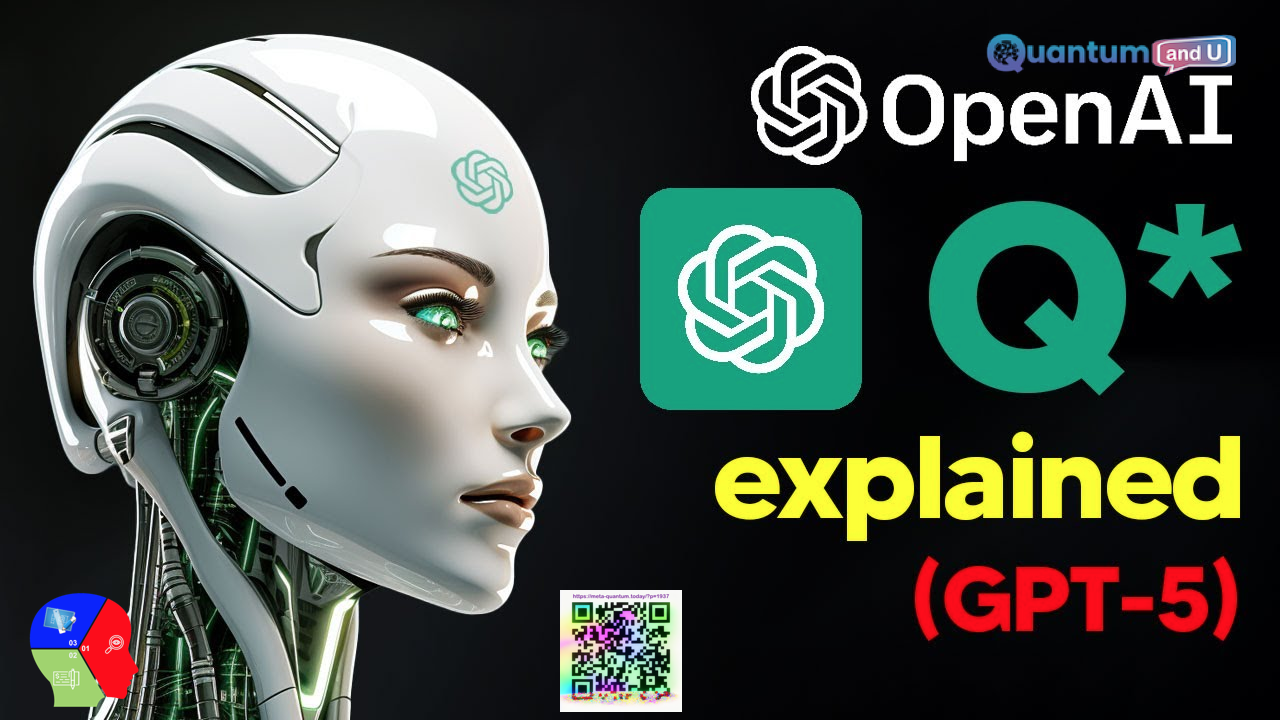
Introduction:
The YouTube review provides a comprehensive analysis of OpenAI’s latest groundbreaking innovation, Q* (Qstar). The review delves deep into the core concepts of Q learning, shedding light on its potential ramifications for large language models and AI systems, with a specific focus on GPT-5. By breaking down the complexities of Q learning, the video aims to make it accessible even to those who are new to the field of AI. Furthermore, the review explores the profound impact that Q learning could have on the future development and advancement of AI, positioning it as a pivotal milestone in the evolution of artificial intelligence.
OpenAI’s Q* – Qstar:
OpenAI’s Q*, also known as Qstar, is a rumored project that is said to be a significant breakthrough in the field of artificial intelligence. While there is no official information available about the project, it is believed to be a new approach to artificial general intelligence (AGI) that combines quantum computing and neuroscience.
Some experts believe that Q* could be a major leap forward in the development of AGI, as it could potentially overcome some of the limitations of current AI models. For example, current AI models are often very good at solving specific tasks, but they struggle to generalize their knowledge to new situations. Q*, on the other hand, is said to be able to learn and reason in a more human-like way, which could make it more versatile and adaptable.
However, there are also concerns about the potential risks of Q*. Some experts worry that Q* could be so powerful that it could become uncontrollable, or even pose a threat to humanity. Others are concerned that the development of Q* could lead to a widening gap between the rich and the poor, as only those who can afford access to the technology will be able to reap its benefits.
Despite the concerns, there is no doubt that Q* is a potentially revolutionary project that could have a profound impact on the future of AI. It is important to continue researching and developing Q* responsibly, so that we can harness its potential while also mitigating its risks.
Here are some of the key features of Q* that are said to be responsible for its potential breakthrough:
- Quantum computing: Q* is said to use quantum computing techniques, which could allow it to process information and solve problems in ways that are impossible for classical computers.
- Neuroscience: Q* is also said to be inspired by neuroscience, which could give it a more human-like understanding of the world.
- Learning and reasoning: Q* is said to be able to learn and reason in a more human-like way, which could make it more versatile and adaptable than current AI models.
It is important to note that all of this information is based on speculation and rumors. There is no official information available about Q*, and OpenAI has not commented on the project. However, the potential benefits of Q* are so significant that it is worth paying attention to any developments, even if they are unconfirmed.
OpenAI’s Q* Future Market Size:
OpenAI’s Q*, also known as Qstar, is a rumored project that is said to be a significant breakthrough in the field of artificial intelligence. While there is no official information available about the project, it is believed to be a new approach to artificial general intelligence (AGI) that combines quantum computing and neuroscience.
Given the potential of Q* to revolutionize various industries and aspects of our lives, it is difficult to overestimate its potential market size. The applications of AGI are vast and could span across various sectors, including healthcare, finance, manufacturing, transportation, and education.
Here’s a breakdown of the potential market size for Q* across different industries:
Healthcare: Q* could be used to develop personalized medicine, diagnose diseases early, and even design new drugs and therapies. The global healthcare market is expected to reach $11.9 trillion by 2028, indicating a massive potential for Q* in this sector.
Finance: Q* could be used to develop more sophisticated financial models, detect fraud, and optimize investment strategies. The global financial services market is estimated to be worth over $20 trillion, making it another lucrative market for Q*.
Manufacturing: Q* could be used to optimize supply chains, improve manufacturing processes, and develop new products. The global manufacturing industry is valued at over $13 trillion, highlighting the potential market for Q* in this sector.
Transportation: Q* could be used to develop self-driving cars, optimize traffic flow, and design new transportation systems. The global transportation industry is worth over $5 trillion, indicating a significant market opportunity for Q*.
Education: Q* could be used to personalize education, provide real-time feedback, and automate grading tasks. The global education market is expected to reach $10 trillion by 2030, making it another potential market for Q*.
These are just a few examples of the potential applications of Q*, and the market size could be even larger if Q* proves to be as revolutionary as it is rumored to be. It is important to note that these are just estimates, and the actual market size for Q* will depend on its capabilities and the rate at which it is adopted.
Overall, the potential market size for Q* is vast and could span across various industries. The applications of AGI are limitless, and Q* has the potential to transform our world in ways we can only begin to imagine.
Understanding Q* from this video:
Related Sections about this Video:
- Origin of Q (Qstar) Name:
The video begins by breaking down the name Q (Qstar), suggesting that the “Q” likely refers to Q learning, a type of machine learning used in reinforcement learning. The “star” part comes from the A search algorithm, a pathfinding and graph traversal algorithm widely used in computer science. - Simplified Explanation of Q Learning: The video provides a simplified definition of Q learning, likening it to training a super-smart robot. It emphasizes that Q learning involves rewarding the AI for good decisions and penalizing it for bad decisions, drawing parallels to training a pet.
- Six Steps to Understanding Q Learning: The video breaks down Q learning into six key steps:
- Step 1: Environment and Agent
- Step 2: States and Actions
- Step 3: Q Table
- Step 4: Learning by Doing
- Step 5: Update the Q Table
- Step 6: Overtime Improvement
- Comparison with Large Language Models (LLMs): The video highlights the limitations of LLMs, such as data dependency, static knowledge, challenges in context understanding, and issues of bias and fairness. It introduces Q learning as a potential solution to overcome these limitations.
- Q (Qstar) as a Future Option: *The video features a clip from a Google DeepMind expert discussing the limitations of current LLMs and the need for systems that can go beyond their training data. It suggests that Q (Qstar) is being explored as a viable option for the future of large language models.
- Limitations of Large Language Models: The video discusses the drawbacks of LLMs, emphasizing their reliance on massive amounts of data, static knowledge, challenges in context understanding, and the presence of biases.
- Optimization of Decisions with Q Learning: The video highlights how Q learning focuses on finding the best decisions to achieve a goal, leading to more effective and efficient decision-making processes in various applications.
Conclusion:
The video concludes by proposing Q learning as a next-level advancement in AI, particularly for large language models. It underlines the potential of Q* (Qstar) to address the limitations of current LLMs and speculates on its impact compared to GPT-4. The audience is left with the anticipation of how this breakthrough could shape the future of AI.
Takeaway Key Points:
- Q* (Qstar) combines Q learning and A* search algorithm for potential breakthroughs in AI.
- Q learning involves environment, agent, states, actions, Q table, learning by doing, and continuous improvement.
- Large language models have limitations, and Q* (Qstar) is explored as a solution.
- Q learning offers more effective decision-making processes with long-term consequences considered.
- Q* (Qstar) could represent a significant leap in AI capabilities, potentially surpassing current models.
Related References: