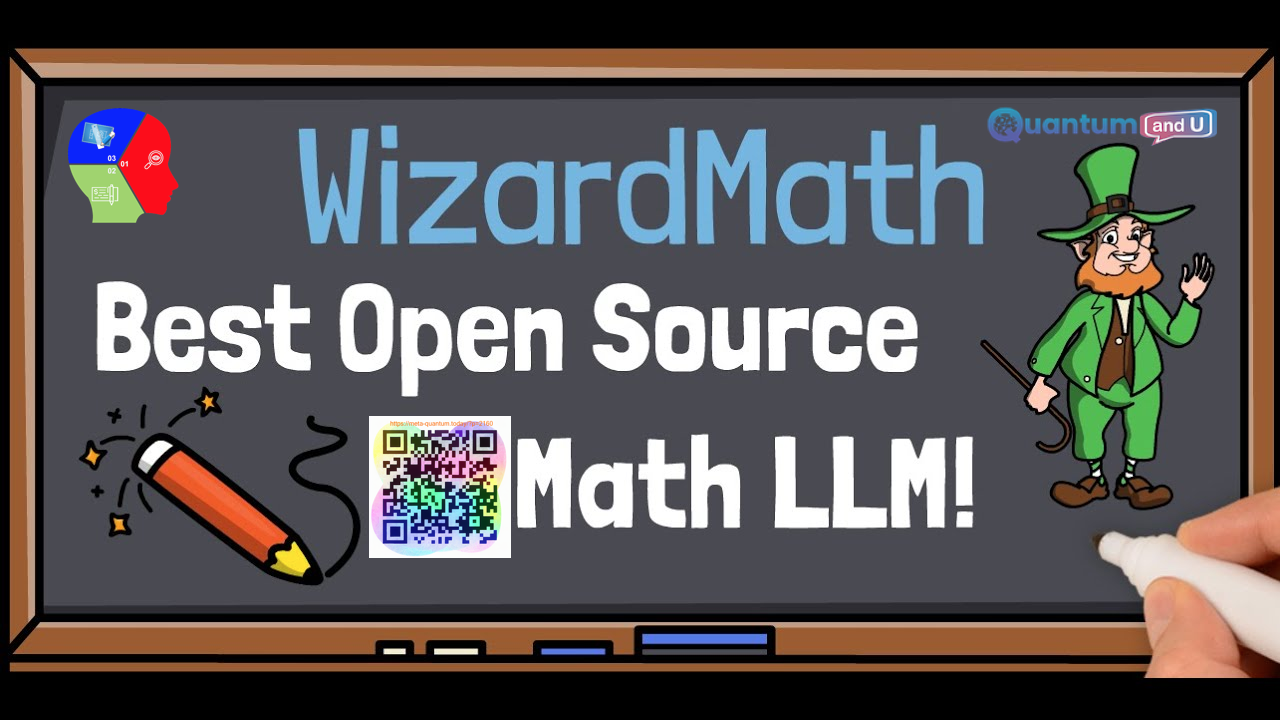
Introduction:
WizardMath, a groundbreaking open source large language model from Microsoft designed for mathematical reasoning. Unlike other large language models, WizardMath combines the strengths of top models like GPT-4 with open source accessibility. In this video, we’ll delve into the research paper introducing WizardMath, titled “WizardMath: Empowering Mathematical Reasoning for Large Language Models via Reinforced Evol-Instruct.” By the end of this presentation, you’ll gain insights into the model’s creation and the innovative Reinforced Evol-Instruct method.
WizardMath from Microsoft:
WizardMath, developed by Microsoft, certainly piques the interest of anyone working with large language models (LLMs) and mathematical applications. Here’s what I found noteworthy about it:
Open Source: This is a significant aspect, as it allows for greater transparency, collaboration, and potential for improvement by the broader AI community. Open-sourcing an LLM like WizardMath can accelerate research and development in the field of mathematical AI.
Focus on Math: Unlike many LLMs trained on general text data, WizardMath specifically targets mathematical problems and reasoning. This tailored training allows it to handle complex mathematical concepts and perform tasks like theorem proving, formula generation, and equation solving more effectively.
Reinforced Evol-Instruct: This intriguing training method combines reinforcement learning with evolutionary algorithms and instructive prompts. This approach could potentially lead to more efficient and effective learning compared to traditional LLM training methods.
Potential Applications: The possibilities for WizardMath are vast, ranging from assisting mathematicians and scientists in their research to powering educational tools and intelligent tutoring systems. Its ability to reason about and generate mathematical concepts could revolutionize how we interact with and learn mathematics.
However, it’s important to remember that WizardMath is still under development. While its open-source nature and focus on math are promising, its capabilities and limitations need further evaluation and testing.
Here are some additional questions to consider:
- How does WizardMath compare to other math-focused LLMs like DeepMind’s AlphaGo Zero or Google’s LaMDA?
- What are the specific tasks that WizardMath excels at, and what are its current limitations?
- How can the open-source community contribute to the development and improvement of WizardMath?
Overall, WizardMath is a fascinating development in the field of AI and mathematics. Its open-source nature and focus on math hold significant promise for the future of mathematical computing and research. As we learn more about its capabilities and limitations, we can better understand its potential impact on how we interact with and understand mathematics.
Enjoy the Introduction of WizardMath Video:
Related Sections for the video:
- Model Creation:
- The video starts by explaining the three-step creation process. It begins with supervised fine-tuning, utilizing a dataset constructed from math instruction and response pairs.
- The dataset is generated by sampling 15k pairs from GSM8k and MATH datasets, transforming responses into a step-by-step format for enhanced understanding.
- The fine-tuned model is named WizardMath, setting the stage for subsequent steps.
- Evol-Instruct Background:
- A brief overview of Evol-Instruct, a method for evolving datasets by using a large language model to rewrite and enhance instructions.
- Explains in-depth and in-breadth evolving techniques, highlighting the significance of diverse and complex instructions.
- Reinforced Evol-Instruct Approach:
- Introduces a novel reinforcement learning approach called Reinforced Evol-Instruct.
- Describes the creation of two reward models: Instruction Reward Model (IRM) for instruction quality and Process-Supervised Reward Model (PRM) for ranking steps in the response.
- Details the creation of instruction ranking and steps ranking datasets for training the reward models.
- Active Evol-Instruct and PPO Training:
- Illustrates the active Evol-Instruct and PPO training process, where evolved instructions are iteratively added to the evolving dataset.
- Highlights the multiplication of rewards from IRM and PRM to get the final reward, leading to Proximal Policy Optimization (PPO) updates.
- Results and Comparisons:
- Presents results comparing WizardMath with other open source models, showcasing its impressive performance on GSM8k and MATH datasets.
- Compares WizardMath with closed source models, indicating its competitive standing and room for improvement.
Impact of Open Source Math LLM with Reinforced Evol-Instruct on SEA’s Education and Market Size:
Positive Impacts:
- Improved Learning Outcomes: WizardMath’s ability to personalize learning, provide targeted feedback, and automate repetitive tasks could significantly improve student engagement and understanding in mathematics. This could lead to higher test scores, graduation rates, and overall academic achievement.
- Increased Access to Quality Education: Open-source nature makes it more affordable and accessible for schools and educators across Southeast Asia, particularly in rural or under-resourced areas. This could help bridge the educational gap and provide quality math education to students regardless of their background or location.
- Teacher Support and Professional Development: WizardMath can assist teachers in lesson planning, grading, and providing differentiated instruction. This can free up teachers’ time to focus on more personalized interactions and professional development, ultimately improving the overall quality of education.
- Development of Local Language Support: The open-source nature allows for customization and adaptation to local languages and dialects, making it more relevant and accessible to students in Southeast Asia. This can address language barriers and improve learning outcomes for diverse student populations.
- Boosting STEM Education: WizardMath’s focus on math could encourage more students to pursue STEM fields, which is crucial for Southeast Asia’s economic development and technological advancement.
Market Size:
- Estimating the exact market size for WizardMath in Southeast Asia is challenging due to its early stage of development and the diverse education landscape. However, considering the region’s growing population, increasing internet penetration, and rising demand for quality education, the potential market is significant.
- Factors like government support for educational technology integration, private sector investments, and the adoption rate by schools and educators will influence market growth.
- Reports suggest the Southeast Asian EdTech market is expected to reach $7.3 billion by 2025, with a CAGR of 18.5%. Open-source LLMs like WizardMath could capture a significant share of this market, especially if they prove effective and cost-effective.
Challenges:
- Infrastructure and Connectivity: Unequal access to reliable internet and technology infrastructure in some parts of Southeast Asia could hinder widespread adoption of WizardMath.
- Teacher Training and Support: Teachers may require training on effectively integrating WizardMath into their teaching practices to maximize its benefits.
- Data Privacy and Security: Concerns regarding student data privacy and security need to be addressed to ensure trust and ethical implementation of the technology.
Conclusion:
In conclusion, WizardMath is a powerful open-source large language model for mathematical reasoning, surpassing existing models in performance. The Reinforced Evol-Instruct approach, combined with active training and PPO optimization, contributes to its success. While it compares favorably with closed-source models, there is room for further improvement. If you enjoyed this content, please subscribe and hit the like button to stay updated on more insightful videos.
Overall, an Open Source Math LLM with Reinforced Evol-Instruct, like WizardMath, has the potential to make a significant impact on education in Southeast Asia. It can improve learning outcomes, increase access to quality education, and support STEM education. However, addressing challenges related to infrastructure, teacher training, and data privacy is crucial for successful implementation and market adoption.
It is important to note that this is only an initial assessment, and further research is needed to accurately predict the impact and market size of WizardMath in SEA. However, its potential to revolutionize math education in the region is undeniable.
Takeaway Key Points:
- WizardMath Creation: Open source large language model specifically designed for mathematical reasoning.
- Evol-Instruct Method: Innovative technique for evolving instruction datasets, ensuring diversity and complexity.
- Reinforced Evol-Instruct: Novel reinforcement learning approach involving Instruction Reward Model (IRM) and Process-Supervised Reward Model (PRM).
- Results: WizardMath outperforms other open source models, showcasing significant improvement on GSM8k and MATH datasets.
- Room for Improvement: While competitive, WizardMath has potential for further enhancement, especially when compared to top closed source models.
References: