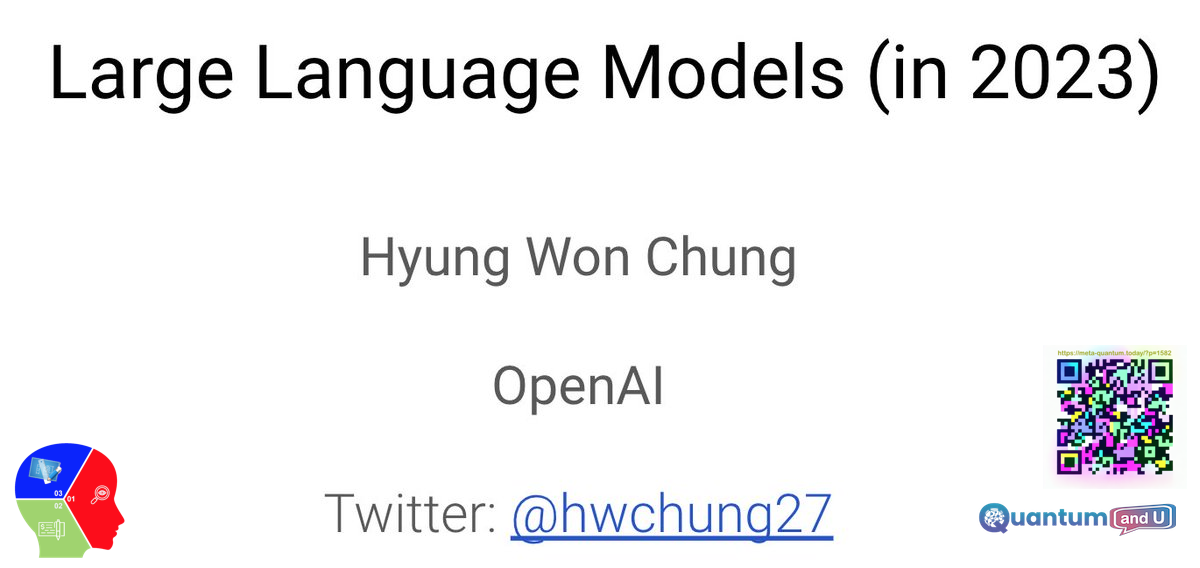
In this YouTube review titled “Large Language Models (in 2023),” the presenter delves into the evolving landscape of large language models, challenging the perception of scale and discussing the unique aspects of these models. They explore fundamental ideas derived from observing the field for several years and interacting with leading experts.
Large Language Models (in 2023):
Large language models (LLMs) have made significant progress in recent years, achieving state-of-the-art performance on a wide range of natural language processing tasks. LLMs are trained on massive datasets of text and code, and can be used to generate text, translate languages, write different kinds of creative content, and answer your questions in an informative way.
What are LLMs and how do they work?
LLMs are a type of machine learning model that is trained to predict the next word in a sequence. They are typically trained on a massive dataset of text and code, and learn to identify patterns and relationships between words. Once trained, LLMs can be used to generate text, translate languages, write different kinds of creative content, and answer your questions in an informative way.
LLMs in 2023
In 2023, LLMs are more powerful and versatile than ever before. They are being used to develop a wide range of new applications, including:
- Code generation: LLMs can be used to generate code in a variety of programming languages, which can save developers time and improve productivity.
- Creative writing: LLMs can be used to write different kinds of creative content, such as poems, stories, and scripts.
- Customer service: LLMs can be used to create chatbots that can answer customer questions and provide support.
- Education: LLMs can be used to develop personalized learning experiences and provide students with feedback on their work.
- Research: LLMs are being used to accelerate scientific research by helping scientists to generate hypotheses, analyze data, and write reports.
Challenges and limitations
While LLMs have made significant progress, there are still some challenges and limitations that need to be addressed. For example, LLMs can be biased and generate inaccurate or harmful content. Additionally, LLMs can be expensive to train and deploy.
Related Sections:
- Scale and Emergence: The presenter extensively discusses the fascinating and remarkable phenomenon where certain remarkable and extraordinary abilities in large language models emerge only at specific, well-defined scales. They strongly emphasize and underscore the utmost importance and significance of viewing and perceiving failures and setbacks as temporary and transitory, thereby indicating that what might not effectively and efficiently work or produce desired outcomes at this present moment or juncture could potentially and quite possibly work and yield fruitful results in the future as these models continue to evolve, advance, and progress.
- Scaling Perspectives: The video provides a comprehensive overview of various aspects related to scaling, tokenization, word embedding, and the computational challenges encountered when dealing with large language models. In addition to that, it delves into the intricacies of data and model parallelism, mesh tensorflow, and the obstacles that arise during model training and debugging. By exploring these concepts in depth, the video aims to provide a thorough understanding of the subject matter and equip viewers with the knowledge they need to navigate these complex topics effectively.
- Learning Objectives and Reward Models: The presenter raises doubts about the effectiveness of maximum likelihood as the sole learning objective for large models. In order to address this concern, they introduce the concept of reward models, which involve incorporating human preferences into the model’s learning process. This approach leads to intriguing discussions on the potential pitfalls of reward hacking and also paves the way for further exploration of open-ended research questions.
- Paradigm Shifts in AI: The review provides a comprehensive analysis of the evolution of AI paradigms. It starts by exploring classical machine learning systems and their impact on the field. It then delves into the revolutionary change brought about by the introduction of large language models like GPT-3. These models have not only transformed the way we approach AI, but have also opened up new possibilities and avenues for research and development. This review carefully examines the implications and potential of such models, shedding light on their significance in shaping the future of AI.
Conclusion and Takeaway Key Points:
The presenter concludes by emphasizing the crucial importance of thoroughly comprehending the perpetually evolving nature of large language models. They strongly underscore the imperative necessity for maintaining a fluid and adaptable mindset, fully acknowledging that strategies that may not yield desirable results presently have the potential to be successful in the future as the models progress and improve. Furthermore, the review elucidates and accentuates the profound significance of scaling the models, the emergence of new possibilities, and the ongoing process of adaptation in the dynamic and ever-evolving field of large language models.
Takeaway Key Points:
- Perspective Shift: Researchers must view failures as temporary (“doesn’t work yet”) due to evolving models.
- Understanding Scale: Understanding problems in terms of scale and parallelism is crucial for large-scale models.
- Reward Models: Exploring reward models and policy networks presents potential avenues but comes with challenges like reward hacking.
Future directions:
Hyung Won Chung also discussed some of the future directions for LLMs in his talk. He suggested that LLMs will become more efficient and accessible in the future, and that they will be used to develop even more innovative applications. He also emphasized the importance of developing responsible and ethical guidelines for the use of LLMs.
References: