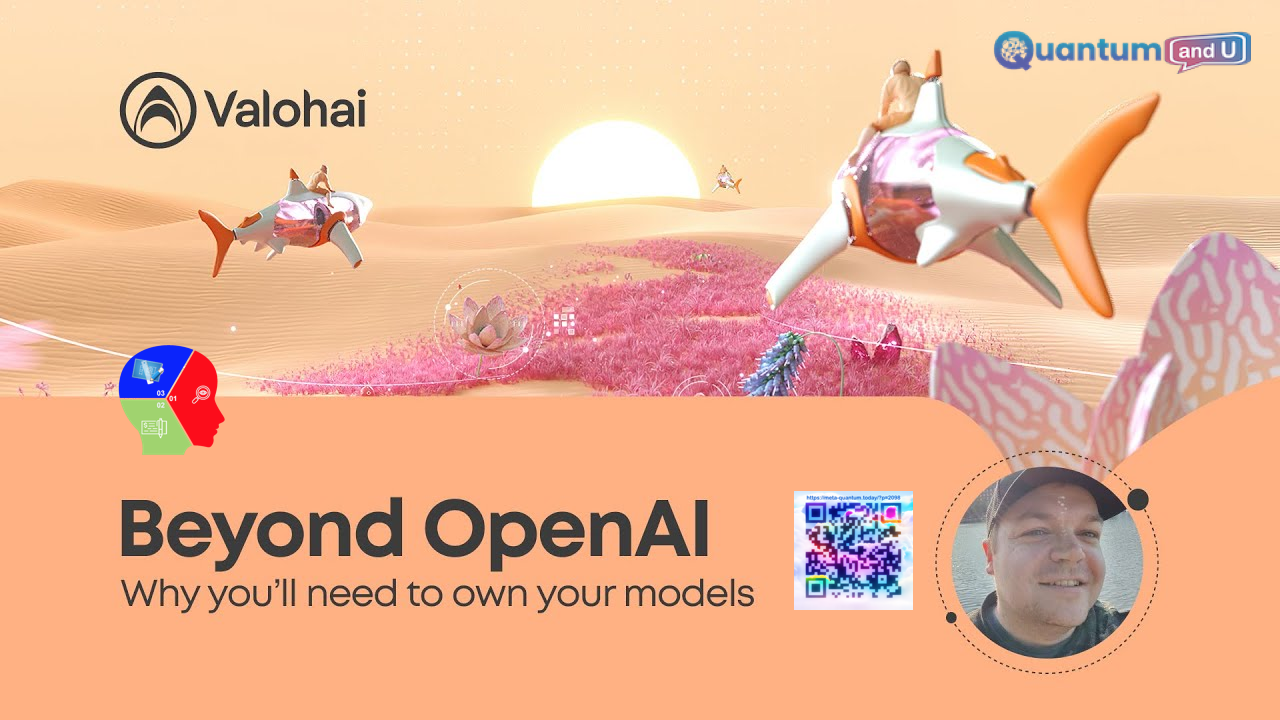
Introduction:
Henrik, who is the Head of Product at Wohi, delves into a comprehensive discussion on the evolution of machine learning models beyond the well-known language models (LLMs) developed by OpenAI. Throughout the review, Henrik highlights the increasing importance of owning your own models in the fast-paced and ever-evolving field of machine learning. He emphasizes the significance of this ownership particularly in light of the dynamic changes taking place within the machine learning landscape. By providing valuable insights and thought-provoking analysis, Henrik offers a fresh and unique perspective that will undoubtedly expand your understanding of the subject matter.
About Valohai AI:
Valohai AI is an end-to-end MLOps platform designed specifically for “ML Pioneers,” a term they use for companies heavily invested in and pushing the boundaries of machine learning. Valohai helps these pioneers manage the entire machine learning lifecycle, from training and deploying models to tracking their performance and collaborating on projects.
Here’s a quick breakdown of what Valohai does:
- Streamlines the ML workflow: Valohai brings together all the tools and processes needed for developing, deploying, and managing machine learning models into a single platform. This can save teams a lot of time and effort, as they no longer need to switch between different tools or manage complex infrastructure.
- Makes collaboration easy: Valohai makes it easy for data scientists, engineers, and other stakeholders to collaborate on machine learning projects. The platform provides features like version control, experiment tracking, and project management tools that help teams stay organized and on track.
- Automates tasks: Valohai can automate many of the tedious tasks involved in deploying and managing machine learning models. This frees up data scientists to focus on more important work, such as developing new models and improving existing ones.
- Runs on any cloud: Valohai is cloud-agnostic, which means it can be used with any major cloud provider. This gives organizations the flexibility to choose the cloud platform that best meets their needs.
Valohai vs. other MLOps platforms:
There are a number of other MLOps platforms on the market, such as MLflow, Kubeflow, and Amazon SageMaker. Valohai differentiates itself from these platforms in a few key ways:
- Focus on ML Pioneers: Valohai is specifically designed for companies that are heavily invested in machine learning and pushing the boundaries of what’s possible. This means that the platform is packed with features that these companies need, such as support for complex ML workflows and advanced experimentation capabilities.
- User-friendly interface: Valohai is known for its user-friendly interface, which makes it easy for data scientists and engineers of all levels to use. This is in contrast to some other MLOps platforms, which can be difficult to learn and use.
- Open source: Valohai’s core platform is open source, which means that it is free to use and can be customized to meet the specific needs of an organization. This is in contrast to some other MLOps platforms, which are closed source and can be expensive to use.
Ultimately, the best MLOps platform for your organization will depend on your specific needs and requirements. However, if you are a company that is heavily invested in machine learning and looking for a platform that can help you accelerate your ML initiatives, Valohai is definitely worth considering.
More about Valohai AI in this Video:
Related Sections About the Video:
- Evolution of ML Use Cases: Henrik starts by highlighting the transition from single-use machine learning cases to the widespread adoption of machine learning in various applications, especially with the emergence of LLMs.
- Impact of OpenAI’s Chat Interface: The pivotal moment, according to Henrik, was when OpenAI introduced the chat interface for LLMs. This led to a surge in interest from diverse stakeholders, including engineers, product managers, designers, ops personnel, and even leadership, resulting in an abundance of use cases.
- Current State of LLM Applications: Henrik observes an explosion of LLM applications, citing examples like a Finnish bread maker’s sandwich bot. He acknowledges the mix of useful and less useful applications in this initial phase.
- Stages of LLM Applications: Henrik describes the current stage as focusing on retrieval-augmented generation (RAG) and complex systems built on top of the OpenAI API. He emphasizes the importance of testing and iterating quickly using the API.
- Challenges in Stage Two: Control over Data, Cost, Performance, and Service: Henrik identifies four critical aspects – control over data, cost, performance, and service – as challenges in the second stage of LLM application development. He elaborates on each, emphasizing the need for increased control.
- Control Over Data and Cost: Henrik discusses concerns about sending data outside one’s environment, especially in sensitive industries like healthcare or finance. He also delves into the challenge of calculating and managing costs associated with LLM scaling.
- Control Over Performance: Building on top of APIs is not new, but Henrik highlights the unique challenges in LLMs where changes in underlying logic can significantly impact performance unpredictably.
- Control Over Service: Henrik discusses the inherent risks associated with building on third-party services, including the potential for services to be shut down or changed, posing an existential risk to businesses.
Market size of MLOps in SEA:
- The MLOps market in Southeast Asia is expected to grow rapidly in the coming years. According to a report by ResearchAndMarkets, the market is expected to reach $1.2 billion by 2025, growing at a CAGR of 32.5%.
- The adoption of MLOps platforms is still in its early stages in the region, but there is a growing awareness of the benefits of these platforms, such as improved model performance, faster time to market, and reduced costs.
- Some of the key players in the MLOps market in Southeast Asia include Valohai AI, MLflow, Kubeflow, Amazon SageMaker, Microsoft Azure Machine Learning, and Google Cloud AI Platform. These platforms offer a variety of features and capabilities that can help organizations of all sizes to deploy and manage their machine learning models.
The overall growth of the MLOps market in Southeast Asia suggests that there is potential for the company to do well in the region. Valohai’s focus on “ML Pioneers” could also be a good fit for the Southeast Asian market, as there are a number of companies in the region that are heavily invested in machine learning.
Here are some additional things to keep in mind:
- The MLOps market in Southeast Asia is still fragmented, with a number of different vendors offering a variety of products and services. This can make it difficult for organizations to choose the right platform for their needs.
- The level of adoption of MLOps platforms varies from country to country in Southeast Asia. For example, Singapore is a more mature market than some of the other countries in the region.
- The cost of MLOps platforms can vary depending on the features and capabilities that are offered.
Conclusion:
In conclusion, Henrik suggests that certain use cases, such as internal productivity tools, may overlook these control issues. However, for core products that heavily rely on LLMs, ownership and control become crucial factors to consider. Henrik advocates for exploring open-source LLMs as a potential solution to address these challenges. By adopting open-source LLMs, companies can have greater transparency and flexibility in managing and controlling their core products.
This approach allows them to have a more active role in shaping the direction and functionality of their LLMs. Additionally, open-source LLMs provide opportunities for collaboration and innovation within the development community. This collaborative environment fosters continuous improvement and the ability to adapt to changing needs and requirements. Ultimately, embracing open-source LLMs can lead to more robust and sustainable solutions for companies relying on these technologies.
Key Takeaway Points:
- Diverse Stakeholder Involvement: LLMs have engaged a wide range of stakeholders beyond data scientists, including product managers, engineers, designers, ops personnel, and leadership.
- Explosion of Use Cases: The introduction of LLMs, particularly through the chat interface, has led to a surge in creative use cases and applications.
- Challenges in Stage Two: Control over data, cost, performance, and service emerge as critical challenges in the second stage of LLM application development.
- Need for Ownership: Henrik advocates for ownership and control over LLMs, especially for enterprises and core applications, suggesting exploration of open-source models.
Related References: