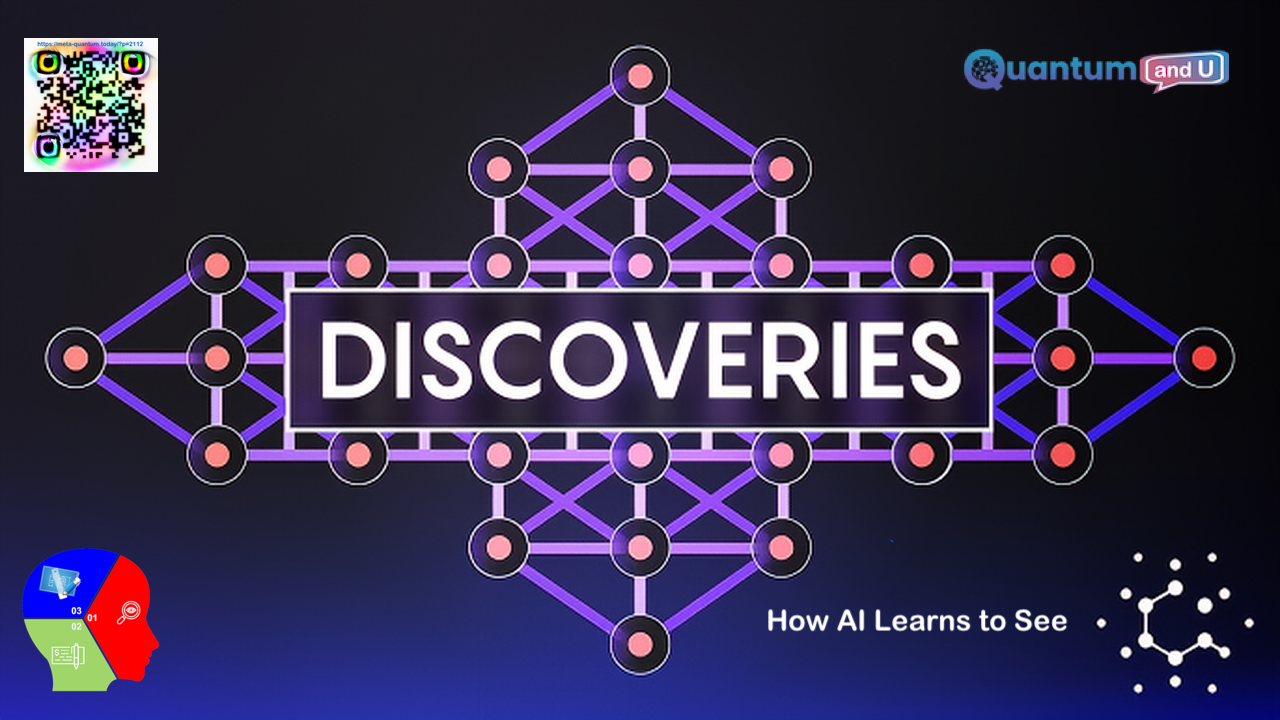
Introduction:
The video titled “How AI Learns to See” provides an in-depth analysis of the complex process of teaching computers to interpret and comprehend the visual world. It offers a comprehensive exploration of the evolution of computer vision, highlighting the various challenges encountered along the way. Moreover, the speaker, drawing from their personal experiences, offers a unique perspective that sheds light on the significance of visual data in this field. Additionally, the video delves into the historical context of AI in vision, painting a vivid picture of how far this technology has come. Lastly, it discusses the paradigm shift that has taken place in contemporary computer vision, unveiling the groundbreaking advancements that have revolutionized this domain.
How AI Learn to See:
Unlike humans who learn to see through a gradual process of interacting with the world around us, Computer Vision AI learns to see through a very different approach. It’s a fascinating process that involves massive amounts of data, complex algorithms, and a bit of human guidance.
Here’s a breakdown of the key steps:
1. Data Collection:
The first step is to gather a huge dataset of images and videos. This can include everything from everyday objects and scenes to medical scans and satellite imagery. The more diverse and comprehensive the data, the better the AI will be able to learn and generalize.
2. Labeling and Annotation:
Once the data is collected, it needs to be labeled and annotated. This means that humans have to go through each image or video and identify the objects, scenes, or actions that are present. This is a tedious and time-consuming process, but it’s essential for the AI to understand what it’s looking at.
3. Training the AI:
The labeled data is then fed into a special type of machine learning algorithm called a convolutional neural network (CNN). CNNs are designed to mimic the structure of the human visual cortex, which is the part of the brain that processes visual information.
As the AI is exposed to more and more data, the CNN learns to identify patterns and relationships in the images. It starts to recognize edges, shapes, textures, and colors, and it begins to associate these features with specific objects or concepts.
4. Testing and Refining:
Once the AI has been trained, it’s time to test its skills. The AI is given new images that it hasn’t seen before, and it’s asked to identify the objects or scenes that are present. If the AI makes mistakes, the training process is repeated with more data or adjustments to the algorithm.
This process of training, testing, and refining is repeated over and over again until the AI reaches a desired level of accuracy.
5. Real-World Applications:
Once the AI is trained, it can be used for a variety of real-world applications, such as:
- Facial recognition: AI can be used to identify people in photos and videos. This is used for security purposes, as well as for marketing and personalization.
- Self-driving cars: AI can be used to help self-driving cars navigate their surroundings and avoid obstacles.
- Medical diagnosis: AI can be used to analyze medical images, such as X-rays and MRIs, to help doctors diagnose diseases.
- Image search: AI can be used to power image search engines, such as Google Images.
The field of computer vision is still in its early stages, but it has already made significant progress. As AI technology continues to develop, we can expect to see even more amazing and innovative applications in the years to come.
I hope this gives you a better understanding of how AI learns to see. If you have any other questions, please feel free to ask!
How AI Learns to See’s Video:
Related Sections about this video:
- The Complexity of Human Vision:
- Describes the natural, complex process of human vision.
- Highlights the challenge of translating this process into a computational problem.
- Early Vision in AI:
- Discusses the initial perception that writing a smart algorithm would teach computers to see.
- Emphasizes the later realization of the need to connect sensory input with past experiences.
- The Role of Visual Memory:
- Shares the speaker’s personal advantage of compensating for poor vision with strong visual memory.
- Stresses the importance of large-scale visual data in understanding and modeling the world.
- Data’s Fundamental Role:
- Explores the significance of data in machine learning and computer vision.
- Highlights the diverse applications of visual data in real-world scenarios, including self-driving cars and computational photography.
- Two Paradigms in Computer Vision:
- Contrasts supervised learning with self-supervised learning.
- Discusses the biases introduced by linguistic supervision and the benefits of removing labels.
- Continuous Learning Challenge:
- Explores the challenge of continuous adaptation in AI compared to biological agents.
- Introduces the concept of test-time training to address the issue of generalization in changing environments.
- Test-Time Training:
- Describes the concept of adapting the model with each new piece of data.
- Provides an example in the context of self-driving cars adapting to different weather conditions.
- Advancements and Excitement:
- Expresses excitement about recent advancements, mentioning text-generative models like Chat GPT.
- Highlights the potential insights gained by exploring the connection between robotics and computer vision.
Market size for AI learns to See applications in SEA for next 5 years:
“How AI learns to see” in Southeast Asia over the next five years is challenging. This is due to several factors:
- Broad scope: The phrase “how computer vision AI learns to see” encompasses a wide range of technologies and applications, making it difficult to isolate a specific market segment.
- Limited data: While the Southeast Asian AI market is growing rapidly, specific data on applications related to AI’s learning process is scarce.
- Evolving landscape: The field of computer vision is constantly evolving, making it difficult to predict which specific applications will gain traction in the future.
However, we can explore some insights and potential trends:
Overall AI market in Southeast Asia:
- The Southeast Asian AI market is expected to reach USD 11.2 billion by 2025, growing at a CAGR of 25.8%.
- Key sectors driving growth include:
- Retail: AI-powered personalization and product recommendations.
- Finance: Fraud detection and risk management.
- Healthcare: Medical imaging analysis and disease diagnosis.
- Government initiatives: Several Southeast Asian governments are actively promoting AI adoption, creating a supportive environment for market growth.
Potential applications for “how computer vision AI learns to see”:
- Educational tools: Interactive platforms demonstrating the inner workings of AI vision algorithms could be used for educational purposes in universities and tech training programs.
- Explainable AI (XAI) tools: Visualizations and explanations of how AI models arrive at their conclusions could be valuable for building trust and understanding in AI systems.
- AI development platforms: Tools and resources that simplify the development and deployment of computer vision applications could be attractive to startups and enterprises in the region.
- Creative applications: AI-powered artistic tools and experiences that leverage the “learning to see” process could find a niche market in the region’s vibrant creative scene.
Market size estimation challenges:
- Quantifying the market size for these specific applications would require further research and analysis, taking into account factors like:
- Specific target audiences and use cases.
- Willingness to pay for such tools and resources.
- Competition from existing solutions in the market.
- Adoption rate and market penetration.
Overall, while the precise market size for applications related to “how AI learns to see” in Southeast Asia is difficult to pinpoint, the overall AI market in the region offers significant growth potential. As AI adoption continues to rise and XAI solutions become more important, the demand for tools and resources that demystify AI vision could create interesting opportunities in the coming years.
Conclusion:
The speaker concludes by expressing ongoing discoveries in the field and the excitement surrounding large datasets, particularly in the context of text-generative models. In addition to the advancements in this field, the speaker highlights the potential implications and applications of these discoveries. The dynamic relationship between data and algorithms is further explored, with the speaker drawing parallels to the field of neuroscience. The speaker emphasizes the idea of “doing neuroscience on the computer” as a way to understand the intricate workings of data and algorithms. This concept highlights the continuous evolution of technology and its impact on various fields of study.
Key Takeaway Points:
- Vision is a complex process for both humans and computers.
- The historical journey of AI in vision, from early optimism to the realization of the importance of connecting sensory input with past experiences.
- The crucial role of visual memory and large-scale visual data in training computers to see.
- The significance of data in machine learning and real-world applications.
- Contrasting supervised and self-supervised learning paradigms in computer vision.
- The challenge of continuous learning in AI and the concept of test-time training.
- Excitement about recent advancements, particularly in text-generative models like Chat GPT.
- The potential insights gained by exploring the intersection of robotics and computer vision.
References: